Confounding Equilibria for Platforms with Private Information on Promotion Value
WEB AND INTERNET ECONOMICS, WINE 2021(2022)
摘要
Online marketplaces allow consumers to evaluate, compare, and purchase products while providing a channel for third-party sellers to reach a broad consumer base and increase demand for their products. As platforms seek to maintain a large consumer base, many platforms prioritize increasing consumer surplus by offering competitively priced products. At the same time, it is common practice in such marketplaces to let sellers determine their own price, but such flexibility may result in higher prices that reduce consumer surplus. In this paper, we consider a platform facilitating trade between sellers and buyers with the objective of maximizing consumer surplus. Even though in many such marketplaces prices are set by revenue-maximizing sellers, platforms can influence prices through (i) price-dependent promotion policies that can increase demand for a product by featuring it in a prominent position on the webpage and (ii) the information revealed to sellers about the value of being promoted. Identifying effective joint information design and promotion policies is a challenging dynamic problem as sellers can sequentially learn the promotion value from sales observations and update prices accordingly. We introduce the notion of confounding promotion policies, which are designed to prevent a Bayesian seller from learning the promotion value (at the expense of the short-run loss of diverting consumers from the best product offering). Leveraging these policies, we characterize the maximum long-run average consumer surplus that is achievable through joint information design and promotion policies when the seller sets prices myopically. We then establish that these strategies are supported in a Bayesian Nash equilibrium, by showing that the seller's best response to the platform's optimal policy is to price myopically at every history. Moreover, the equilibrium we identify is platform-optimal within the class of horizon-maximin equilibria, in which strategies are not predicated on precise knowledge of the horizon length, and are designed to maximize payoff over the worst-case horizon. Our analysis allows one to identify practical long-run average optimal platform policies for a broad range of demand models.
更多查看译文
关键词
Information design, Bayesian learning, Online marketplaces
AI 理解论文
溯源树
样例
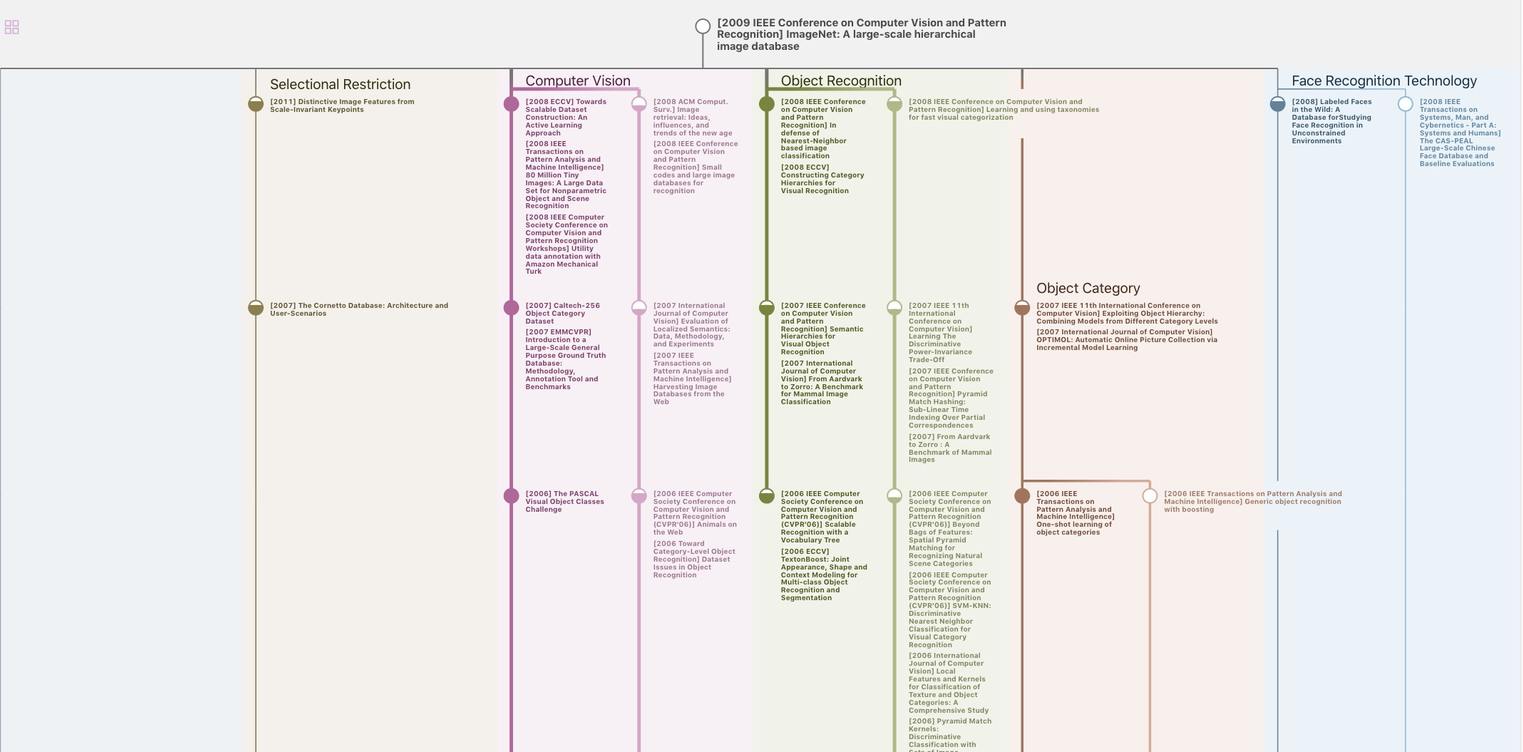
生成溯源树,研究论文发展脉络
Chat Paper
正在生成论文摘要