The substrate scopes of enzymes: a general prediction model based on machine and deep learning
biorxiv(2022)
摘要
For a comprehensive understanding of metabolism, it is necessary to know all potential substrates for each enzyme encoded in an organism's genome. However, for most proteins annotated as enzymes, it is unknown which primary and/or secondary reactions they catalyze, as experimental characterizations are time-consuming and costly. Machine learning predictions could provide an efficient alternative, but are hampered by a lack of information regarding enzyme non-substrates, as available training data comprises mainly positive examples. Here, we present ESP, a general machine learning model for the prediction of enzyme-substrate pairs, with an accuracy of over 90% on independent and diverse test data. This accuracy was achieved by representing enzymes through a modified transformer model with a trained, task-specific token, and by augmenting the positive training data by randomly sampling small molecules and assigning them as non-substrates. ESP can be applied successfully across widely different enzymes and a broad range of metabolites. It outperforms recently published models designed for individual, well-studied enzyme families, which use much more detailed input data. We implemented a user-friendly web server to predict the substrate scope of arbitrary enzymes, which may support not only basic science, but also the development of pharmaceuticals and bioengineering processes.
### Competing Interest Statement
The authors have declared no competing interest.
更多查看译文
关键词
enzymes,substrate scopes,general prediction model
AI 理解论文
溯源树
样例
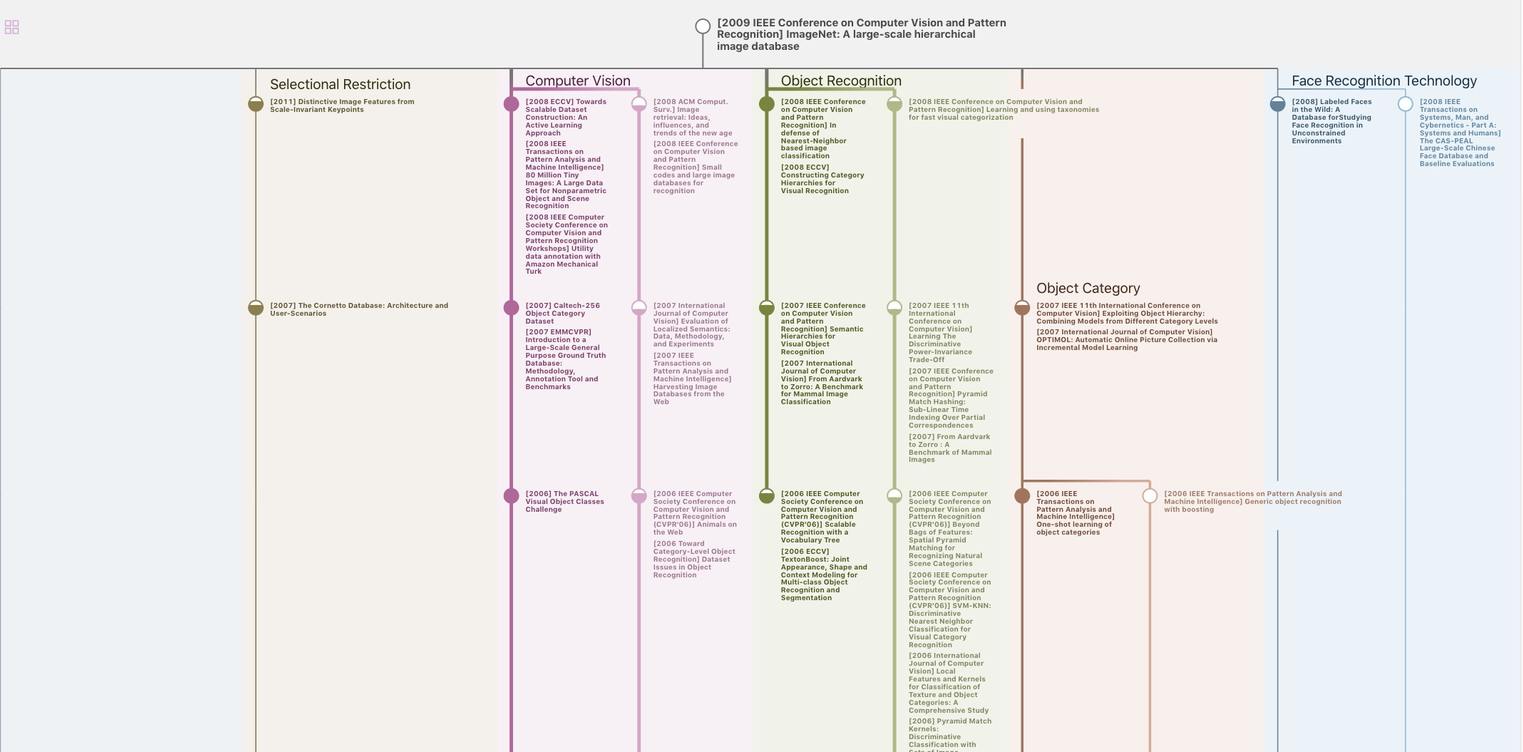
生成溯源树,研究论文发展脉络
Chat Paper
正在生成论文摘要