Comparison of Complex k-Space Data and Magnitude-Only for Training of Deep Learning-Based Artifact Suppression for Real-Time Cine MRI
FRONTIERS IN PHYSICS(2021)
摘要
Propose: The purpose of this study was to compare the performance of deep learning networks trained with complex-valued and magnitude images in suppressing the aliasing artifact for highly accelerated real-time cine MRI. Methods: Two 3D U-net models (Complex-Valued-Net and Magnitude-Net) were implemented to suppress aliasing artifacts in real-time cine images. ECG-segmented cine images (n = 503) generated from both complex k-space data and magnitude-only DICOM were used to synthetize radial real-time cine MRI. Complex-Valued-Net and Magnitude-Net were trained with fully sampled and synthetized radial real-time cine pairs generated from highly undersampled (12-fold) complex k-space and DICOM images, respectively. Real-time cine was prospectively acquired in 29 patients with 12-fold accelerated free-breathing tiny golden-angle radial sequence and reconstructed with both Complex-Valued-Net and Magnitude-Net. Cardiac function, left-ventricular (LV) structure, and subjective image quality [1(non-diagnostic)-5(excellent)] were calculated from Complex-Valued-Net- and Magnitude-Net-reconstructed real-time cine datasets and compared to those of ECG-segmented cine (reference). Results: Free-breathing real-time cine reconstructed by both networks had high correlation (all R-2 > 0.7) and good agreement (all p > 0.05) with standard clinical ECG-segmented cine with respect to LV function and structural parameters. Real-time cine reconstructed by Complex-Valued-Net had superior image quality compared to images from Magnitude-Net in terms of myocardial edge sharpness (Complex-Valued-Net = 3.5 +/- 0.5; Magnitude-Net = 2.6 +/- 0.5), temporal fidelity (Complex-Valued-Net = 3.1 +/- 0.4; Magnitude-Net = 2.1 +/- 0.4), and artifact suppression (Complex-Valued-Net = 3.1 +/- 0.5; Magnitude-Net = 2.0 +/- 0.0), which were all inferior to those of ECG-segmented cine (4.1 +/- 1.4, 3.9 +/- 1.0, and 4.0 +/- 1.1). Conclusion: Compared to Magnitude-Net, Complex-Valued-Net produced improved subjective image quality for reconstructed real-time cine images and did not show any difference in quantitative measures of LV function and structure.
更多查看译文
关键词
real-time cine, artifact suppression, deep learning, complex, magnitude
AI 理解论文
溯源树
样例
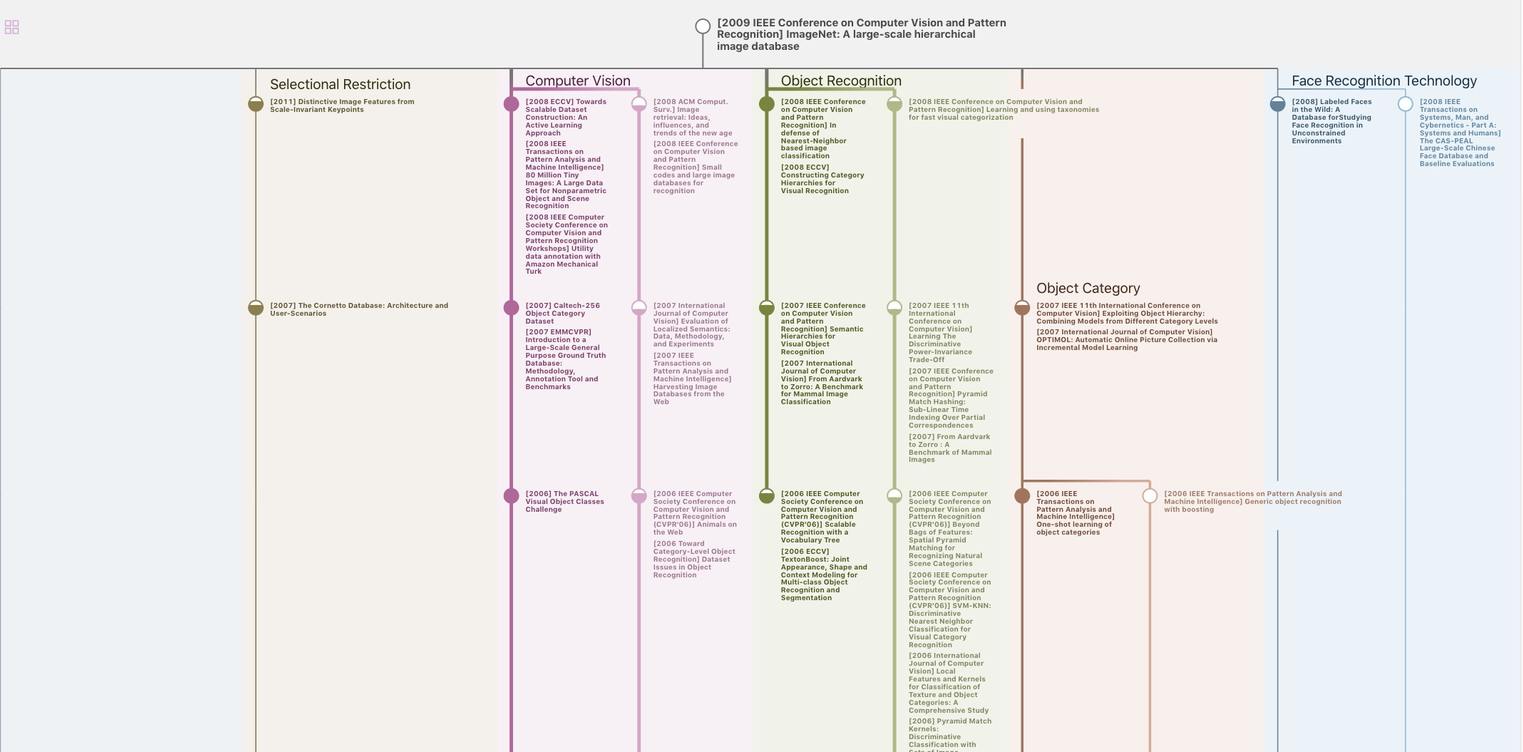
生成溯源树,研究论文发展脉络
Chat Paper
正在生成论文摘要