The Importance of Image Interpretation: Patterns of Semantic Misclassification in Real-World Adversarial Images
arxiv(2022)
Abstract
Adversarial images are created with the intention of causing an image classifier to produce a misclassification. In this paper, we propose that adversarial images should be evaluated based on semantic mismatch, rather than label mismatch, as used in current work. In other words, we propose that an image of a "mug" would be considered adversarial if classified as "turnip", but not as "cup", as current systems would assume. Our novel idea of taking semantic misclassification into account in the evaluation of adversarial images offers two benefits. First, it is a more realistic conceptualization of what makes an image adversarial, which is important in order to fully understand the implications of adversarial images for security and privacy. Second, it makes it possible to evaluate the transferability of adversarial images to a real-world classifier, without requiring the classifier's label set to have been available during the creation of the images. The paper carries out an evaluation of a transfer attack on a real-world image classifier that is made possible by our semantic misclassification approach. The attack reveals patterns in the semantics of adversarial misclassifications that could not be investigated using conventional label mismatch.
MoreTranslated text
Key words
misclassification,image interpretation,images,real-world real-world
AI Read Science
Must-Reading Tree
Example
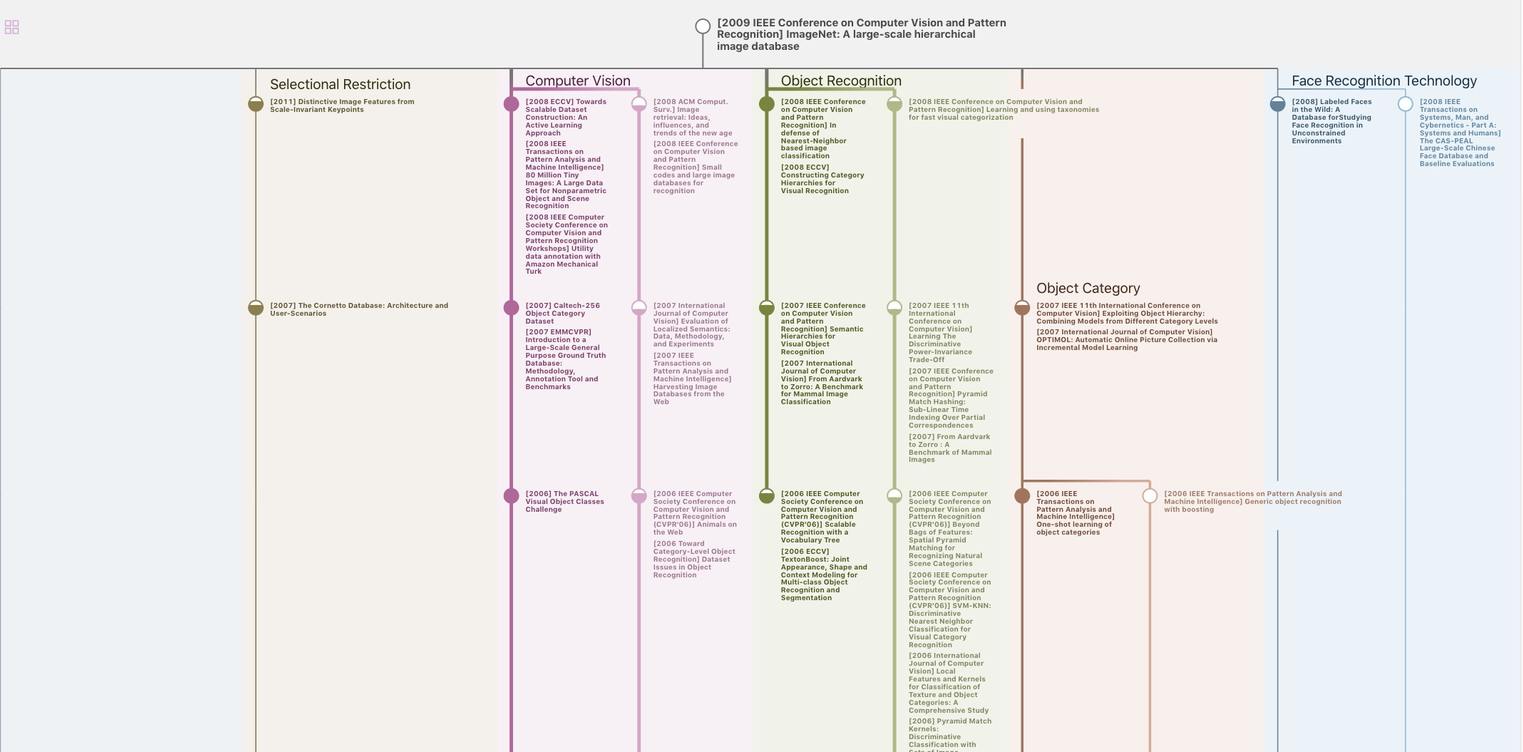
Generate MRT to find the research sequence of this paper
Chat Paper
Summary is being generated by the instructions you defined