Preference-driven multi-objective GP search for regression models with new dominance principle and performance indicators
Applied Intelligence(2022)
摘要
Regression is a multi-objective optimization task, which aims to determine accurate and simple relationship expressions between variables. Multi-objective genetic programming (MOGP) methods are popularly-used for regression, which search for trade-off solutions of all objectives, producing a set of solutions (Pareto front). Yet, users normally are not interested in the whole Pareto front and select certain solutions based on preference for specific tasks. There are existing preference-based multi-objective methods. However, existing techniques may not be compliant to Pareto dominance, leading convergence to be deteriorated, or require extra parameters. To handle these issues, a preference-driven dominance (pd-dominance) principle is designed, which is Pareto-compliant and parameterless. Then it is introduced into two base MOGP methods (NSGP (non-dominated sorting genetic programming) and SPGP (strength Pareto genetic programming)) to form two new preference-driven MOGP methods, i.e. pdNSGP and pdSPGP. In addition, three existing performance indicators for multi-objective optimization are improved to adapt to regression tasks. Results show that pdNSGP and pdSPGP outperform MOGP methods with popular preference techniques. For example on the function Dic 3, pdSPGP reaches 3E-2 based on a distance measure (the lower the better); while the reference MOGP methods achieve 1.93E-1 . Moreover, compared with seven reference regression methods, the proposed methods ranks the first three places for most test cases.
更多查看译文
关键词
Multi-objective optimization, Preference, Performance indicator, Genetic programming, Regression
AI 理解论文
溯源树
样例
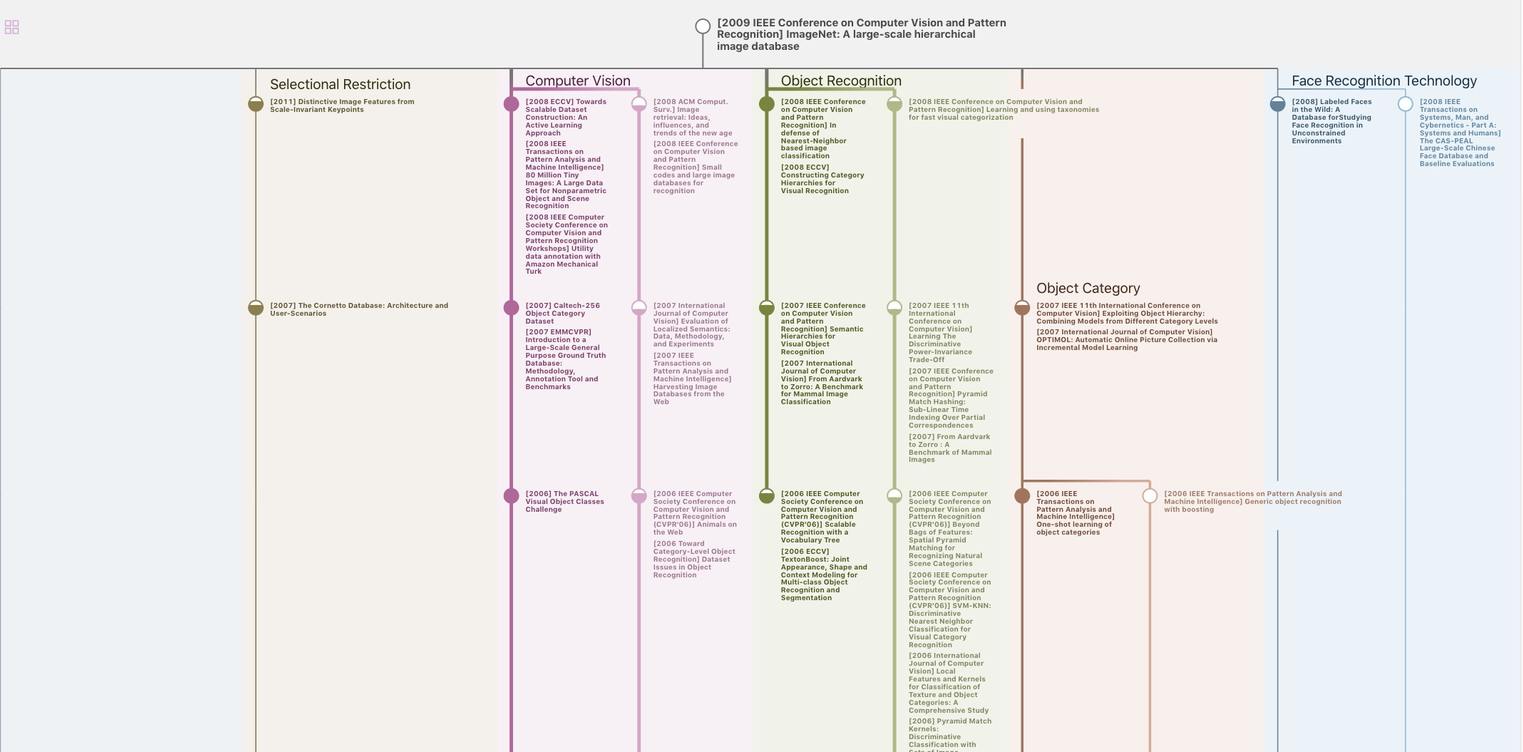
生成溯源树,研究论文发展脉络
Chat Paper
正在生成论文摘要