Monaural Speech Separation Method Based on Deep Learning Feature Fusion and Joint Constraints
Journal of Electronics & Information Technology(2022)
摘要
To improve the performance of monaural speech separation, a monaural speech separation method based on deep learning feature fusion and joint constraints is proposed. The loss function of the traditional separation algorithm based on deep learning only considers the error between the predicted value and the true one, which makes the error between the separated speech and the pure speech larger. To combat it, a new joint constrained loss function is proposed, which not only constrains the error between the predicted value and the true one of ideal ratio mask, but also penalizes the error of the corresponding amplitude spectrum. In addition, to make full use of the complementarity of multiple features, a Convolutional Neural Network (CNN) structure with feature fusion layer is proposed, which extract the depth feature of the multi-channel input feature, and then fuse the depth feature and the acoustic feature in the fusion layer to train the separation model. The fused separation feature contains abundant acoustic information and has a strong acoustic representative ability, which makes the mask predicted by the separation model more accurate. The experimental results show that from Signal Distortion Ratio (SDR), Perceptual Evaluation of Speech Quality (PESQ) and Short-Time Objective Intelligibility (STOI), compared with other excellent speech separation methods based on deep learning, the proposed method can separate the mixed speech more effectively.
更多查看译文
AI 理解论文
溯源树
样例
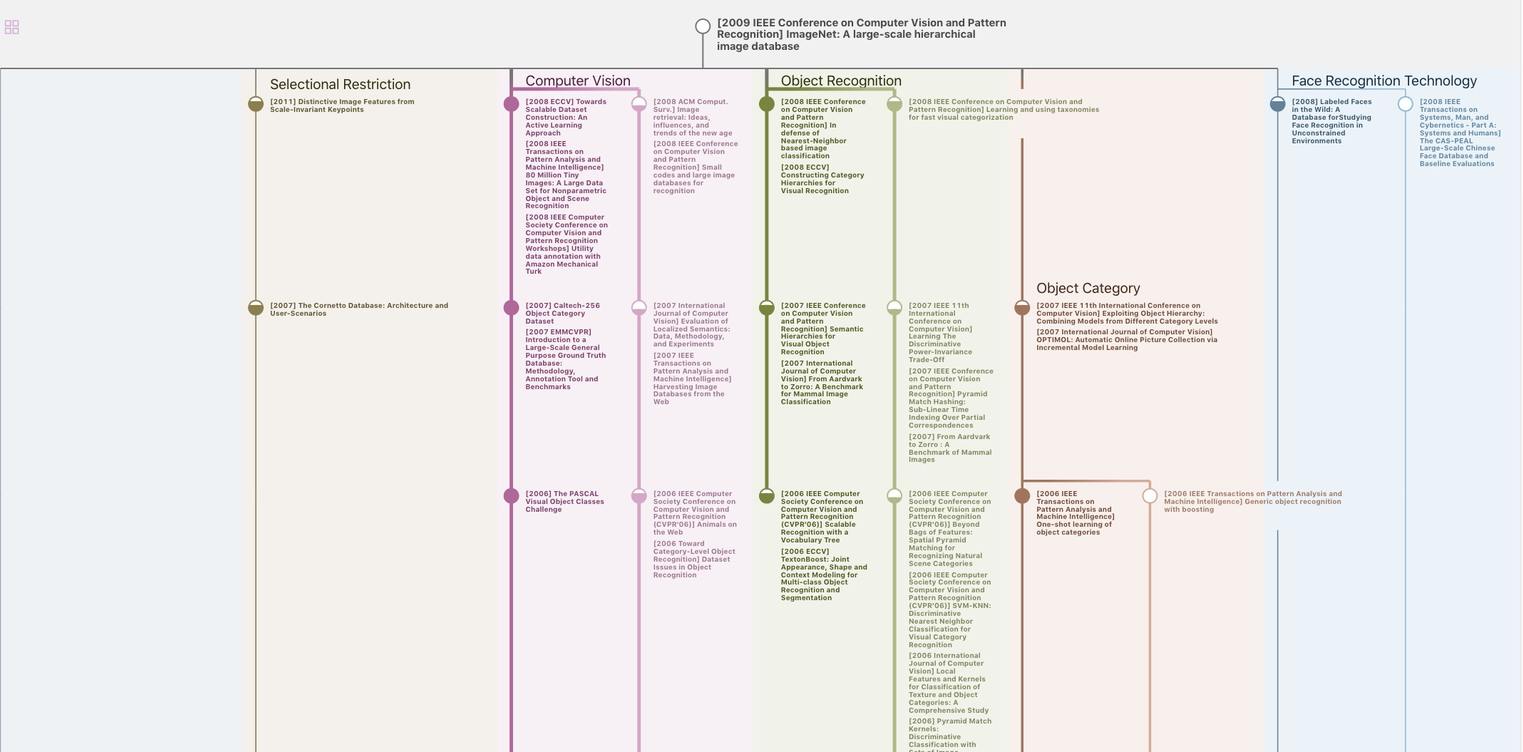
生成溯源树,研究论文发展脉络
Chat Paper
正在生成论文摘要