Assessing temporal biases across aggregated historical spatial data: a case study of North Carolina's freshwater fishes
ECOSPHERE(2021)
摘要
Historical records from museums or government agencies are of tremendous utility for illuminating the factors that shape the spatial distribution of the planet's biodiversity. However, these data were often collected under heterogeneous and opportunistic sampling designs and therefore likely contain significant sampling biases that change over time. Understanding historical biases is particularly important for aquatic vertebrates, where no studies of changes in sampling effort have yet been conducted. Here, we use a dataset of 276,138 records that span all freshwater fishes known to occur in North Carolina as a case study from which to highlight major shifts in collection trends that cause sample biases in datasets that aggregate historical records. We found evidence for three distinct phases of sampling over the last two centuries: (1) early sampling in the late 19th and early 20th century that was largely dominated by the research interests of a few "mega-collectors"; (2) a mid-20th century shift toward more widespread sampling; and (3) a major surge of sampling that corresponds to the rise of major environmental movements. We find each period possesses distinct phylogenetic and spatial biases. Moreover, these phases mirror trends in other spatial datasets that aggregate historical records spanning plants to terrestrial vertebrates, thereby suggesting that historical contingency and a temporal bias toward recent records are likely hallmarks of compiled historical datasets. Given that the pace of spatial data sampling continues to grow, our results strongly caution that the continued development of new models and methods to mitigate against bias-driven statistical artifacts will be critical to effectively harnessing the power of historical data.
更多查看译文
关键词
biodiversity survey, camera trap surveillance, environmental DNA, environmental niche modeling, museum records
AI 理解论文
溯源树
样例
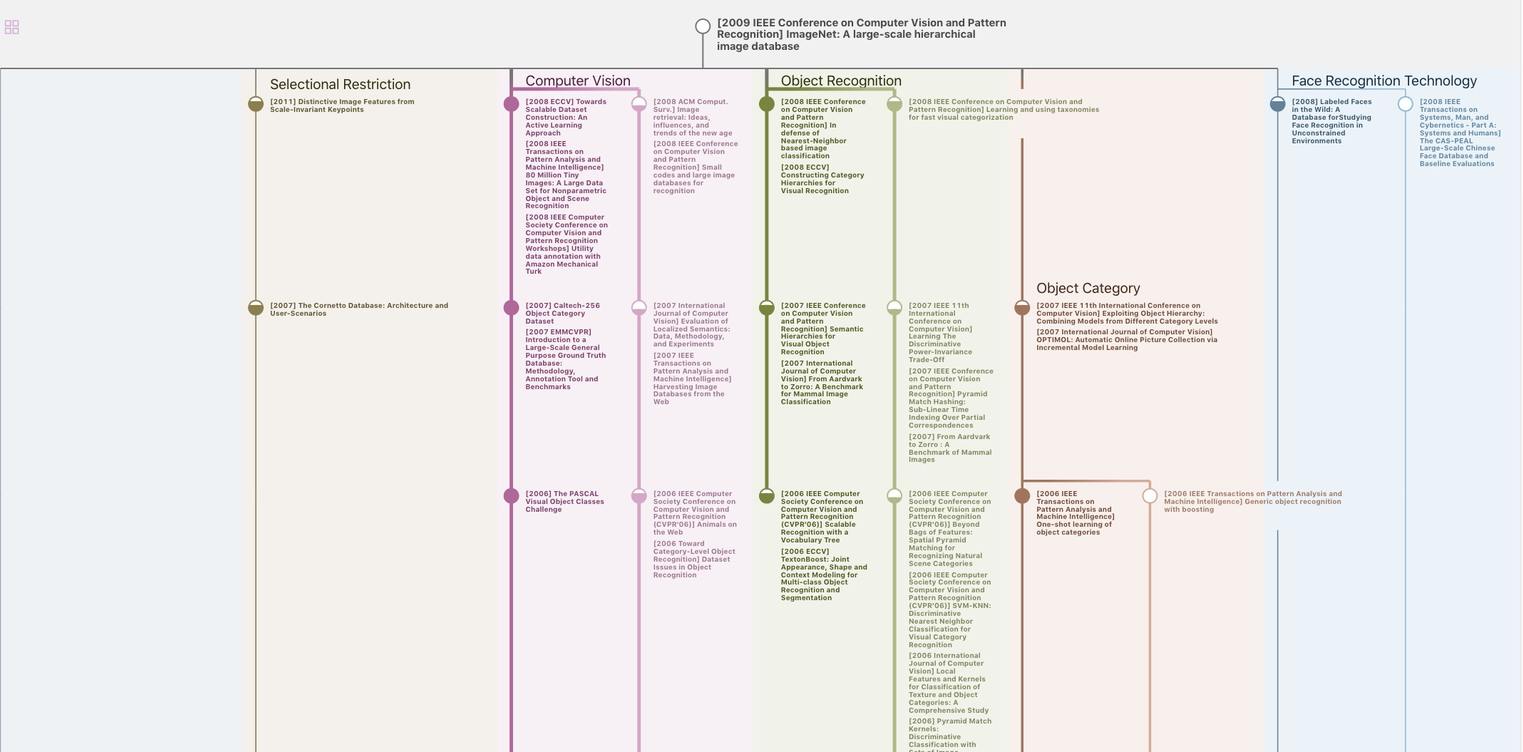
生成溯源树,研究论文发展脉络
Chat Paper
正在生成论文摘要