Identifying Abnormal CFRP Holes Using Both Unsupervised and Supervised Learning Techniques on In-Process Force, Current, and Vibration Signals
International Journal of Precision Engineering and Manufacturing(2022)
摘要
This study aims to conduct abnormality detection by applying machine learning algorithms when drilling a carbon fiber reinforced plastic laminate. In-process signals including current, thrust force, and vibration were captured during the dry drilling experiments using a 6 mm physical vapor deposit diamond-coated drill at the consistent spindle speed of 6500 RPM and 0.05 mm/rev. Across measurements from out-of-process variables, including hole diameter, roundness, surface roughness, entry/exit delamination, and entry/exit uncut fiber area, in-process measurements were most able to find outliers with respect to diameter. Both Principal Component Analysis, an unsupervised dimensionality reduction technique, and Linear Discriminant Analysis, a supervised dimensionality reduction technique, could separate oversize or undersize holes from average-sized holes when using fast Fourier transformation data of in-process vibration. Predictive performance with k-Nearest Neighbors shows that our machine learning pipeline can predict oversized vs. non-oversized holes with over 85% accuracy in this dataset. Peak prediction performance is obtained when in-process measurement data is viewed from the frequency domain, and predictions are weighted based on the relative distances of the nearest neighbors.
更多查看译文
关键词
CFRP drilling, Machine learning, Unsupervised learning, Supervised learning, Abnormality detection, Hole quality
AI 理解论文
溯源树
样例
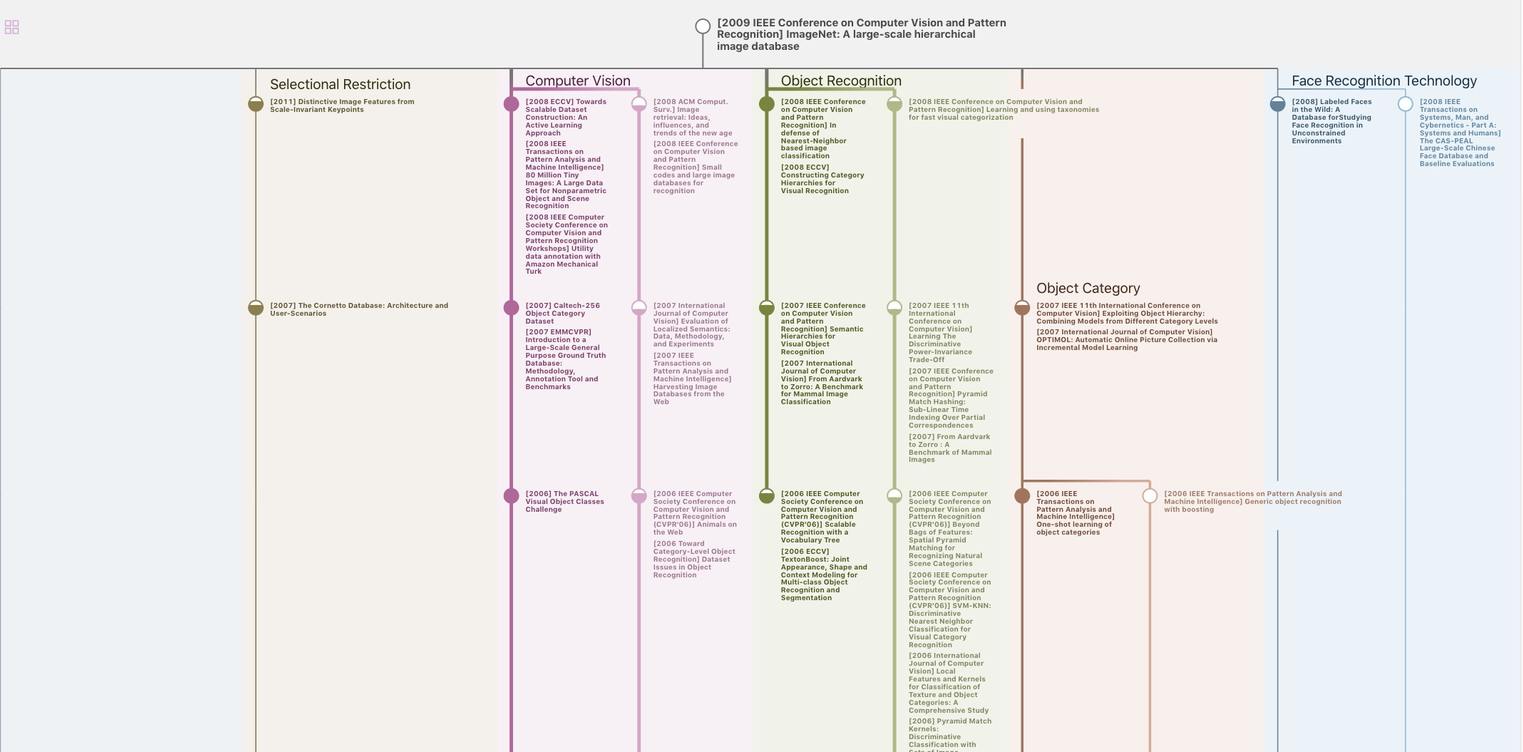
生成溯源树,研究论文发展脉络
Chat Paper
正在生成论文摘要