Interpolating Strange Attractors via Fractional Brownian Bridges
ENTROPY(2022)
摘要
We present a novel method for interpolating univariate time series data. The proposed method combines multi-point fractional Brownian bridges, a genetic algorithm, and Takens' theorem for reconstructing a phase space from univariate time series data. The basic idea is to first generate a population of different stochastically-interpolated time series data, and secondly, to use a genetic algorithm to find the pieces in the population which generate the smoothest reconstructed phase space trajectory. A smooth trajectory curve is hereby found to have a low variance of second derivatives along the curve. For simplicity, we refer to the developed method as PhaSpaSto-interpolation, which is an abbreviation for phase-space-trajectory-smoothing stochastic interpolation. The proposed approach is tested and validated with a univariate time series of the Lorenz system, five non-model data sets and compared to a cubic spline interpolation and a linear interpolation. We find that the criterion for smoothness guarantees low errors on known model and non-model data. Finally, we interpolate the discussed non-model data sets, and show the corresponding improved phase space portraits. The proposed method is useful for interpolating low-sampled time series data sets for, e.g., machine learning, regression analysis, or time series prediction approaches. Further, the results suggest that the variance of second derivatives along a given phase space trajectory is a valuable tool for phase space analysis of non-model time series data, and we expect it to be useful for future research.
更多查看译文
关键词
time series interpolation, phase space reconstruction, Takens' theorem, interpolation, stochastic interpolation, genetic algorithm, time series data, preprocessing, strange attractor, attractor, attractor reconstruction
AI 理解论文
溯源树
样例
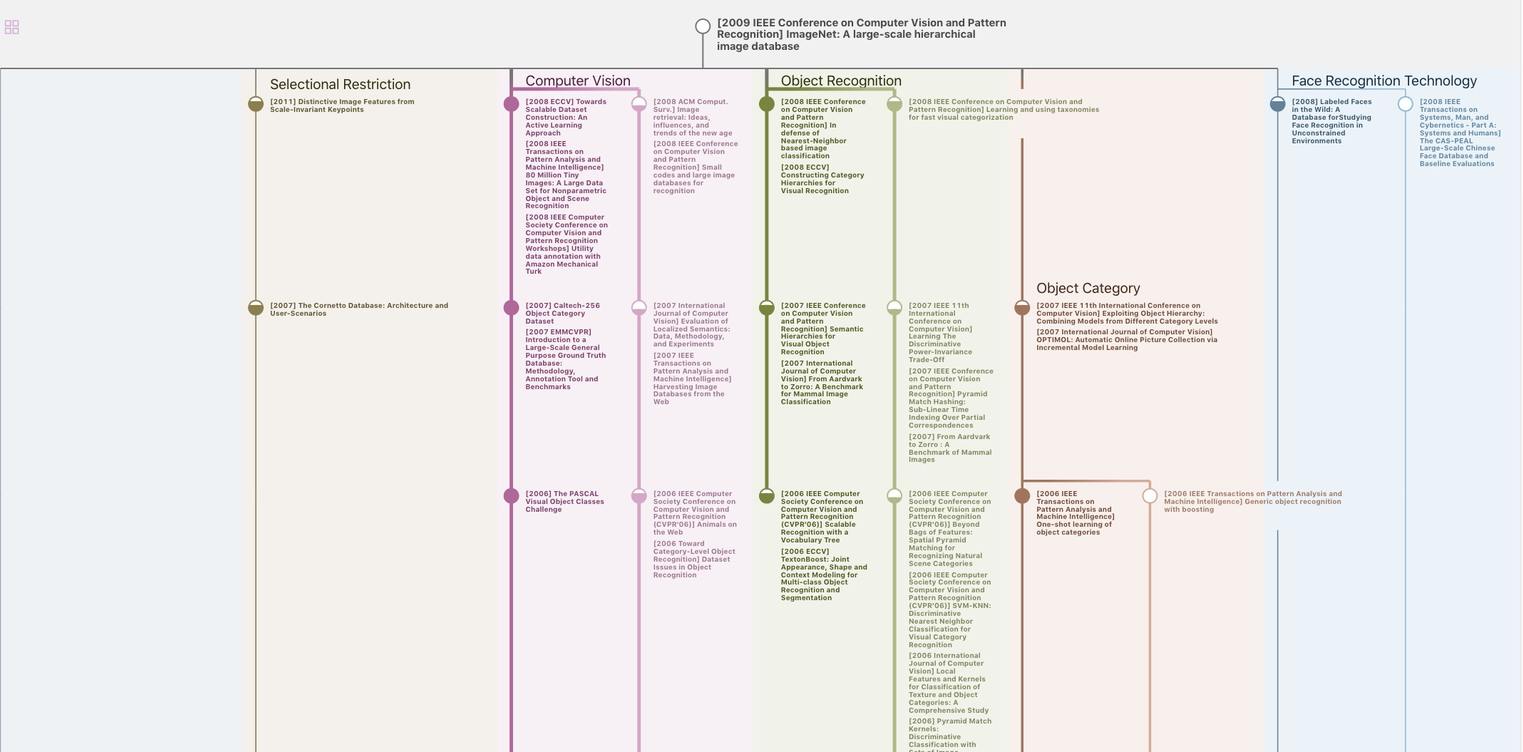
生成溯源树,研究论文发展脉络
Chat Paper
正在生成论文摘要