ARFNet: Attention-Oriented Refinement and Fusion Network for Light Field Salient Object Detection
IEEE Systems Journal(2022)
摘要
Light field salient object detection plays an important role in computer vision tasks because of the excellent performance on challenging scenes. However, the imprecise extraction of saliency features from light field imaging puts a negative impact on the accuracy of saliency results, and the invalid fusion of these extracted features makes saliency results lose details, further cutting down their accuracy. In this article, to effectively extract and fuse saliency features of light field imaging, we propose an attention-oriented refinement and fusion network to solve these problems, which mainly includes the attention-oriented refinement module (ARM) and the attention-oriented fusion module (AFM). Specifically, ARM can precisely refine similar features between focal slices and all-focus image, and fully extract abundant structural characteristics of the light field. Subsequently, AFM can efficiently fuse those features extracted by ARM to obtain saliency results with detail information. Furthermore, different dilated convolution layers embedded with ARM and AFM are leveraged to further capture complete saliency results with detail information. Experimental results demonstrate that our proposed method outperforms other 20 state-of-the-art methods and achieves Top-1 accuracies on three light field datasets.
更多查看译文
关键词
Attention-oriented refinement and fusion network,light field,salient object detection (SOD)
AI 理解论文
溯源树
样例
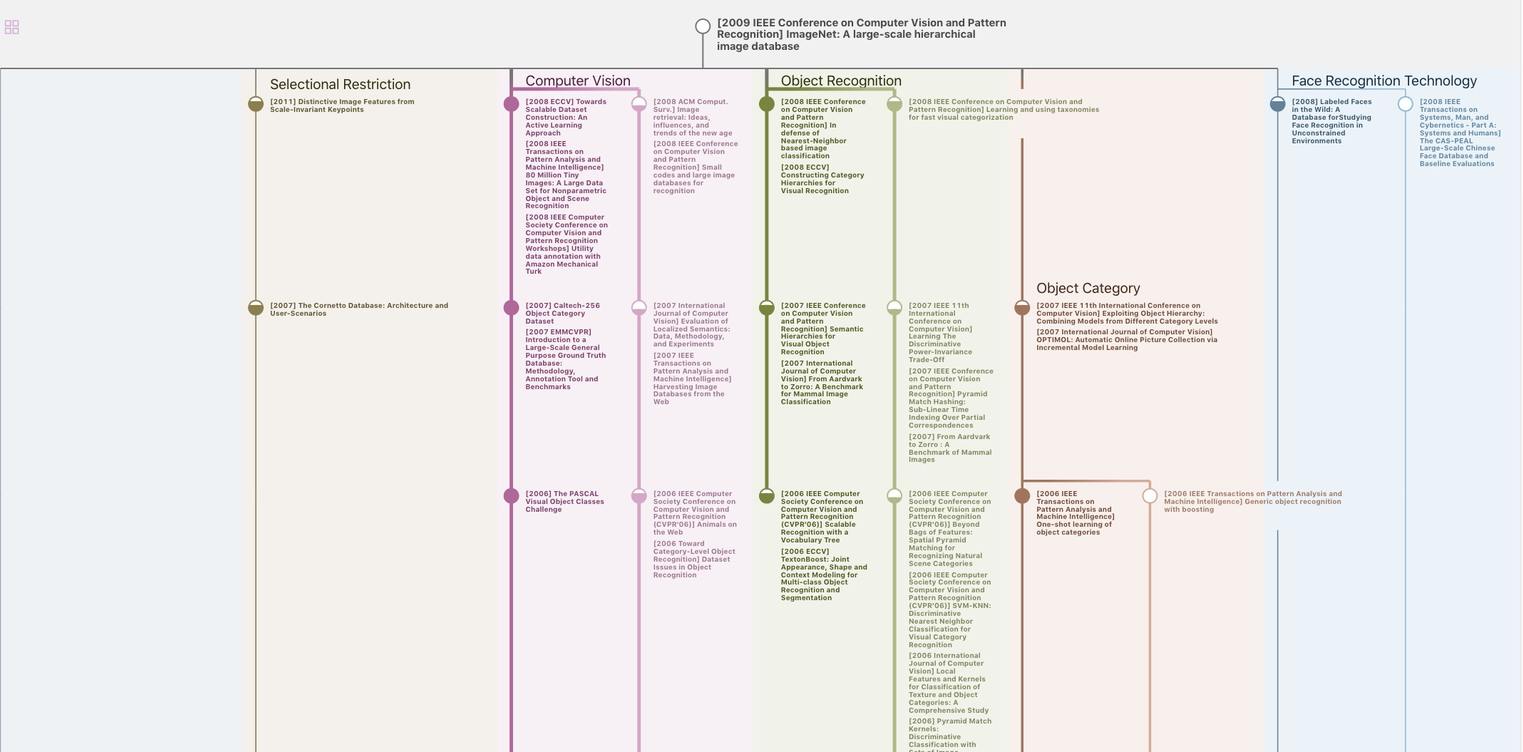
生成溯源树,研究论文发展脉络
Chat Paper
正在生成论文摘要