Cloud detection using convolutional neural networks on remote sensing images
SOLAR ENERGY(2021)
摘要
Cloud detection is an important task for remote sensing and solar resource modeling, and an initial step toward more complex tasks like solar forecasting. Recent advances in remote sensing have increased the spectral, spatial, and temporal resolution of observations. This study demonstrates the image analysis capacity of convolutional neural networks (CNNs) to identify the presence of clouds from remote sensing directly, without ancillary data and at relatively high temporal resolution. Cloud detection in images from the Geostationary Operational Environmental Satellite (GOES)-16 Advanced Baseline Imager (ABI) is validated against ground telemetry from a set of 12 locations of diverse geographic and climatic conditions across the continental U.S. (CONUS). As spatial coverage through ground station networks is typically sparse, transfer learning of baseline models is studied in order to assess the robustness and portability of baseline models through transfer learning scenarios. Performance of the CNN-based cloud mask (CCM) is discussed in three parts: (1) baseline training in comparison to a deterministic model, the ABI cloud mask (ACM), (2) transfer learning of baseline models, and (3) transfer learning of models trained on combined location pairs. In baseline models, the CCM showed average percent improvement over the ACM of 11% in accuracy (ACC) and 30% in Matthews correlation coefficient (MCC) score. In transfer learning scenarios, adding a second location to training samples improved aggregate performance by 18.8%. Results show that CCM transfer learning is highly asymmetric. A framework is proposed to characterize and predict transfer learning performance with respect to this asymmetry.
更多查看译文
关键词
Convolutional neural network, Cloud detection, Remote sensing, Transfer learning, Portability
AI 理解论文
溯源树
样例
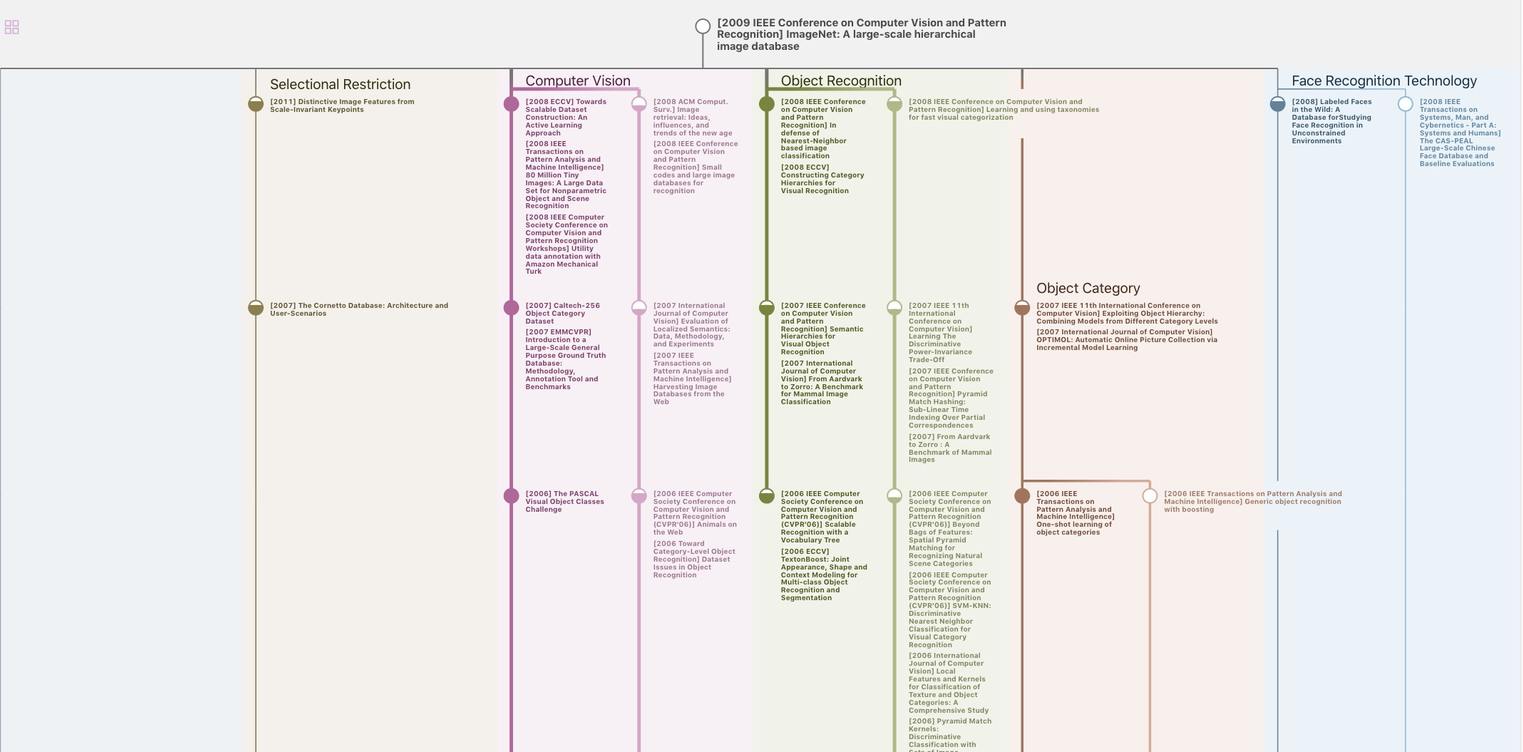
生成溯源树,研究论文发展脉络
Chat Paper
正在生成论文摘要