SEQ2SEQ++: A Multitasking-Based Seq2seq Model to Generate Meaningful and Relevant Answers
IEEE ACCESS(2021)
摘要
Question-answering chatbots have tremendous potential to complement humans in various fields. They are implemented using either rule-based or machine learning-based systems. Unlike the former, machine learning-based chatbots are more scalable. Sequence-to-sequence (Seq2Seq) learning is one of the most popular approaches in machine learning-based chatbots and has shown remarkable progress since its introduction in 2014. However, chatbots based on Seq2Seq learning show a weakness in that it tends to generate answers that can be generic and inconsistent with the questions, thereby becoming meaningless and, therefore, may lower the chatbot adoption rate. This weakness can be attributed to three issues: question encoder overfit, answer generation overfit, and language model influence. Several recent methods utilize multitask learning (MTL) to address this weakness. However, the existing MTL models show very little improvement over single-task learning, wherein they still generate generic and inconsistent answers. This paper presents a novel approach to MTL for the Seq2Seq learning model called SEQ2SEQ++, which comprises a multifunctional encoder, an answer decoder, an answer encoder, and a ternary classifier. Additionally, SEQ2SEQ++ utilizes a dynamic tasks loss weight mechanism for MTL loss calculation and a novel attention mechanism called the comprehensive attention mechanism. Experiments on NarrativeQA and SQuAD datasets were conducted to gauge the performance of the proposed model in comparison with two recently proposed models. The experimental results show that SEQ2SEQ++ yields noteworthy improvements over the two models on bilingual evaluation understudy, word error rate, and Distinct-2 metrics.
更多查看译文
关键词
Task analysis, Chatbots, Computational modeling, Decoding, Training, Transformers, Benchmark testing, Sequence to sequence learning, natural answer generation, multitask learning, attention mechanism
AI 理解论文
溯源树
样例
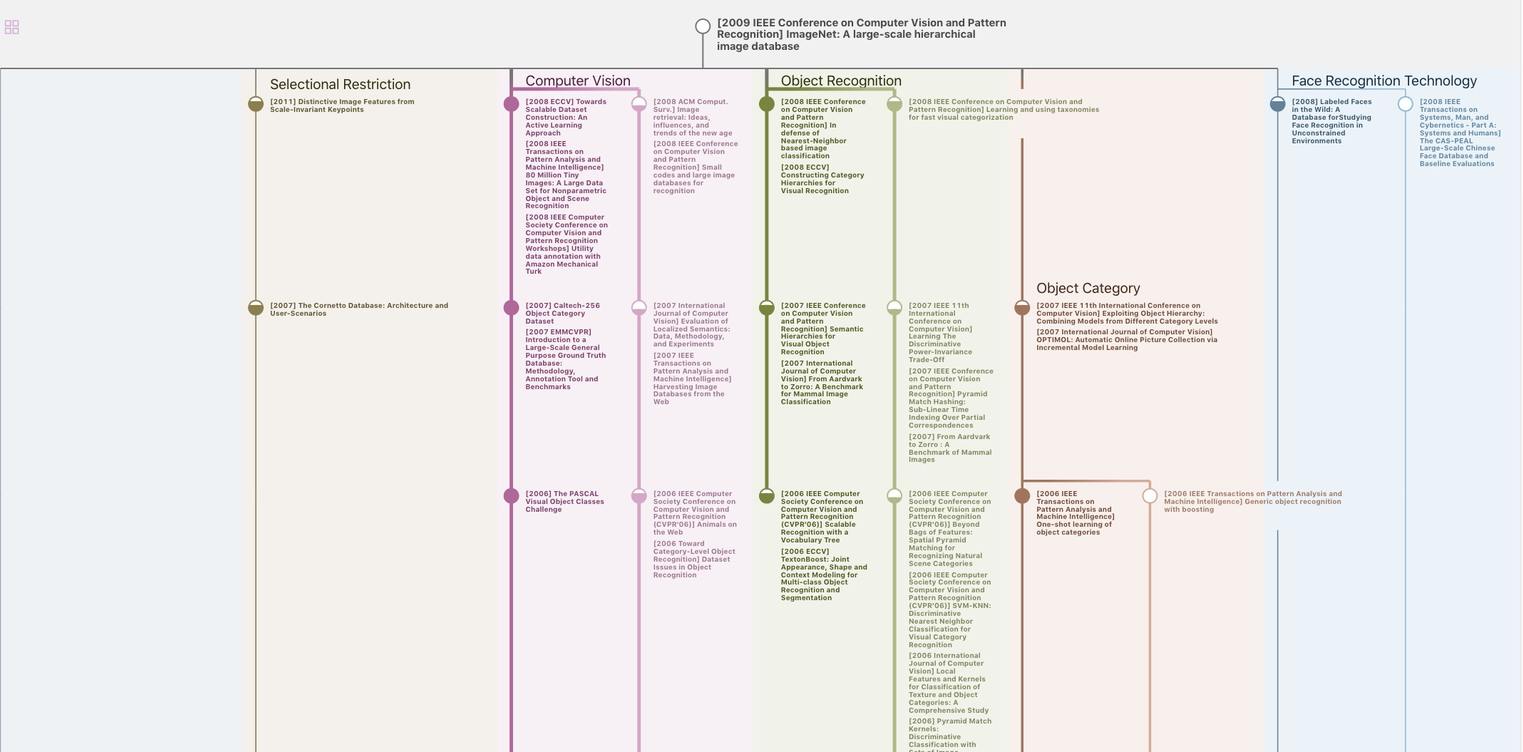
生成溯源树,研究论文发展脉络
Chat Paper
正在生成论文摘要