Landslide susceptibility mapping: improvements in variable weights estimation through machine learning algorithms—a case study of upper Indus River Basin, Pakistan
ENVIRONMENTAL EARTH SCIENCES(2022)
摘要
The northern region of Pakistan is a top tourist destination that is highly susceptible to landslides. Current mega infrastructure development projects in the region have further boosted the importance of the area at national and international scales, yet detailed studies of landslide susceptibility in upper Indus River Basin (UIRB) is still lacking. The aim of this study is to generate and compare landslide susceptibility maps from machine learning algorithms (MLAs) and a traditional geographic information system (GIS) based approach. Past landslide locations are used for model training and testing with data of eleven controlling factors in MLAs, including random forest (RF), support vector machine (SVM), and Naïve Bayes (NB) classifiers. Among the three MLAs, average accuracy of RF model is found between 89 and 90.5%, for SVM the range is between 88 and 90% and for NB the range is between 86 and 87%. The results show that the traditional GIS based weighted overlay technique overestimated vulnerable areas with most of the study area falling in moderate to high susceptible zones. The machine learning models performed much better than the traditional technique as only areas that were identified as most susceptible were locations where landslides had occurred in the past. Within the three ML techniques, RF model’s performance is marginally better than that of the SVM model, but RF and SVM performed significantly better compared to the NB model. The resultant susceptibility maps highlight the areas where safety measures should be taken before installing mega infrastructure projects.
更多查看译文
关键词
Landslides, Pakistan, Machine learning, Random forest, Support vector machine, Naive Bayes
AI 理解论文
溯源树
样例
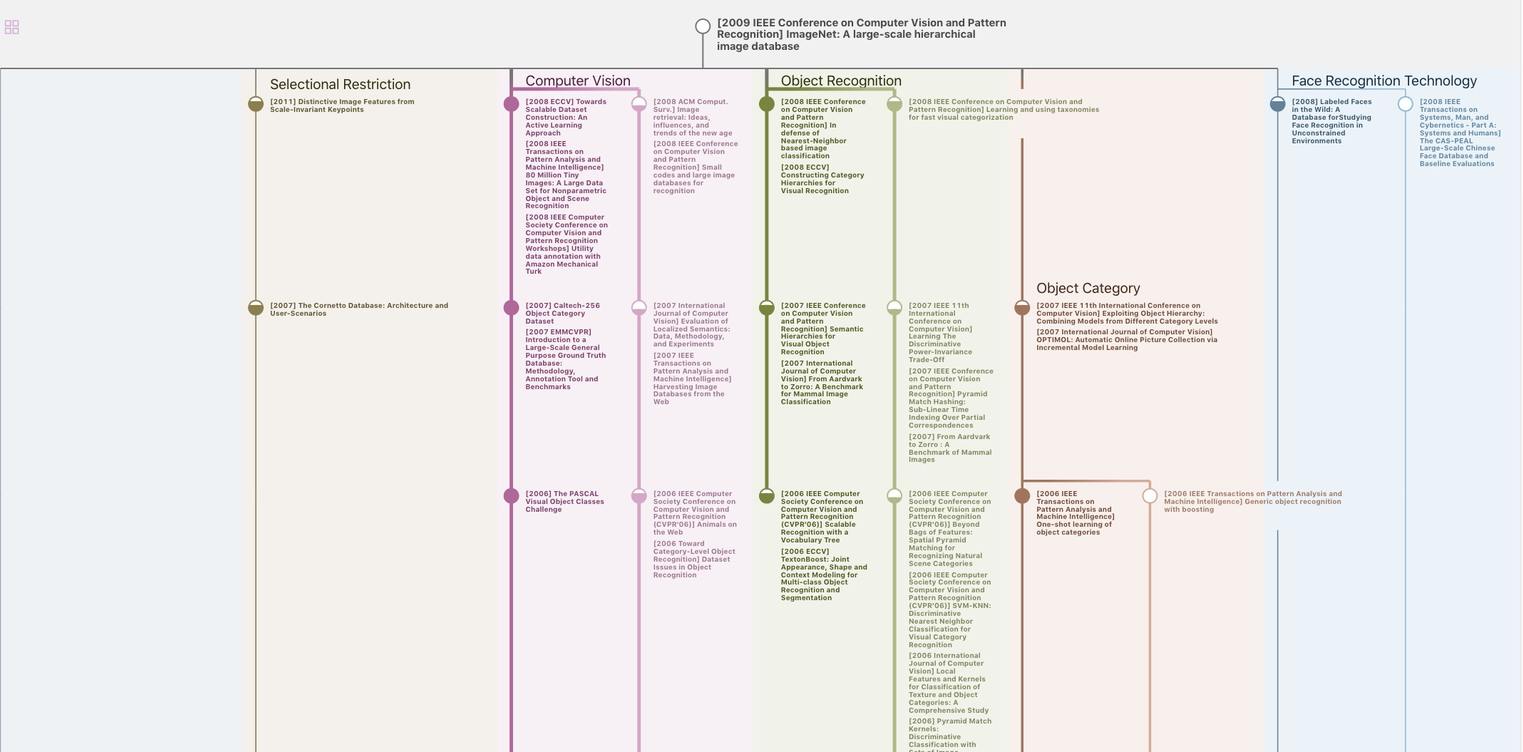
生成溯源树,研究论文发展脉络
Chat Paper
正在生成论文摘要