Comparison of different statistical downscaling models and future projection of areal mean precipitation of a river basin under climate change effect
WATER SUPPLY(2022)
摘要
Investigation of the hydrological impacts of climate change at the local scale requires the use of a statistical downscaling technique. In order to use the output of a Global Circulation Model (GCM) model, downscaling technique is used. In this study, statistical downscaling of monthly areal mean precipitation of Goksun River basin in Turkey was carried out using the Group Method of Data Handling (GMDH), Support Vector Machines (SVM) and Gene-expression Programming (GEP) techniques. Large-scale weather factors are used for a basin with monthly areal mean precipitation (P-M) record from 1971 to 2000 for training and testing periods. The R-2-value for precipitation in SVM, GEP and GMDH models are 0.62, 0.59, and 0.6 respectively, for testing periods. The results showed that SVM has the best model performance than the other proposed downscaling models, however, AIC values showed the GEP model has the lowest AIC value. The simulated results for CGCM3 A1B and A2 scenarios show a similarity in their average precipitation prediction. Generally, both scenarios anticipate a decrease in the average monthly precipitation during the simulated periods. Therefore, the results of future projections show that the mean precipitation might decrease during the period of 2021-2100.
更多查看译文
关键词
artificial intelligence, climate change, GCMs, precipitation, statistical downscaling
AI 理解论文
溯源树
样例
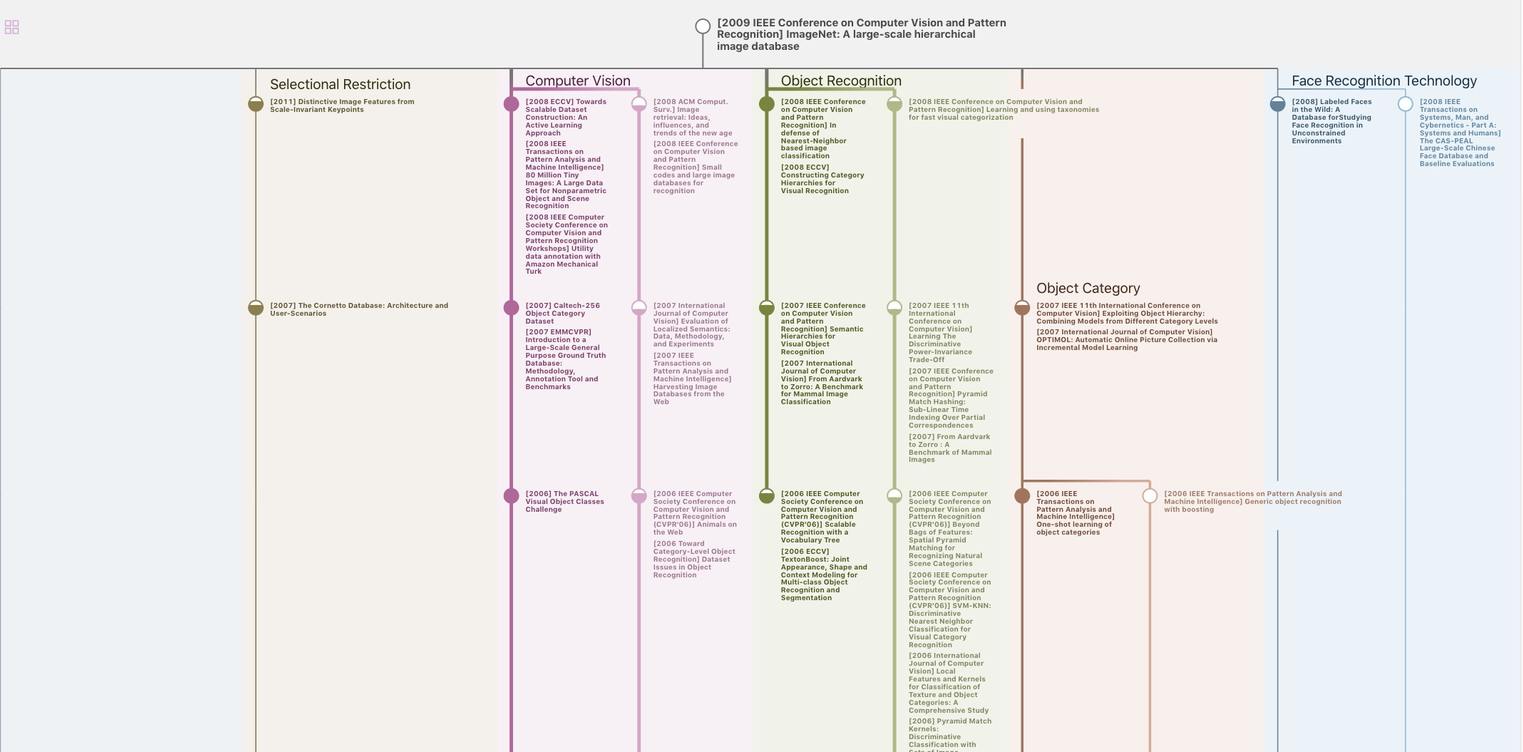
生成溯源树,研究论文发展脉络
Chat Paper
正在生成论文摘要