Opening the black box – Quantile neural networks for loss given default prediction
Journal of Banking & Finance(2022)
摘要
We extend the linear quantile regression with a neural network structure to enable more flexibility in every quantile of the bank loan loss given default distribution. This allows us to model interactions and non-linear impacts of any kind without the need of specifying the exact form beforehand. The precision of the quantile forecasts increases up to 30% compared to the benchmark, especially for higher quantiles which are most important in credit risk. By using a novel feature importance measure, we calculate the strength, direction, interactions and other non-linear impacts for every conditional quantile and every variable. This enables us to explain why our extension exhibits superior performance over the benchmark. Moreover, we find that the macroeconomy is up to two times more important in USA than in Europe and has large joint impacts in both regions. The macroeconomy is most important in the US, whereas in Europe collateralization is essential.
更多查看译文
关键词
Quantile regression,Black box,Neural networks,Explainable machine learning,Global credit data
AI 理解论文
溯源树
样例
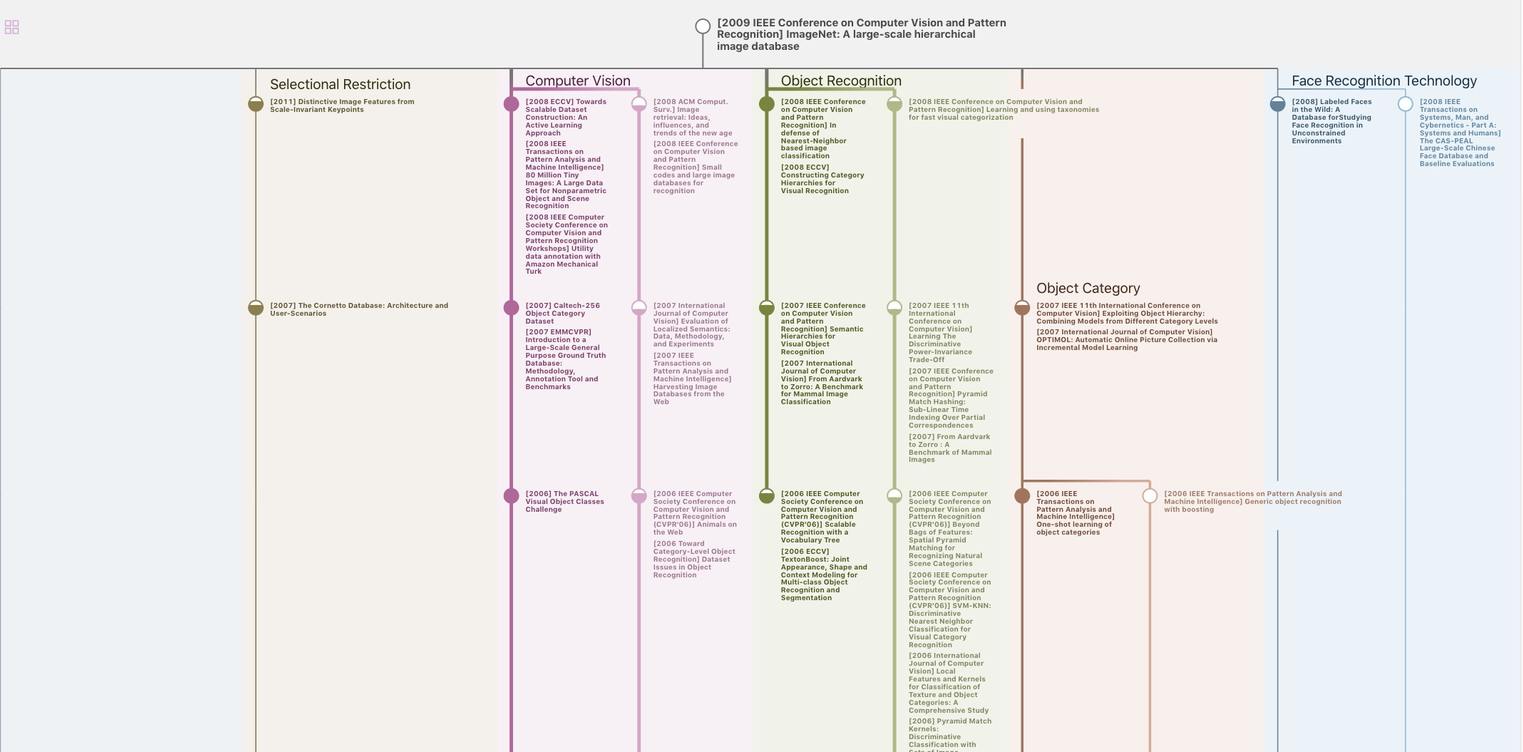
生成溯源树,研究论文发展脉络
Chat Paper
正在生成论文摘要