Combining Different Marker Prioritization Methods in the Analysis of High-density and Sequence Data
JOURNAL OF ANIMAL SCIENCE(2021)
摘要
Abstract High-density and sequence genotypes were expected to increase accuracy of genomic predictions through inclusion of markers in high linkage disequilibrium with causal loci, yet the realized increase has been minimal. Marker preselection has been proposed as a strategy to prioritize the most relevant markers to reduce the dimensionality of the association model and potentially increase accuracy. Strength of association statistics (estimated effect, p-value) and population differentiation measurements (FST score) have both been explored as criteria for preselection, but sensitivity to identify relevant markers decreases as random noise exceeds true signal variation. Combining both criteria into an index would leverage the unique contributions of each criterion and potentially increase prediction accuracies. A simulation consisting of 200 QTL, 777k SNP, and 7 generations under selection was generated (10 replicates). Marker preselection was compared across three criteria: only estimated effect (EFF), only FST score (FST), or an index combining the two previous statistics (COMB). In the COMB scenario, markers from genomic regions with high correlation (>0.7) between estimated effect and FST score were selected along with markers whose estimated effect or FST score exceeded a certain threshold. Across replicates, COMB identified additional markers tagging between 1 and 7 QTL not tagged by EFF or FST that explain 0.2–5.4% of the genetic variance. The highest accuracy for EFF and FST was 0.76 and 0.73 when preselecting 2k and 10k markers, respectively. Under the best-case scenario (3,297 preselected markers), COMB improved accuracy by less than 1% and 4% compared to EFF and FST scenarios, respectively. Though an index combining multiple statistics may increase the number of QTL tagged by preselected markers and genetic variance explained relative to single-statistic preselection, this does not necessarily translate to a meaningful increase in accuracy. However, the results are dependent on the indexing method.
更多查看译文
关键词
marker preselection, accuracy
AI 理解论文
溯源树
样例
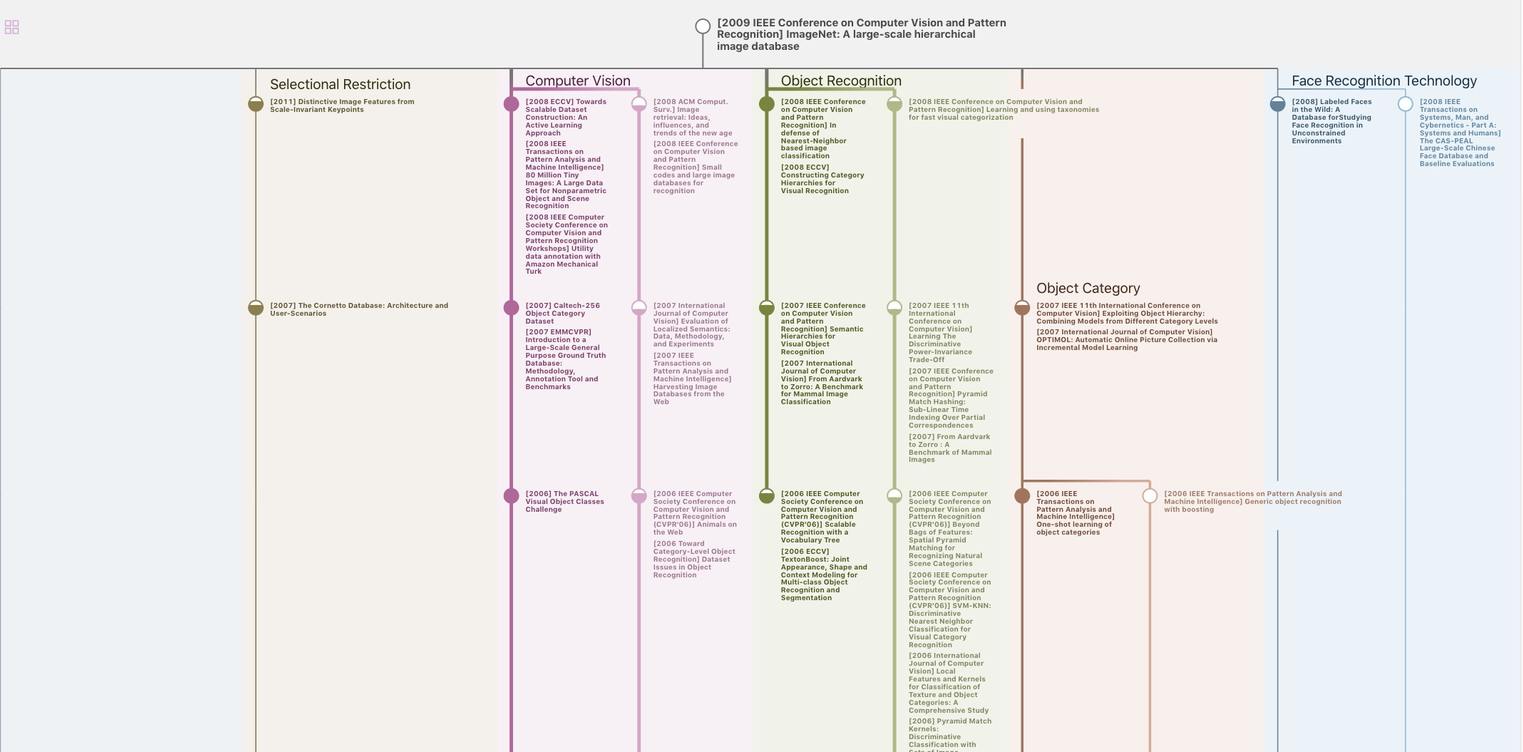
生成溯源树,研究论文发展脉络
Chat Paper
正在生成论文摘要