Using convolutional neural networks to classify melt pools in a pulsed selective laser melting process
Journal of Manufacturing Processes(2022)
摘要
In a selective laser melting (SLM) process, the fluctuation of as-produced part quality remains a problem that hinders its broader industrial applications. In situ monitoring that identifies the variations of melt pool characteristics is promising to improve part quality and control the SLM process. So far, such monitoring approaches have been impeded by the limitation of image processing methods that cannot classify large volumes of melt pool images during a short time. Here we demonstrate that convolutional neural networks (CNN) could be an effective, efficient, and reliable solution to improve the SLM process. In this study, we employ the images captured at five input laser energy levels to train and test CNN models. Compared with a statistical method, the use of CNN has improved the classification of melt pools to the highest achieved accuracy of 96.6%, which is resulted from the ability of utilizing complex image features from the melt pool region. Thus, the CNN is a reliable method with the potential to monitor the influence of other process parameters on melt pool characteristics, potentially leading to the improvement of production in SLM.
更多查看译文
关键词
Selective laser melting (SLM),Melt pool,In situ monitoring,Convolutional neural networks (CNN)
AI 理解论文
溯源树
样例
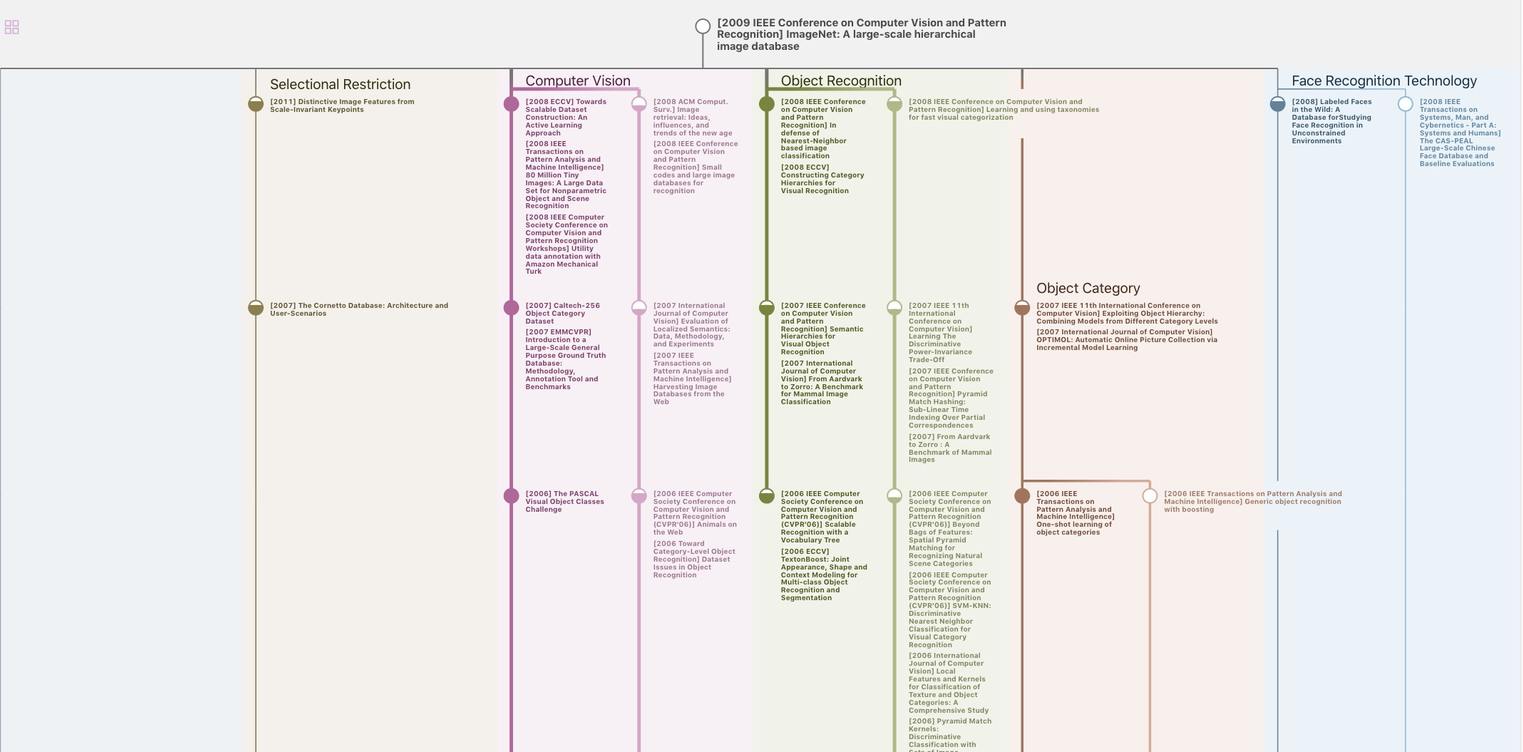
生成溯源树,研究论文发展脉络
Chat Paper
正在生成论文摘要