Minimizing the Late Work of the Flow Shop Scheduling Problem with a Deep Reinforcement Learning Based Approach
APPLIED SCIENCES-BASEL(2022)
摘要
In the field of industrial manufacturing, assembly line production is the most common production process that can be modeled as a permutation flow shop scheduling problem (PFSP). Minimizing the late work criteria (tasks remaining after due dates arrive) of production planning can effectively reduce production costs and allow for faster product delivery. In this article, a novel learning-based approach is proposed to minimize the late work of the PFSP using deep reinforcement learning (DRL) and graph isomorphism network (GIN), which is an innovative combination of the field of combinatorial optimization and deep learning. The PFSPs are the well-known permutation flow shop problem and each job comes with a release date constraint. In this work, the PFSP is defined as a Markov decision process (MDP) that can be solved by reinforcement learning (RL). A complete graph is introduced for describing the PFSP instance. The proposed policy network combines the graph representation of PFSP and the sequence information of jobs to predict the distribution of candidate jobs. The policy network will be invoked multiple times until a complete sequence is obtained. In order to further improve the quality of the solution obtained by reinforcement learning, an improved iterative greedy (IG) algorithm is proposed to search the solution locally. The experimental results show that the proposed RL and the combined method of RL+IG can obtain better solutions than other excellent heuristic and meta-heuristic algorithms in a short time.
更多查看译文
关键词
combinatorial optimization, graph neural network, deep reinforcement learning, flow shop scheduling, late work, pointer network, iterated greedy
AI 理解论文
溯源树
样例
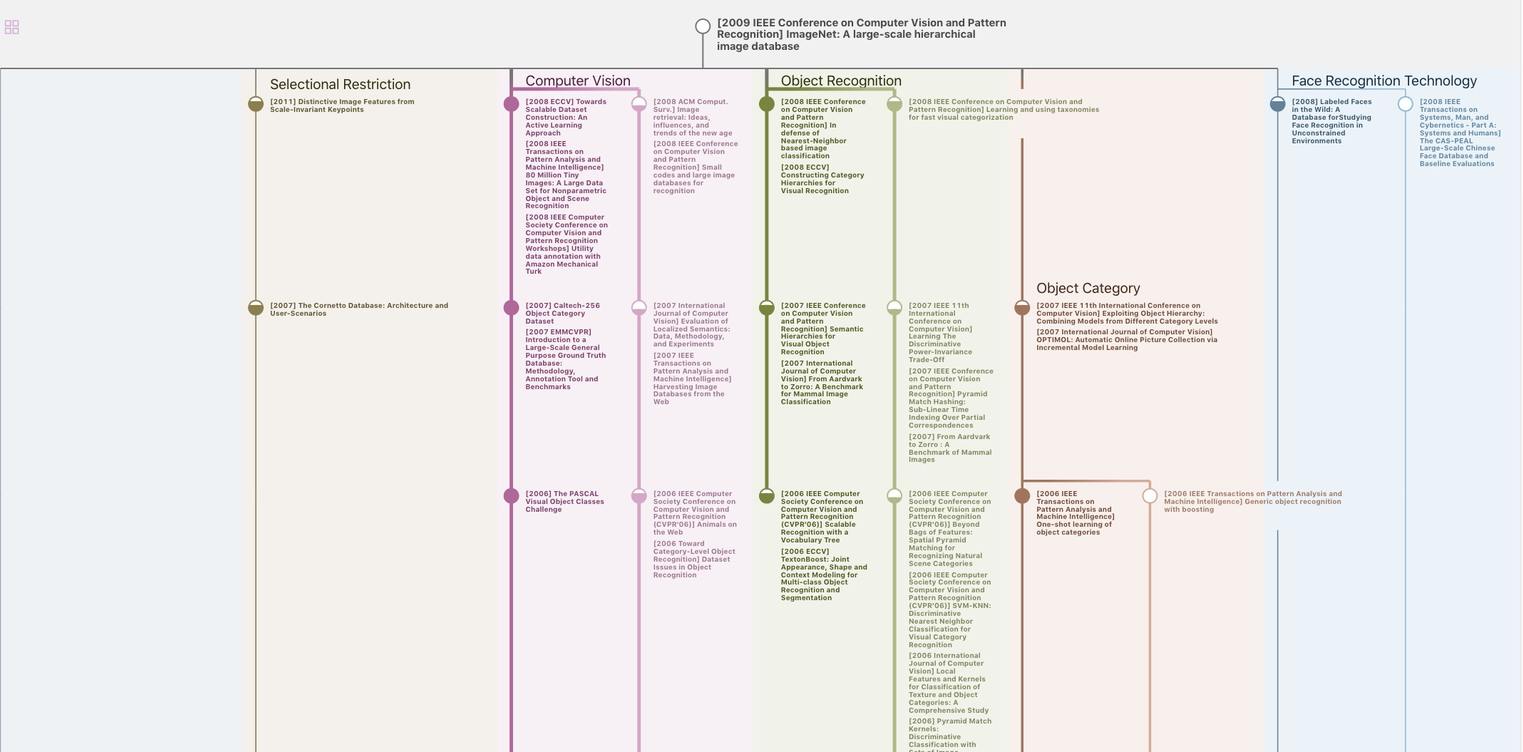
生成溯源树,研究论文发展脉络
Chat Paper
正在生成论文摘要