A hybrid model for water quality parameter prediction based on CEEMDAN-IALO-LSTM ensemble learning
Environmental Earth Sciences(2022)
摘要
Water pollution is a major global environmental issue. Predicting water quality parameters in advance is of utmost importance in the normal operation of society. However, existing empirical models exhibited low precision in water quality prediction due to the non-stationarity and non-linearity of the water quality series, and the performance of the long short-term memory network (LSTM) integrated with the improved meta-heuristic algorithm is still unclear and worth exploring. In this paper, we proposed a hybrid model based on the ensemble learning method that integrates the complete ensemble empirical mode decomposition with adaptive noise (CEEMDAN) and improved LSTM to model and forecast the variation of water quality parameters. First, the water quality series was denoised by an integrated filter based on CEEMDAN and fuzzy entropy (FE), and then, the ant lion algorithm based on chaos initialization, Cauchy mutation, and opposition-based learning (OBL) optimization (IALO) was used to determine the hyperparameters of LSTM. Weekly dissolved oxygen (DO) concentration data, from 1/2010-12/2016, collected at Yongjiang River and Beijiang River gauging stations in the Pearl River Basin were used to validate the effectiveness of the proposed model. The experimental results reveal that the proposed model has better predictive accuracy than other data-driven models because of better error performance, with average MAE , MAPE , and RMSE of 0.42, 5.37%, and 0.53 in the test stage, which is 50.24%, 47.66%, and 49.66% lower than the baseline LSTM model, respectively.
更多查看译文
关键词
IALO,Denoising technology,Water quality prediction,Ensemble learning,Hybrid model
AI 理解论文
溯源树
样例
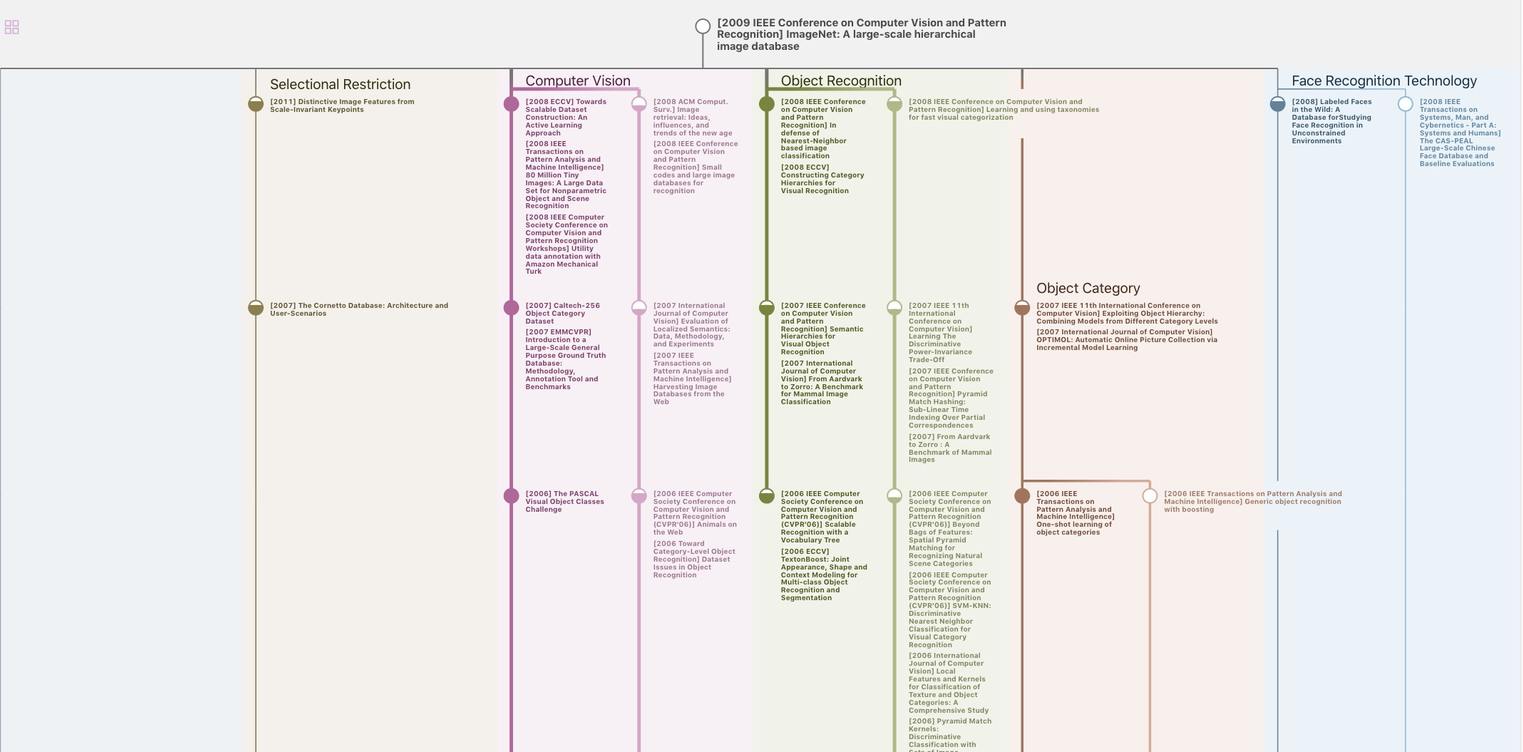
生成溯源树,研究论文发展脉络
Chat Paper
正在生成论文摘要