Deep Learning Based Volume Fraction Estimation for Two-Phase Water-Containing Flows
2021 IEEE SENSORS(2021)
摘要
The estimation of water volume fraction in water-containing multiphase flows is important for several industrial applications. There are many published works detailing with methods to estimate and monitor water-containing multiphase flows based on different sensor modalities, including electrical capacitance tomography (ECT). A recently developed method based on ECT sensors to estimate the water volume fraction in water-containing two-phase flows utilizes the Hanai's mixture formula. Fresh or tap water is conductive and has large electrical permittivity, thus making the ECT-based estimation problem highly nonlinear and challenging to solve. Most of these works assume that the mixture state of water in the two-phase flow is known as either continuous or disperse. However, in practice, the state of water might not be known a priori. In this work, we propose a deep learning based approach to classify water-containing flows into water-dispersed or water-continuous flows and to estimate the water volume fraction present in the flow.
更多查看译文
关键词
ECT, water-containing, multiphase-flows, deep learning, DNN, low-frequency, regression, classification
AI 理解论文
溯源树
样例
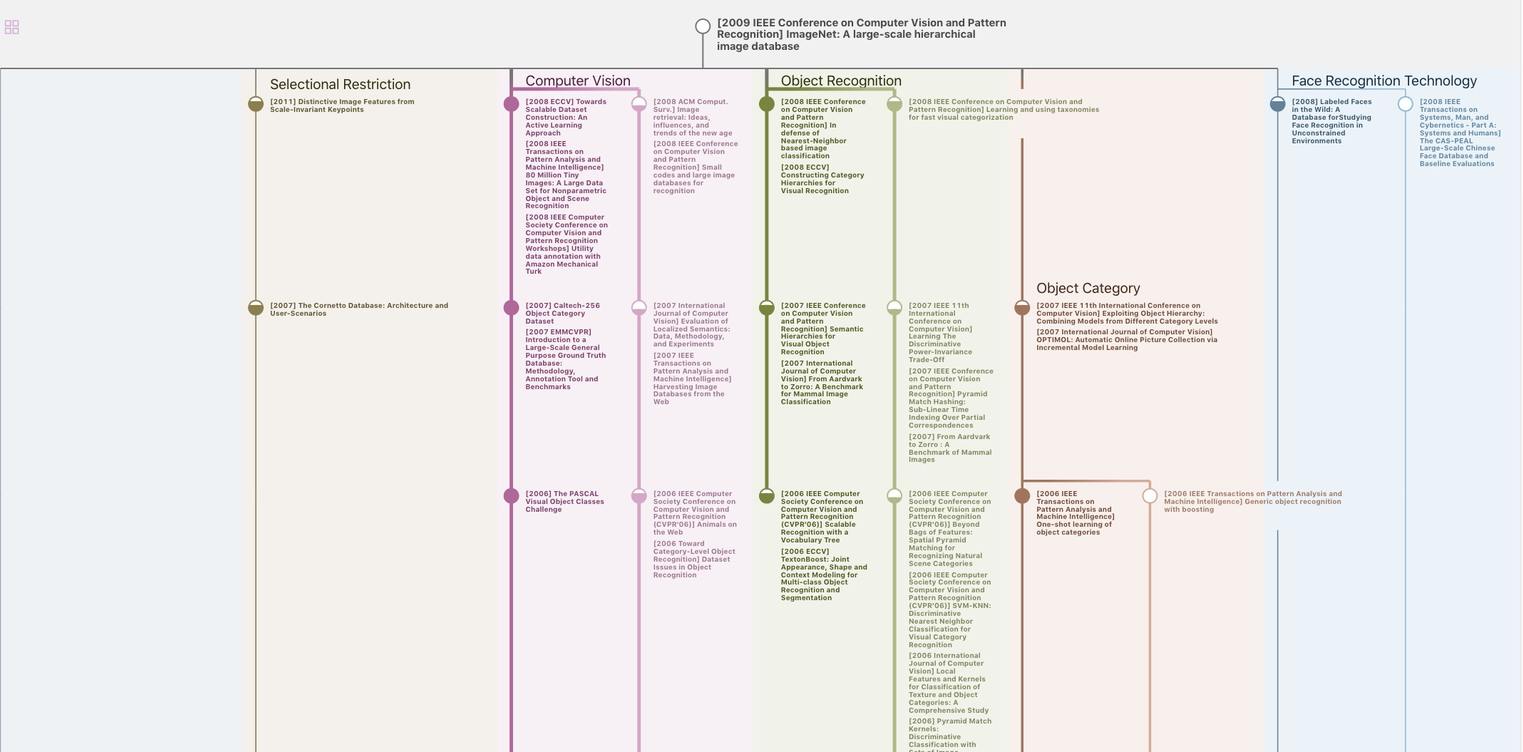
生成溯源树,研究论文发展脉络
Chat Paper
正在生成论文摘要