Mutated traffic detection and recovery: an adversarial generative deep learning approach
Annals of Telecommunications(2022)
摘要
Machine learning (ML)-based traffic classification is evolving into a well-established research domain. Considering statistical characteristics of the traffic flows, ML-based classification methods have succeeded in even classifying encrypted traffic. However, recent research efforts have emerged, for privacy preservation, where traffic obfuscation is being considered as a way to hide traffic characteristics preventing traffic classification. Traffic mutation is one such obfuscation technique that consists of modifying the flow packet sizes and inter-arrival times. However, at the same time, these techniques can be used by malicious attackers to hide their attack traffic and avoid detection. In this paper, we propose a deep learning (DL) model to detect mutated traffic and recover the original one. The experimental results show the effectiveness of the proposed model in detecting mutated traffic with a detection rate up to 95%, on average, and denoising recovery loss less than 3 × 10 − 1 .
更多查看译文
关键词
Machine learning, Network security, Traffic classification, Obfuscation, Deep learning, IoT, Autoencoder, Generative adversarial network
AI 理解论文
溯源树
样例
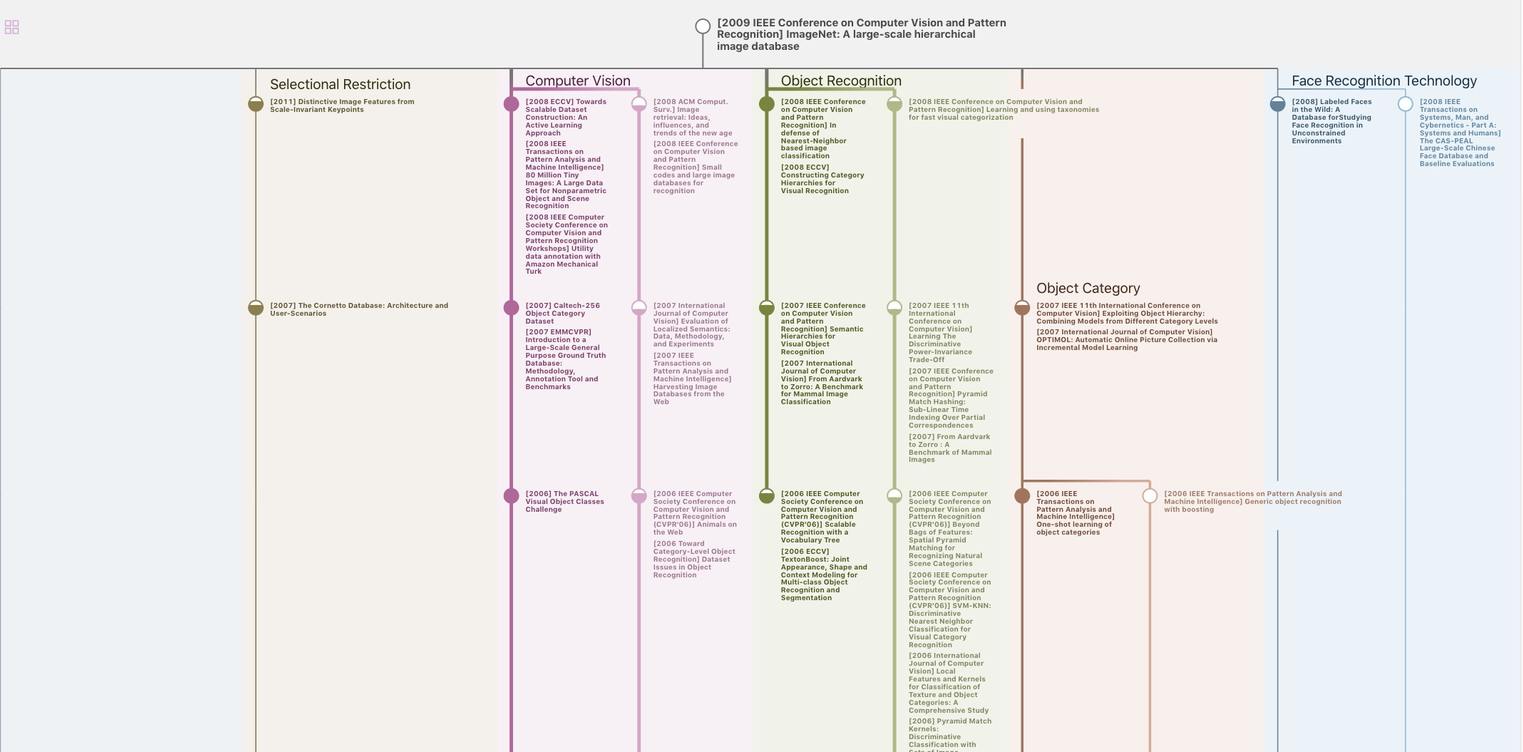
生成溯源树,研究论文发展脉络
Chat Paper
正在生成论文摘要