Theory-guided machine learning to predict density evolution of sand dynamically compacted under K o condition
ACTA GEOTECHNICA(2022)
摘要
This paper introduces a theory-guided machine learning (TGML) framework, which combines a theoretical model (TM) and a machine learning (ML) algorithm to predict compaction density under cyclic loading. Several 1-D tests were conducted on uniformly graded fine sand compacted at varying moisture contents ( w ) , stress levels ( σ_z) and loading frequencies ( f ) , simulating the field compaction of materials using a vibratory roller. The laboratory compaction data were first analysed using a revised TM and an artificial neural network (ANN), and their performance was measured using mean absolute error (MAE). Next, the data were analysed using the TGML framework, which involves three different techniques. TGML1 increased the ML’s ability to extrapolate (MAE improved from 2.2 × 10 −3 to 1.2 × 10 −3 ); TGML2 ensured ML and TM complemented each other to model observations better (MAE improved from 2.3 × 10 −3 to 7.9 × 10 −4 ); and TGML3 assisted in regularising the ML with an additional loss function which ensured the model followed the mechanistic understandings of the underlying physics (MAE improved from 9.2 × 10 −3 to 2.7 × 10 −3 ). Considering TGML3 during modelling is essential when dealing with noisy field datasets, and this is the highlight of this paper. TGML frameworks showed less error and lower model uncertainty, estimated using the novel Monte Carlo dropout technique. Furthermore, the developed TGML framework was used to demonstrate a termination criterion, i.e. the number of cycles of roller movement required to achieve the desired degree of compaction. Finally, an approach is proposed by which a simplified TM and ML model can estimate field compaction behaviour during roller movement.
更多查看译文
关键词
Compaction,Density,Monte Carlo dropout,Termination criteria,Theory-guided machine learning,Uncertainty
AI 理解论文
溯源树
样例
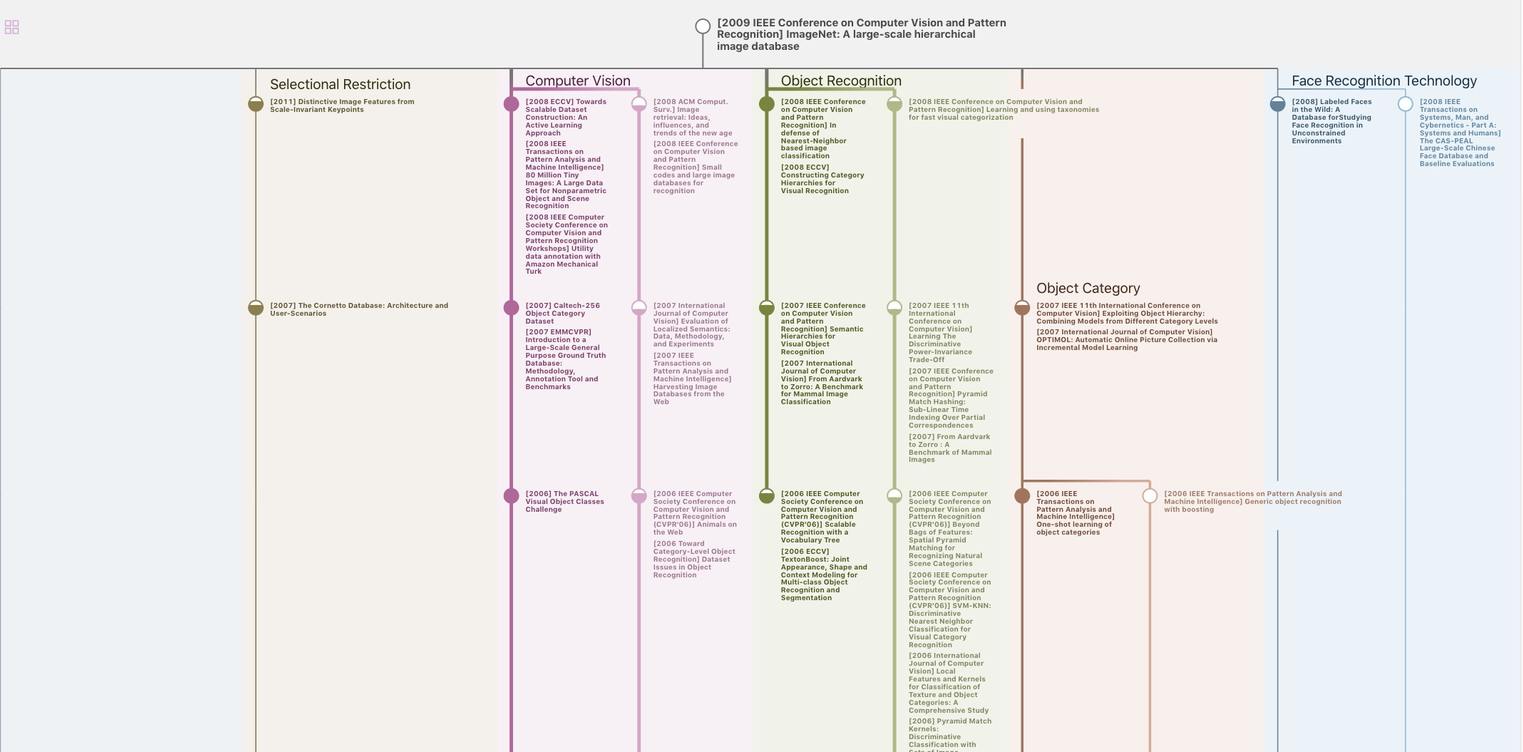
生成溯源树,研究论文发展脉络
Chat Paper
正在生成论文摘要