PIXAL: Anomaly Reasoning with Visual Analytics
CoRR(2022)
摘要
Anomaly detection remains an open challenge in many application areas. While there are a number of available machine learning algorithms for detecting anomalies, analysts are frequently asked to take additional steps in reasoning about the root cause of the anomalies and form actionable hypotheses that can be communicated to business stakeholders. Without the appropriate tools, this reasoning process is time-consuming, tedious, and potentially error-prone. In this paper we present PIXAL, a visual analytics system developed following an iterative design process with professional analysts responsible for anomaly detection. PIXAL is designed to fill gaps in existing tools commonly used by analysts to reason with and make sense of anomalies. PIXAL consists of three components: (1) an algorithm that finds patterns by aggregating multiple anomalous data points using first-order predicates, (2) a visualization tool that allows the analyst to build trust in the algorithmically-generated predicates by performing comparative and counterfactual analyses, and (3) a visualization tool that helps the analyst generate and validate hypotheses by exploring which features in the data most explain the anomalies. Finally, we present the results of a qualitative observational study with professional analysts. These results of the study indicate that PIXAL facilitates the anomaly reasoning process, allowing analysts to make sense of anomalies and generate hypotheses that are meaningful and actionable to business stakeholders.
更多查看译文
关键词
anomaly reasoning,visual analytics
AI 理解论文
溯源树
样例
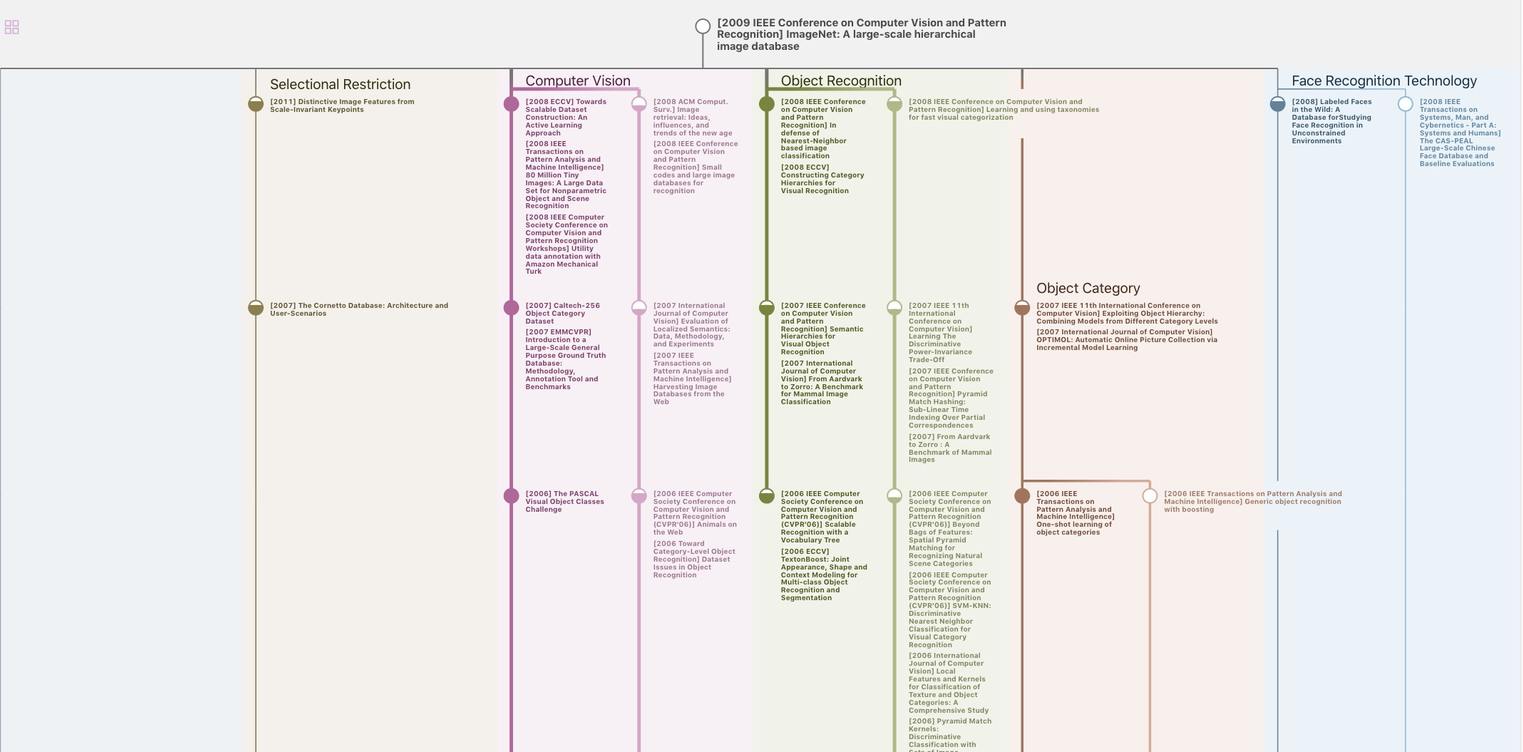
生成溯源树,研究论文发展脉络
Chat Paper
正在生成论文摘要