Predicting coordinates of peptide features in raw timsTOF data with machine learning for targeted extraction reduces missing values in label-free DDA LC-MS/MS proteomics experiments
biorxiv(2022)
摘要
The determination of relative protein abundance in label-free data dependant acquisition (DDA) LC-MS/MS proteomics experiments is hindered by the stochastic nature of peptide detection and identification. Peptides with an abundance near the limit of detection are particularly effected. The possible causes of missing values are numerous, including; sample preparation, variation in sample composition and the corresponding matrix effects, instrument and analysis software settings, instrument and LC variability, and the tolerances used for database searching.
There have been many approaches proposed to computationally address the missing values problem, predominantly based on transferring identifications from one run to another by data realignment, as in MaxQuant’s matching between runs (MBR) method, and/or statistical imputation. Imputation transfers identifications by statistical estimation of the likelihood the peptide is present based on its presence in other technical replicates but without probing the raw data for evidence.
Here we present a targeted extraction approach to resolving missing values without modifying or realigning the raw data. Our method, which forms part of an end-to-end timsTOF processing pipeline we developed called Targeted Feature Detection and Extraction (TFD/E), predicts the coordinates of peptides using machine learning models that learn the delta of each peptide’s coordinates from a reference library. The models learn the variability of a peptide’s location in 3D space from the variability of known peptide locations around it. Rather than realigning or altering the raw data, we create a run-specific ‘lens’ through which to observe the data, targeting a location for each peptide of interest and extracting it. By also creating a method for extracting decoys, we can estimate the false discovery rate (FDR). Our method outperforms MaxQuant and MSFragger by achieving substantially fewer missing values across an experiment of technical replicates. The software has been developed in Python using Numpy and Pandas and open sourced with an MIT license (DOI 10.5281/zenodo.6513126) to provide the opportunity for further improvement and experimentation by the community. Data are available via ProteomeXchange with identifier PXD030706.
Author Summary Missed identifications of peptides in data-dependent acquisition (DDA) proteomics experiments are an obstacle to the precise determination of which proteins are present in a sample and their relative abundance. Efforts to address the problem in popular analysis workflows include realigning the raw data to transfer a peptide identification from one run to another. Another approach is statistically analysing peptide identifications across an experiment to impute peptide identifications in runs in which they were missing.
We propose a targeted extraction technique that uses machine learning models to construct a run-specific lens through which to examine the raw data and predict the coordinates of a peptide in a run. The models are trained on differences between observations of confidently identified peptides in a run and a reference library of peptide observations collated from multiple experiments. To minimise the risk of drawing unsound experimental conclusions based on an unknown rate of false discoveries, our method provides a mechanism for estimating the false discovery rate (FDR) based on the misclassification of decoys as target features. Our approach outperforms the popular analysis tool suites MaxQuant and MSFragger/IonQuant, and we believe it will be a valuable contribution to the proteomics toolbox for protein quantification.
### Competing Interest Statement
The authors have declared no competing interest.
更多查看译文
关键词
peptide features,raw timstof data,label-free
AI 理解论文
溯源树
样例
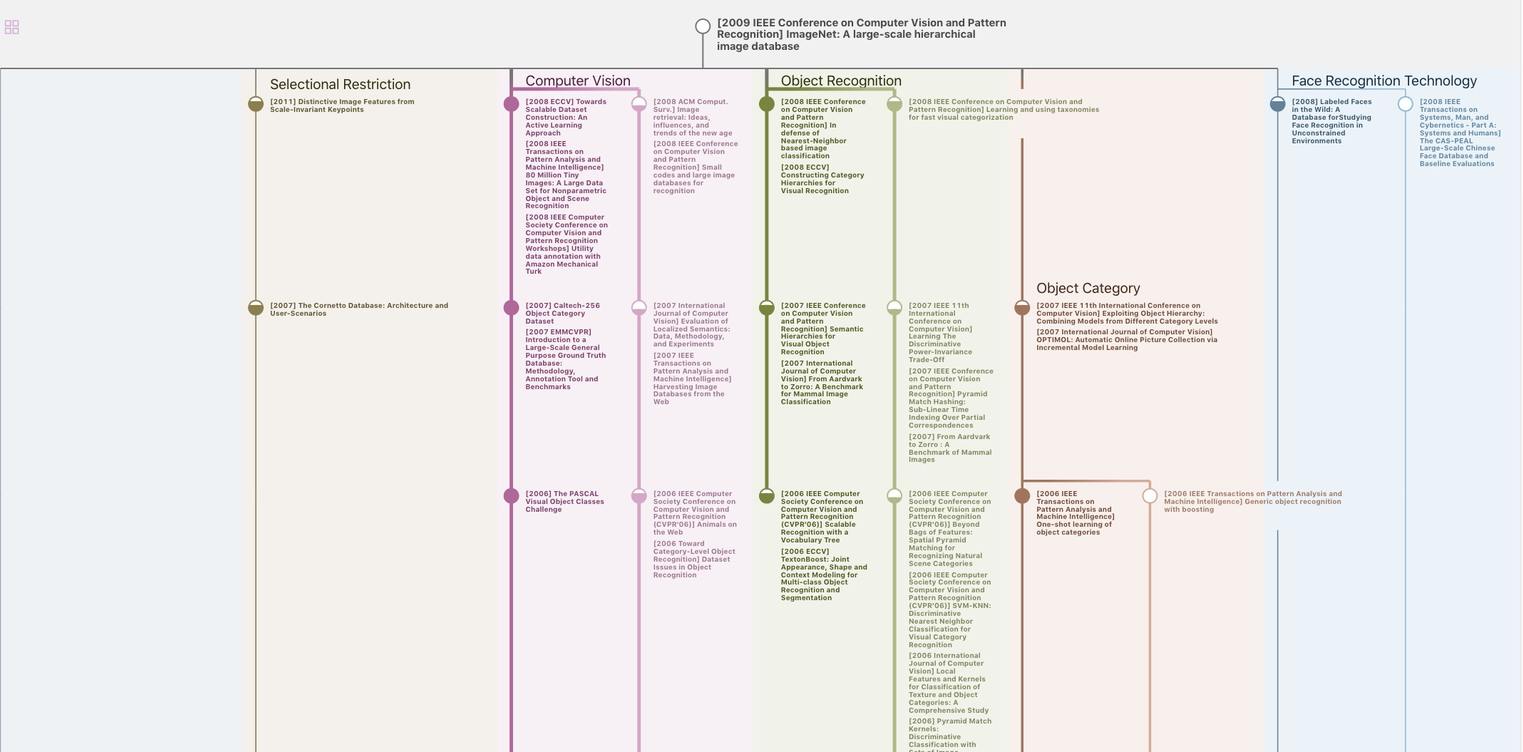
生成溯源树,研究论文发展脉络
Chat Paper
正在生成论文摘要