Nonlinear Predictive Control of a Bioreactor by Surrogate Model Approximation of Flux Balance Analysis
INDUSTRIAL & ENGINEERING CHEMISTRY RESEARCH(2021)
摘要
The application of economic model predictive control (EMPC) techniques in bioprocesses is scarce due to limitations in obtaining accurate dynamic models. Simplified unstructured models (e.g., Monod) can be easily developed, but their prediction capacity is poor. On the other hand, models based on dynamic flux balance analysis (dFBA) of the detailed metabolic network appear as a promising alternative. However, using dFBA inside the controller leads to a bilevel optimization problem, which could require excessive computational effort. In the present work, a control approach is proposed to tackle this limitation. By combining mass balances with a surrogate model for the metabolic network, our approach provides a significant reduction in online computation while still accurately describing the microorganism of interest. A case study of a fed-batch bioreactor of Saccharomyces cerevisiae for ethanol maximization was chosen to test the new approach. First, flux balance analysis simulations with Yeast 8.30 genome-scale model were performed, then a simple polynomial model was fitted to these data by partial least-squares regression (PLSR). The identification step showed that only 11 PLS components were necessary to allow the FBA to be replaced by the surrogate model with a good accuracy. The surrogate model was coupled to the EMPC, and the results were similar to those presented in the literature, where the bilevel optimization problem is explicitly solved. The EMPC was able to compensate for structural errors in the identification process, and it provided a higher ethanol titer in comparison to the open-loop operation. The results showed that applying surrogate models to dFBA is a viable strategy to the solution of a bilevel optimization problem.
更多查看译文
AI 理解论文
溯源树
样例
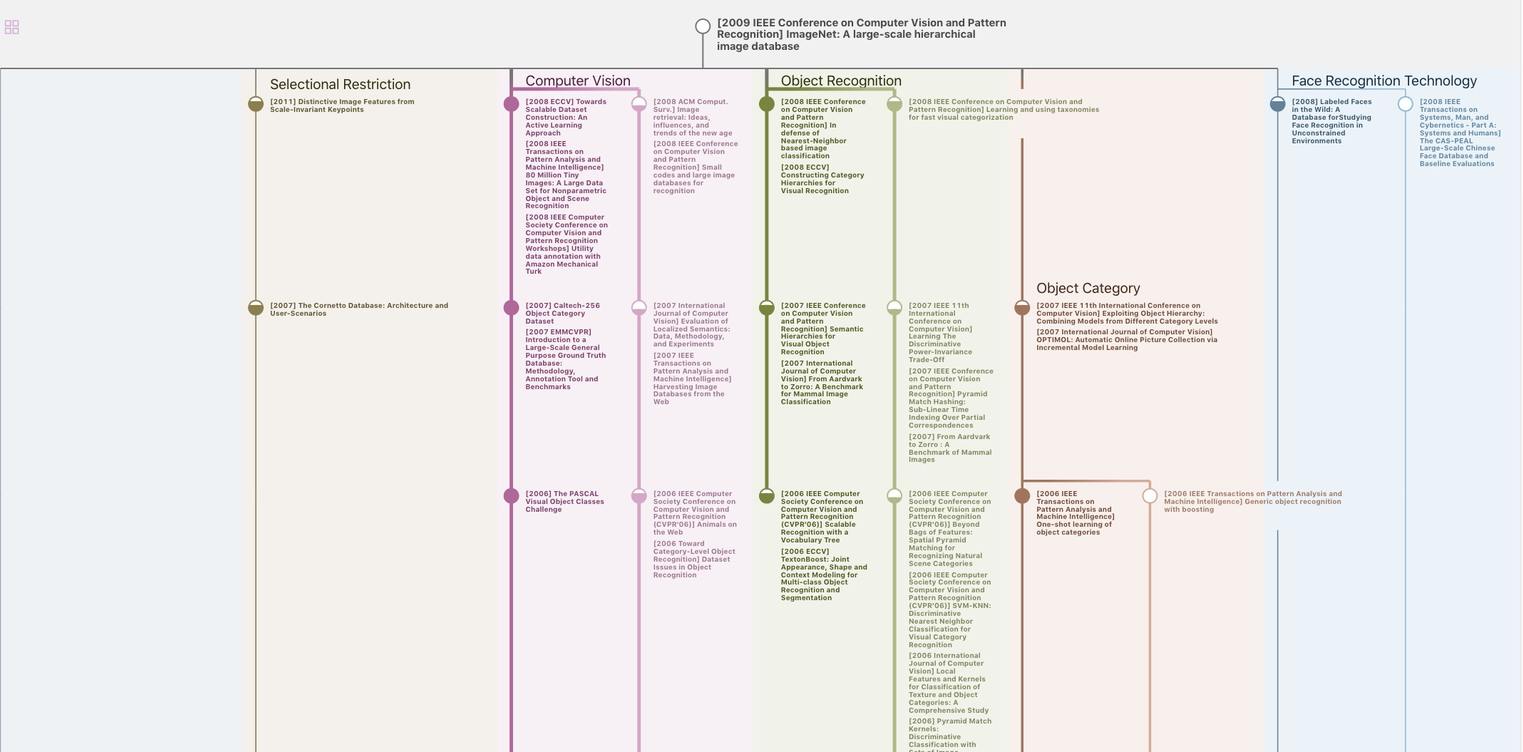
生成溯源树,研究论文发展脉络
Chat Paper
正在生成论文摘要