Aspect-Based Sentiment Analysis With Heterogeneous Graph Neural Network
IEEE Transactions on Computational Social Systems(2023)
摘要
Aspect-based sentiment analysis aims to predict sentiment polarities of given aspects in text. Most current approaches employ attention-based neural methods to capture semantic relationships between aspects and words in one sentence. However, these methods ignore the fact that sentences with the same aspect and sentiment polarity often share the structure and semantic information in a domain, which leads to lower model performance. To mitigate this problem, we propose a heterogeneous aspect graph neural network (HAGNN) to learn the structure and semantic knowledge from intersentence relationships. Our model is a heterogeneous graph neural network since it contains three different kinds of nodes: word nodes, aspect nodes, and sentence nodes. These nodes can pass structure and semantic information between each other and update their embeddings to improve the performance of our model. To the best of our knowledge, we are the first to use a heterogeneous graph to capture relationships between sentences and aspects. The experimental results on five public datasets show the effectiveness of our model outperforming some state-of-the-art models.
更多查看译文
关键词
Aspect-based sentiment analysis (ABSA),aspect-category sentiment analysis (ACSA),heterogeneous graph neural network (GNN)
AI 理解论文
溯源树
样例
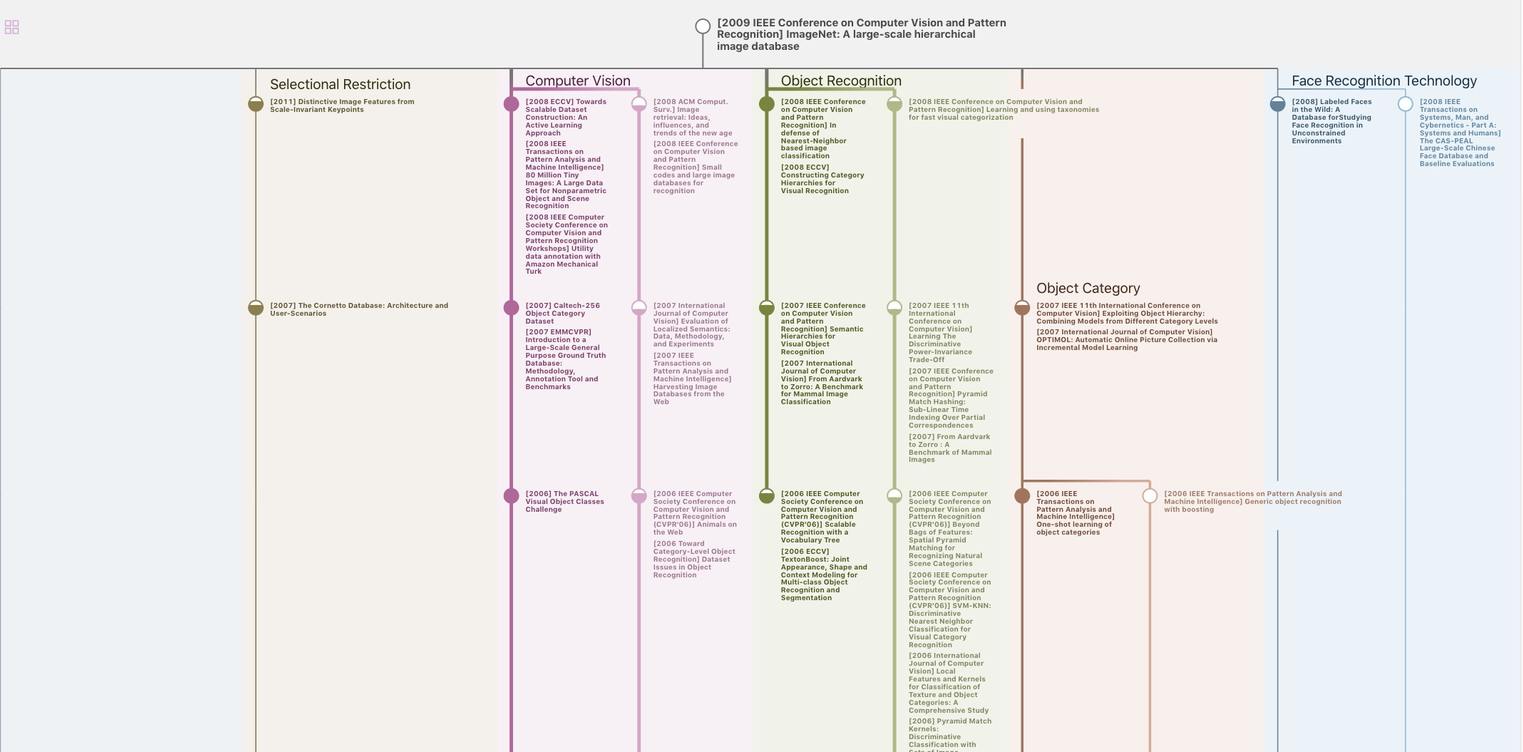
生成溯源树,研究论文发展脉络
Chat Paper
正在生成论文摘要