Data-Driven Identification of Turbulent Oceanic Mixing From Observational Microstructure Data
GEOPHYSICAL RESEARCH LETTERS(2021)
摘要
Characterizing how ocean turbulence transports heat is critically important for accurately parameterizing global circulation models. We present a novel data-driven approach for identifying distinct regions of turbulent mixing within a microstructure data set that uses unsupervised machine learning to cluster fluid patches according to their background buoyancy frequency N, and turbulent dissipation rates of kinetic energy epsilon and thermal variance chi. Applied to data collected near the Velasco Reef in Palau, our clustering algorithm discovers spatial and temporal correlations between the mixing characteristics of a fluid patch and its depth, proximity to the reef and the background current. While much of the data set is characterized by the canonical mixing coefficient Gamma = 0.2, elevated local mixing efficiencies are identified in regions containing large density fluxes derived from chi. Once applied to further datasets, unsupervised machine learning has the potential to advance community understanding of global patterns and local characteristics of turbulent mixing. Plain Language Summary Turbulent fluid motions enhance the mixing of heat between different layers of the ocean, playing a critical role in driving large-scale currents that influence the Earth's climate. Measurements of centimeter-scale velocity and temperature fluctuations within the ocean, termed microstructure, are used to study the properties of turbulent mixing and characterize its variability in both space and time. We present a new technique for analyzing microstructure data that uses a machine learning algorithm to identify fluid regions with similar measured characteristics automatically, yielding insight into the underlying turbulent mechanisms driving mixing. Applied to a data set collected near the Velasco Reef in Palau, our algorithm highlights several distinct turbulent regions whose properties depend on their depth, proximity to the reef and the background current. Our findings demonstrate that machine learning is a valuable new technique for characterizing ocean turbulence that, applied to further data sets, has the potential to advance our understanding of global patterns and local characteristics of turbulent mixing.
更多查看译文
AI 理解论文
溯源树
样例
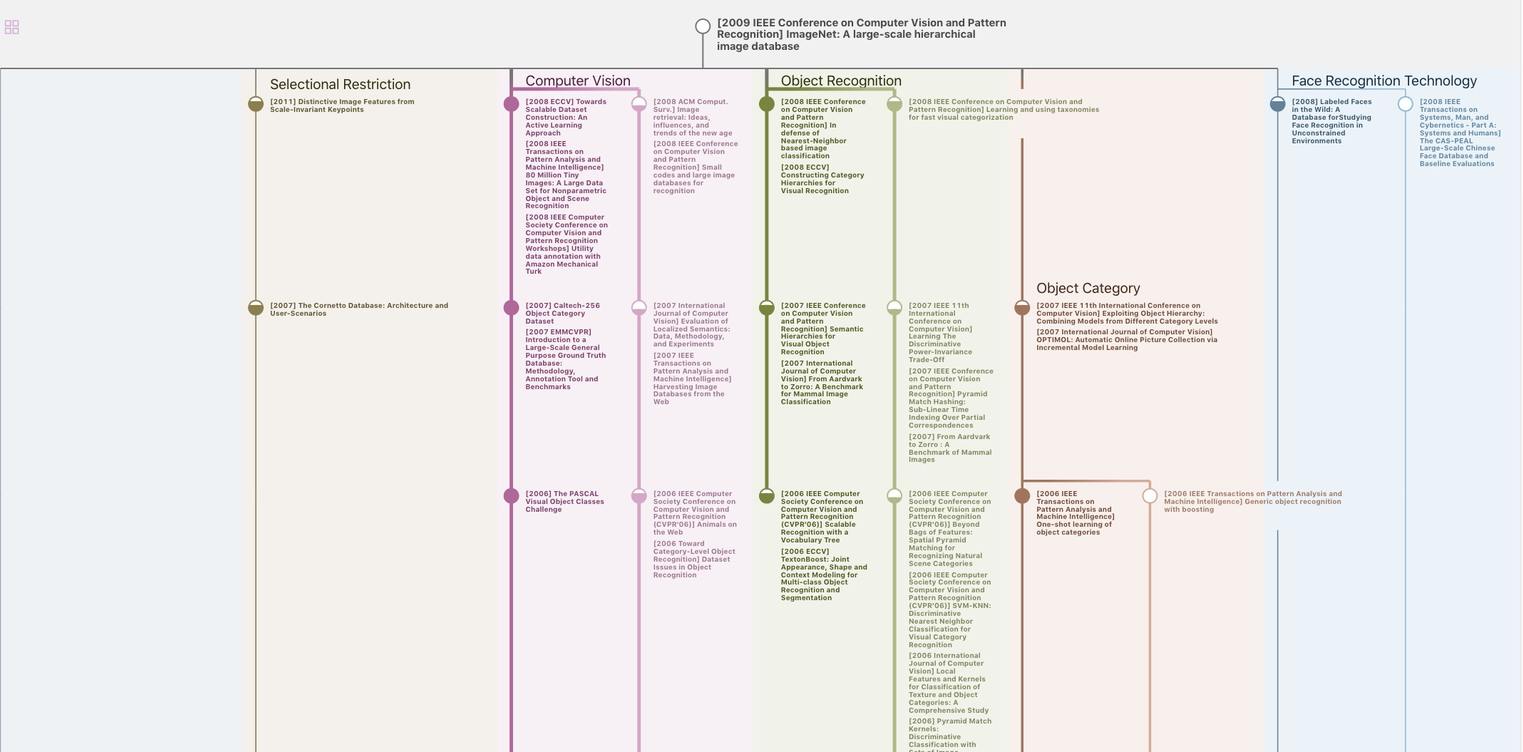
生成溯源树,研究论文发展脉络
Chat Paper
正在生成论文摘要