Predicting Shale Volume from Seismic Traces Using Modified Random Vector Functional Link Based on Transient Search Optimization Model: A Case Study from Netherlands North Sea
Natural Resources Research(2022)
摘要
Seismic data have the advantage of wide aerial distribution and deep extent unlike well data that are restricted to a borehole’s location, measuring intervals, and depth. In addition, seismic data are suitable for delineation of structural and stratigraphic features, whereas well logs are suitable for delineation of much smaller scale petrophysical properties. However, both methods are beneficial for extending small-scale petrophysical parameters to large-scale seismic volumes. In seismic data, seismic traces are mainly band-limited, whereas sources of seismic data do not offer the entire band of frequencies required for the desired resolution to be comparable to well data. Therefore, it is a big challenge to compare well data with a resolution that is several orders greater than that of seismic data. The integration of petrophysical parameters and seismic traces helps to predict the lateral distribution of petrophysical properties. However, the traditional prediction methods have their limitations. Therefore, this study used seismic and well logs to predict shale volume using the proposed model with an artificial neural network. The proposed hybrid model consists of a conventional random vector functional link (RVFL) network and the transient search optimization (TSO) algorithm, and the model is named TSO–RVFL. This model predicts shale volume in wells. The TSO–RVFL was compared with the standalone RVFL and other two hybrid models. The results of this study validated the successful performance of the artificial neural network for calculating and predicting petrophysical parameters, such as shale volume. The TSO–RVFL outperformed the three other models based on different statistical measures.
更多查看译文
关键词
Random vector functional link (RVFL), Transient search optimization (TSO), Seismic, Well logging, Petrophysics, Machine learning
AI 理解论文
溯源树
样例
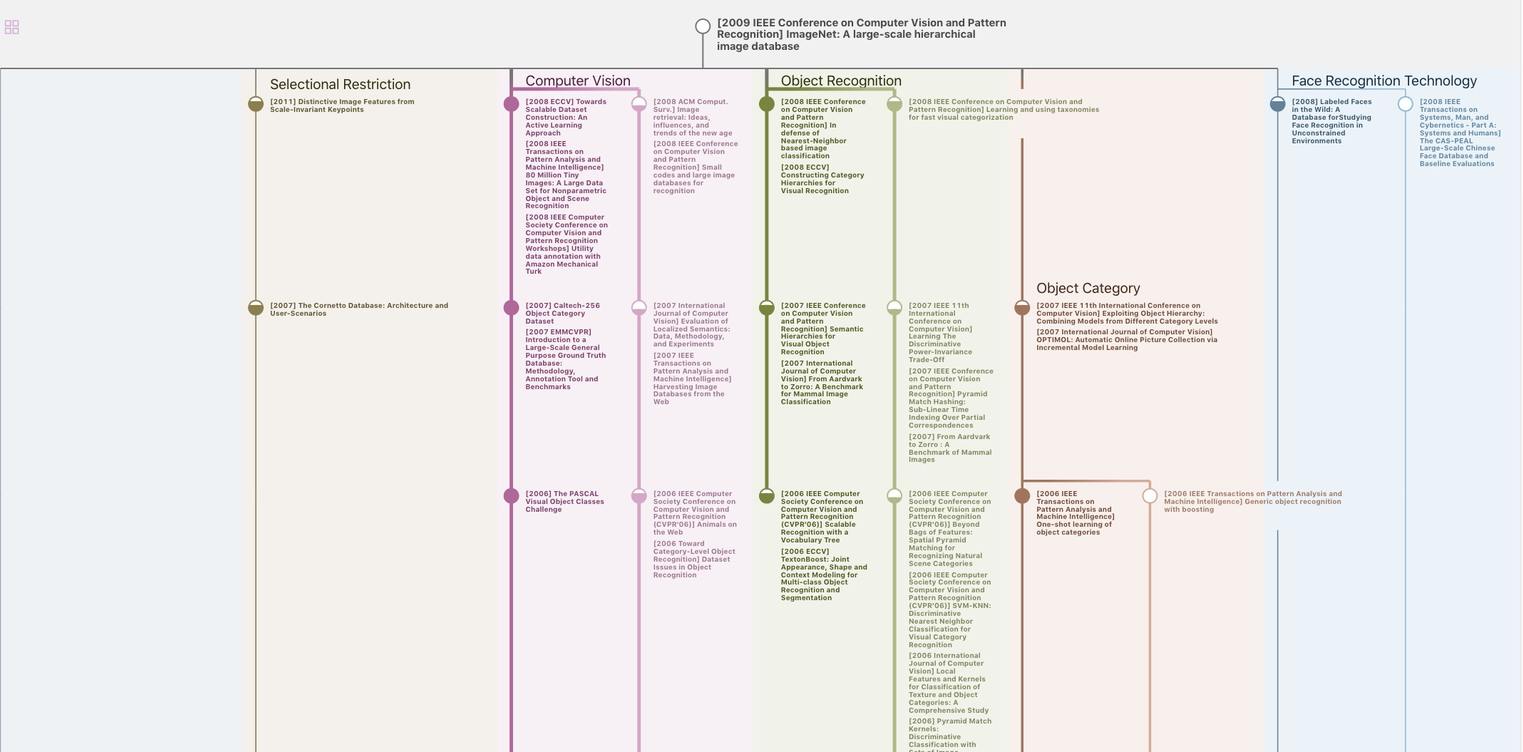
生成溯源树,研究论文发展脉络
Chat Paper
正在生成论文摘要