Online Advertising Incrementality Testing: Practical Lessons, Paid Search and Emerging Challenges
Advances in Information Retrieval(2022)
摘要
Online advertising has historically been approached as an ad-to-user matching problem within sophisticated optimization algorithms. As the research and ad tech industries have progressed, advertisers have increasingly emphasized the causal effect estimation of their ads (incrementality) using controlled experiments (A/B testing). With low lift effects and sparse conversion, the development of incrementality testing platforms at scale suggests tremendous engineering challenges in measurement precision. Similarly, the correct interpretation of results addressing a business goal requires significant data science and experimentation research expertise. We propose a practical tutorial in the incrementality testing landscape, including: – The business need – Literature solutions and industry practices – Designs in the development of testing platforms – The testing cycle, case studies, and recommendations – Paid search effectiveness in the marketplace – Emerging privacy challenges for incrementality testing and research solutions We provide first-hand lessons based on the development of such a platform in a major combined DSP and ad network, and after running several tests for up to two months each over recent years. With increasing privacy constraints, we survey literature and current practices. These practices include private set union and differential privacy for conversion modeling, and geo-testing combined with synthetic control techniques.
更多查看译文
关键词
advertising,testing,paid search
AI 理解论文
溯源树
样例
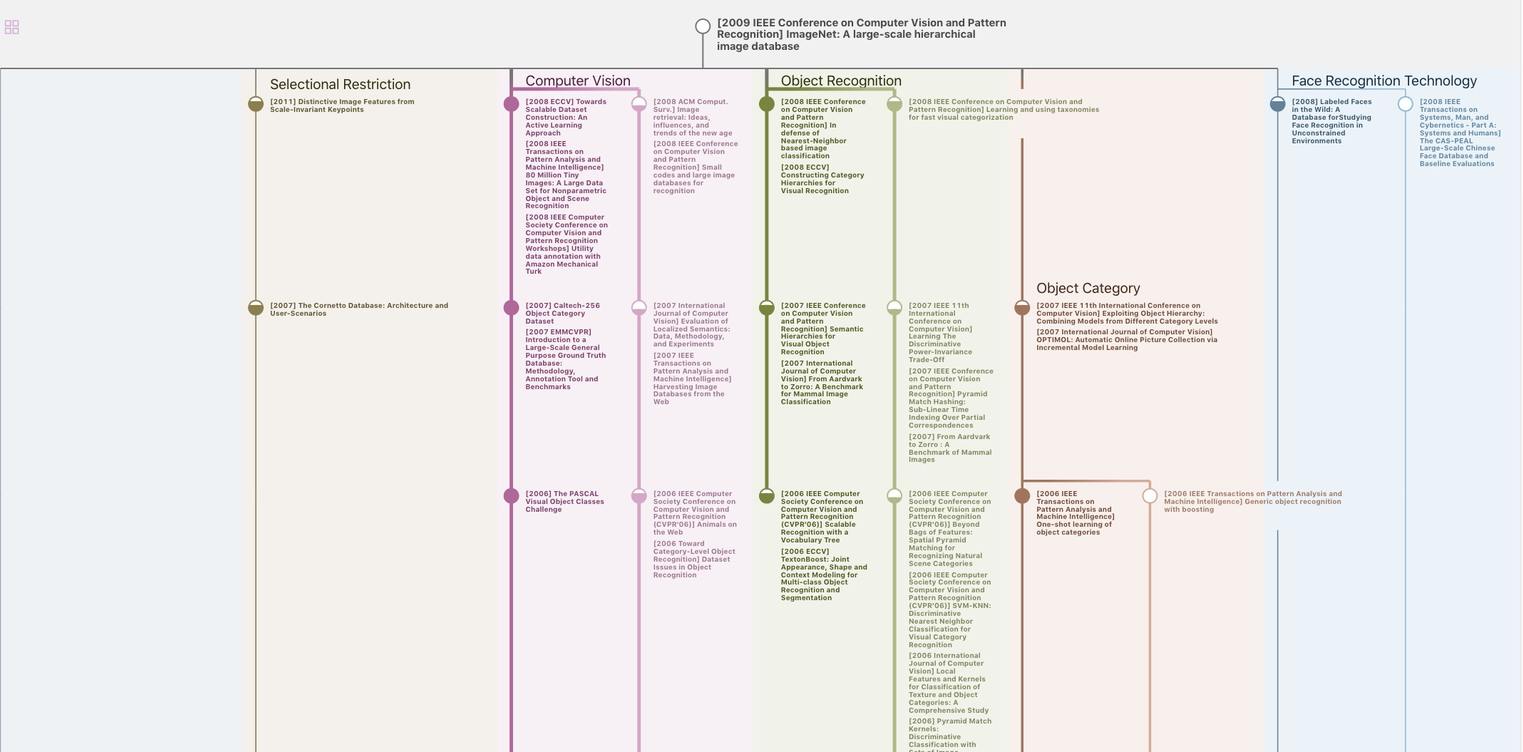
生成溯源树,研究论文发展脉络
Chat Paper
正在生成论文摘要