Local Explanations of Global Rankings: Insights for Competitive Rankings
IEEE ACCESS(2022)
摘要
Explaining complex algorithms and models has recently received growing attention in various domains to support informed decisions. Ranking functions are widely used for almost every form of human activity to enable effective decision-making processes. Hence, explaining ranking indicators and their importance are essential properties to enhance performance. Local explanation techniques have recently become a prominent way to interpret individual predictions of machine learning models. However, there has been limited investigation into explaining competitive rankings. This work proposes a hierarchical ranking explanation framework to capture local explanations for competitive rankings by defining a proper neighborhood construction approach. We explore various explanation techniques to identify the local contribution of ranking indicators based on the position of an instance in the ranking as well as the size of the neighborhood around the instance of interest. We evaluate the generated explanations for the Times Higher Education university ranking dataset as a benchmark of competitive ranking. The results reveal insights for a wide range of instances in the ranking list and indicate the importance of local explanations for competitive rankings.
更多查看译文
关键词
Education, Benchmark testing, Machine learning, Web pages, Task analysis, Principal component analysis, Predictive models, Competitive ranking, feature importance, local explanation
AI 理解论文
溯源树
样例
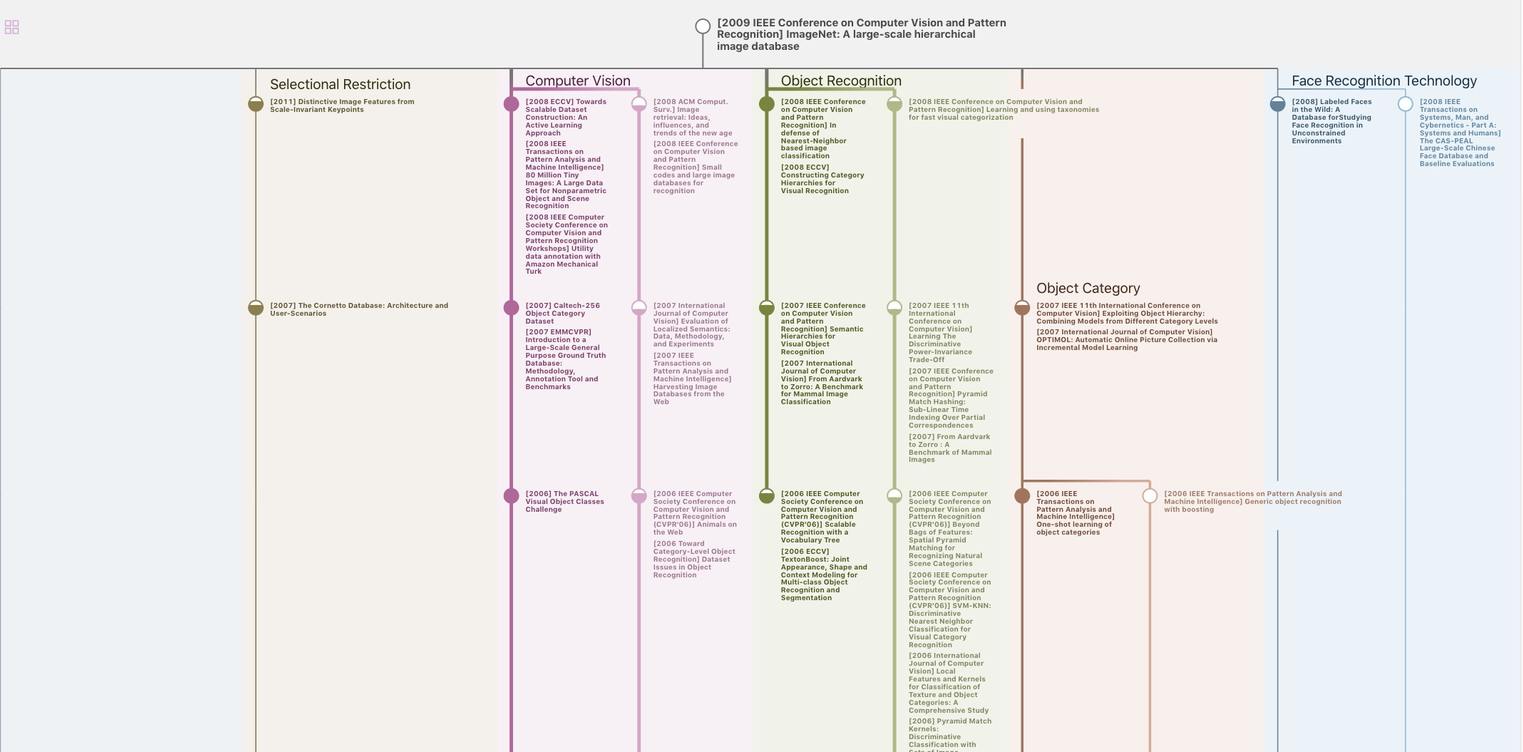
生成溯源树,研究论文发展脉络
Chat Paper
正在生成论文摘要