A Low-Cost Hardware-Friendly Spiking Neural Network Based on Binary MRAM Synapses, Accelerated Using In-Memory Computing
ELECTRONICS(2021)
摘要
In recent years, the scaling down that Moore's Law relies on has been gradually slowing down, and the traditional von Neumann architecture has been limiting the improvement of computing power. Thus, neuromorphic in-memory computing hardware has been proposed and is becoming a promising alternative. However, there is still a long way to make it possible, and one of the problems is to provide an efficient, reliable, and achievable neural network for hardware implementation. In this paper, we proposed a two-layer fully connected spiking neural network based on binary MRAM (Magneto-resistive Random Access Memory) synapses with low hardware cost. First, the network used an array of multiple binary MRAM cells to store multi-bit fixed-point weight values. This helps to simplify the read/write circuit. Second, we used different kinds of spike encoders that ensure the sparsity of input spikes, to reduce the complexity of peripheral circuits, such as sense amplifiers. Third, we designed a single-step learning rule, which fit well with the fixed-point binary weights. Fourth, we replaced the traditional exponential Leak-Integrate-Fire (LIF) neuron model to avoid the massive cost of exponential circuits. The simulation results showed that, compared to other similar works, our SNN with 1184 neurons and 313,600 synapses achieved an accuracy of up to 90.6% in the MNIST recognition task with full-resolution (28 x 28) and full-bit-depth (8-bit) images. In the case of low-resolution (16 x 16) and black-white (1-bit) images, the smaller version of our network with 384 neurons and 32,768 synapses still maintained an accuracy of about 77%, extending its application to ultra-low-cost situations. Both versions need less than 30,000 samples to reach convergence, which is a >50% reduction compared to other similar networks. As for robustness, it is immune to the fluctuation of MRAM cell resistance.
更多查看译文
关键词
spiking neural network (SNN), binary MRAM synapses, spike-rate neural coding, unsupervised learning, discretized spike-timing-dependent plasticity (STDP), leak-integrate-fire (LIF) model, in-memory computing, hardware acceleration
AI 理解论文
溯源树
样例
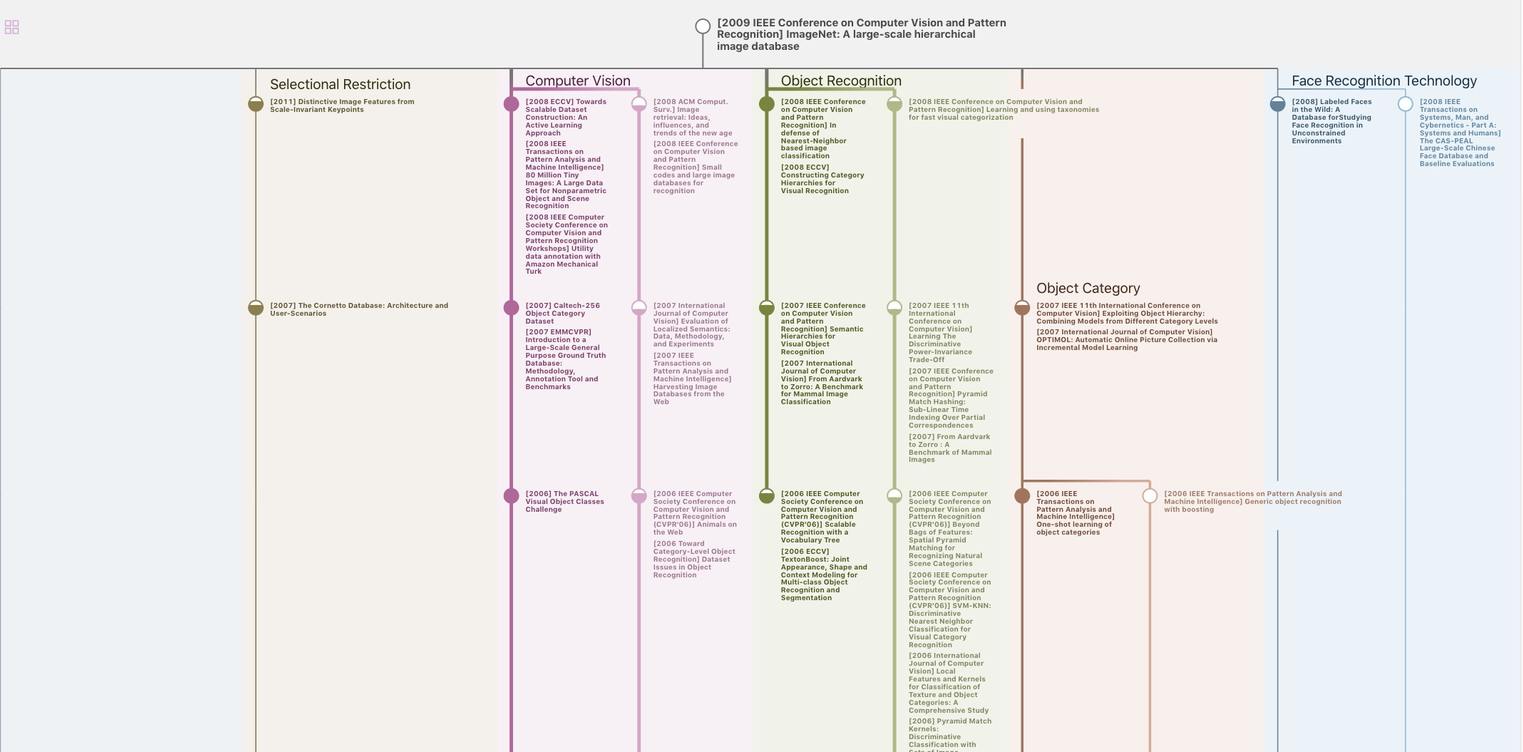
生成溯源树,研究论文发展脉络
Chat Paper
正在生成论文摘要