Commonsense Knowledge Salience Evaluation with a Benchmark Dataset in E-commerce
arxiv(2022)
摘要
In e-commerce, the salience of commonsense knowledge (CSK) is beneficial for widespread applications such as product search and recommendation. For example, when users search for ``running'' in e-commerce, they would like to find products highly related to running, such as ``running shoes'' rather than ``shoes''. Nevertheless, many existing CSK collections rank statements solely by confidence scores, and there is no information about which ones are salient from a human perspective. In this work, we define the task of supervised salience evaluation, where given a CSK triple, the model is required to learn whether the triple is salient or not. In addition to formulating the new task, we also release a new Benchmark dataset of Salience Evaluation in E-commerce (BSEE) and hope to promote related research on commonsense knowledge salience evaluation. We conduct experiments in the dataset with several representative baseline models. The experimental results show that salience evaluation is a challenging task where models perform poorly on our evaluation set. We further propose a simple but effective approach, PMI-tuning, which shows promise for solving this novel problem. Code is available in \url{https://github.com/OpenBGBenchmark/OpenBG-CSK.
更多查看译文
关键词
knowledge,benchmark dataset,evaluation,e-commerce
AI 理解论文
溯源树
样例
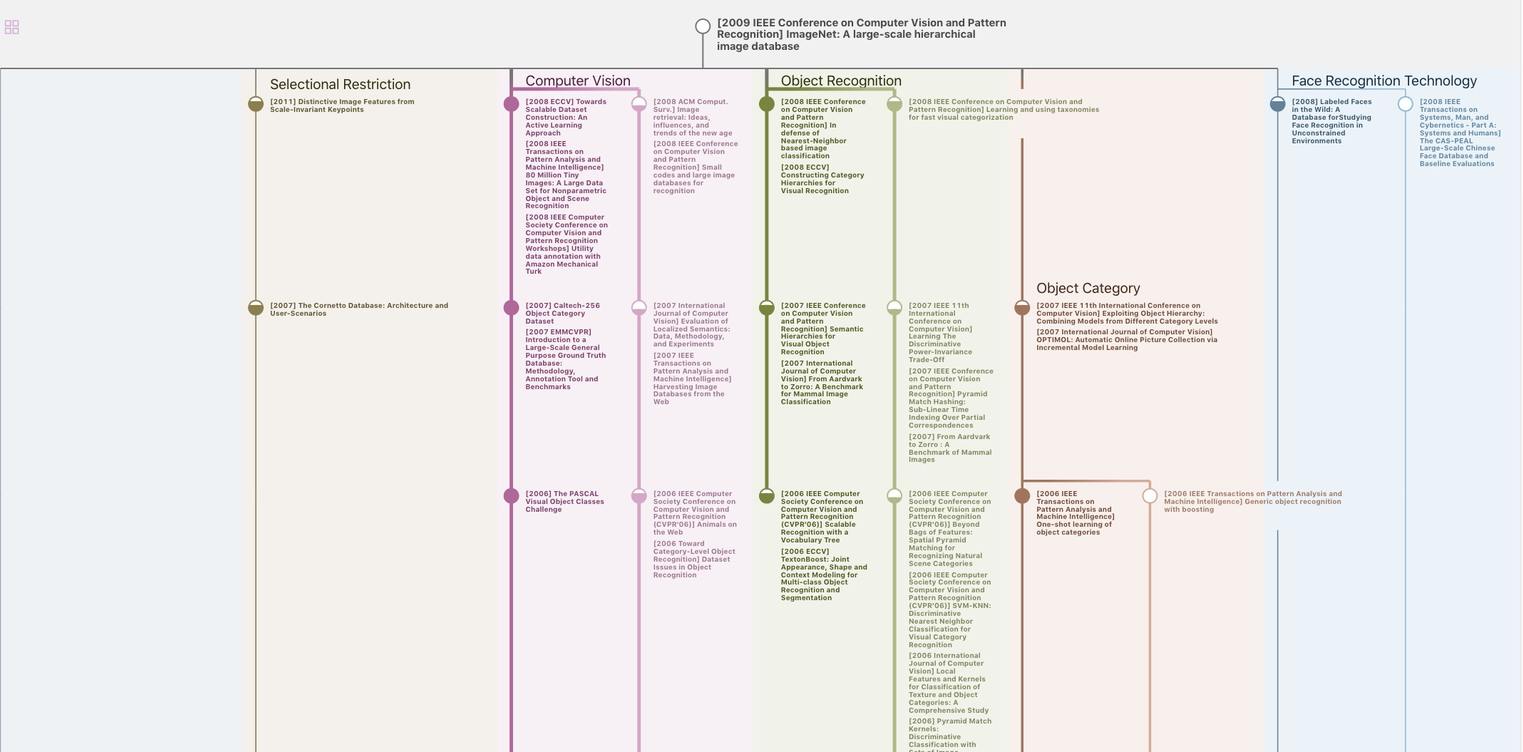
生成溯源树,研究论文发展脉络
Chat Paper
正在生成论文摘要