Multi-fidelity power flow solver
2022 Resilience Week (RWS)(2022)
摘要
We propose a multi-fidelity neural network (MFNN) tailored for rapid high-dimensional grid power flow simulations and contingency analysis with scarce high-fidelity contingency data. The proposed model comprises two networks—the first one trained on DC approximation as low-fidelity data and coupled to a high-fidelity neural network trained on both low- and high-fidelity power flow data. Each network features a latent module which parametrizes the model by a discrete grid topology vector for generalization (e.g., n power lines with k disconnections or contingencies, if any), and the targeted high-fidelity output is a weighted sum of linear and nonlinear functions. We tested the model on 14- and 118-bus test cases and evaluated its performance based on the n – k power flow prediction accuracy with respect to imbalanced contingency data and high-to-low-fidelity sample ratio. The results presented herein demonstrate MFNN's potential and its limits with up to two orders of magnitude faster and more accurate power flow solutions than DC approximation.
更多查看译文
关键词
contingency analysis,grid,machine learning,multi-fidelity modeling,power flow,resilience
AI 理解论文
溯源树
样例
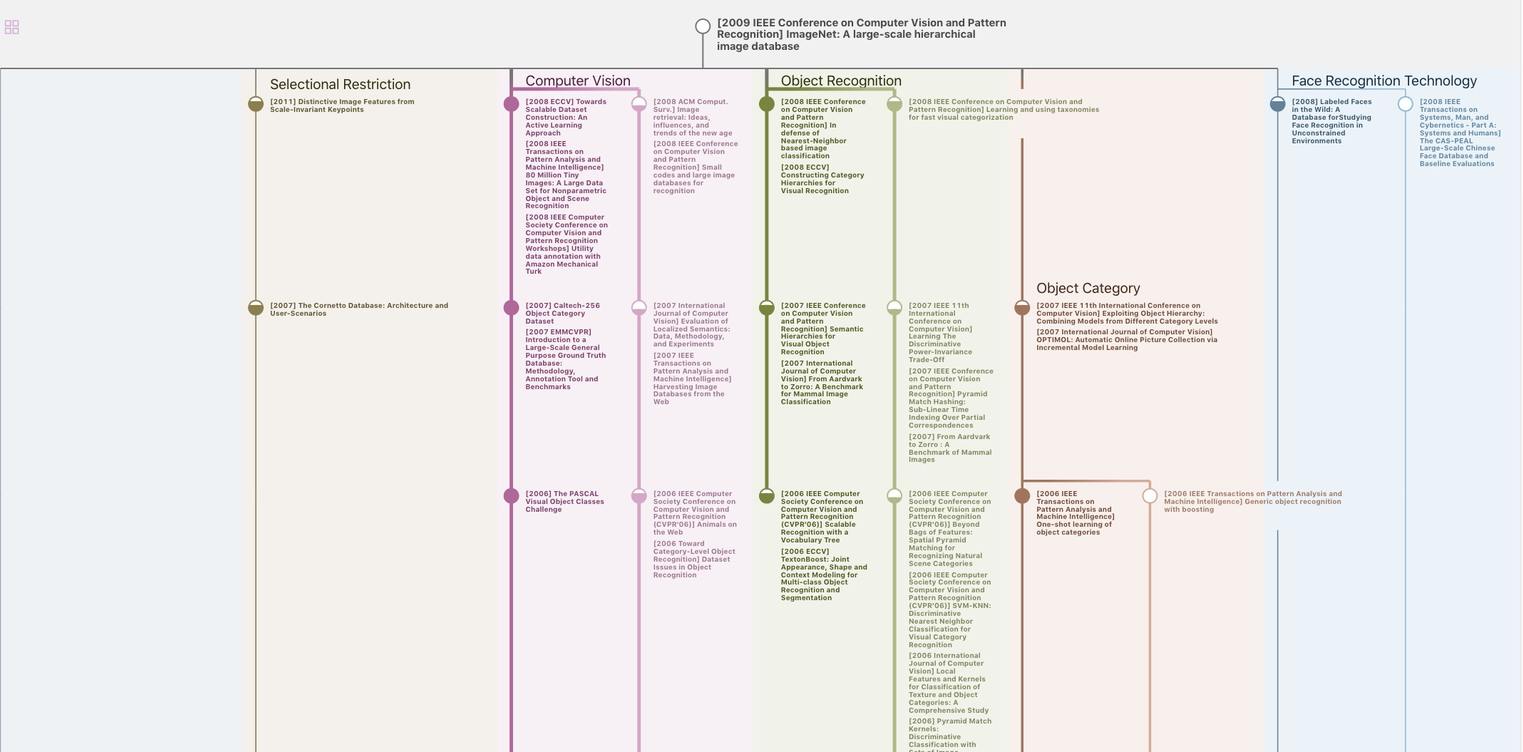
生成溯源树,研究论文发展脉络
Chat Paper
正在生成论文摘要