Unidentifiability of errors-in-variables models with rank deficiency from measurements
Measurement(2022)
摘要
Although errors-in-variables (EIV) models have been substantially investigated, rank deficient EIV models have not been properly addressed. This paper proves the following major results: (i) given a full EIV model that the elements of its coefficient matrix are continuous random variables with a positive definite variance–covariance matrix, such an EIV model cannot be rank deficient with probability one, indicating that the non-uniqueness condition of total least squares solutions by Golub and van Loan [1] and van Huffel and Vandewalle [2], [3] cannot be true with probability one; (ii) even if a full EIV model is theoretically rank deficient, its rank deficiency cannot be identifiable from measurements with probability one. A full EIV model with theoretical rank deficiency can almost always be incorrectly identified to be an estimable model with probability one, due to the randomness of the coefficient matrix; and (iii) if an EIV model is rank deficient, it must be a partial EIV model, though a partial EIV model is not necessarily rank deficient. Further, if a partial EIV model is rank deficient, we may not numerically obtain its exact rank. These theoretical results have a practical engineering implication that solutions to a rank deficient EIV model from random measurements can be totally incorrect without the notice of an engineer, since there exist an infinite number of mathematically equivalent solutions to such an EIV model. We propose two methods to solve a partial rank deficient EIV model for a unique solution by imposing extra subjective information.
更多查看译文
关键词
Identifiability,Nonlinear adjustment,Random matrix,Total least squares
AI 理解论文
溯源树
样例
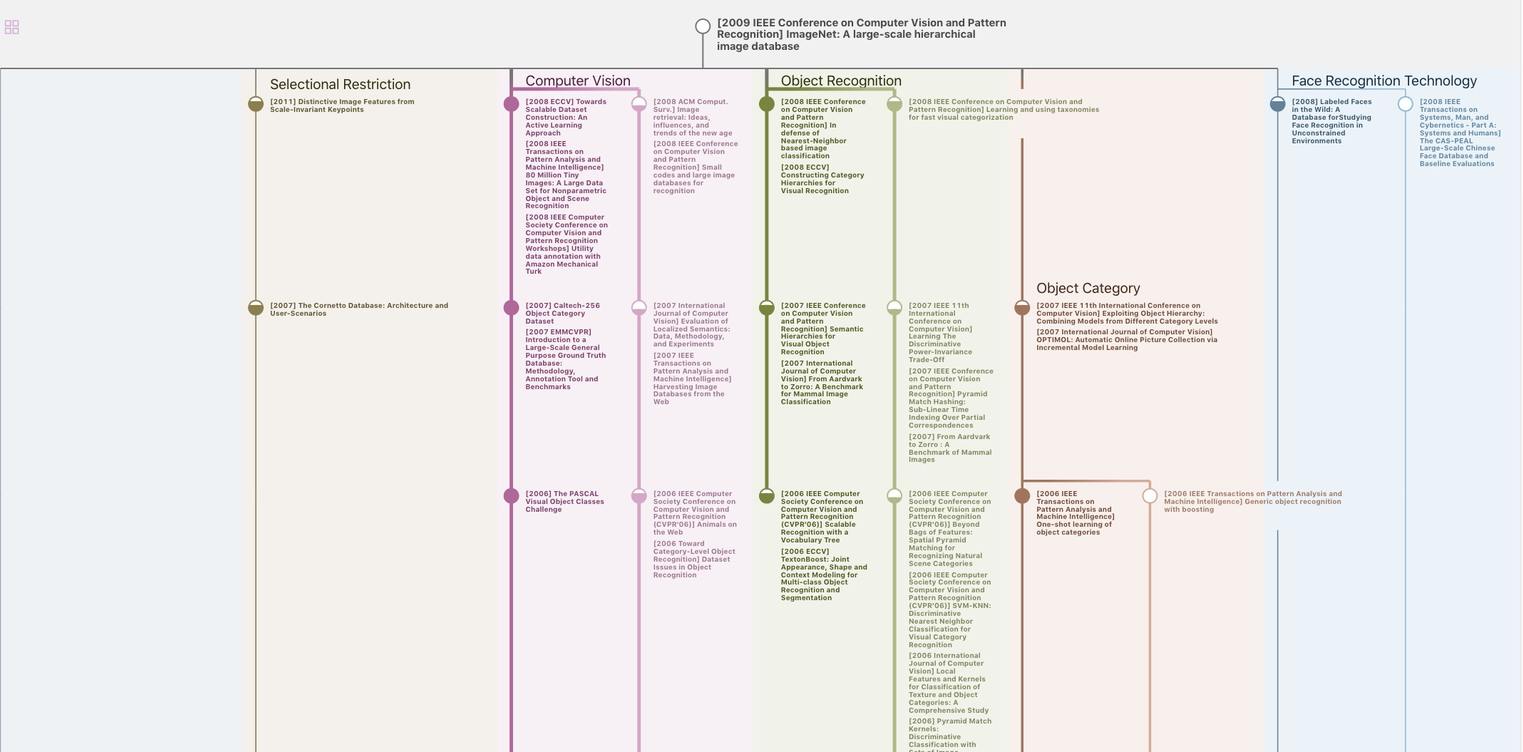
生成溯源树,研究论文发展脉络
Chat Paper
正在生成论文摘要