Complex behavior from intrinsic motivation to occupy action-state path space
arxiv(2022)
摘要
Most theories of behavior posit that agents tend to maximize some form of
reward or utility. However, animals very often move with curiosity and seem to
be motivated in a reward-free manner. Here we abandon the idea of reward
maximization, and propose that the goal of behavior is maximizing occupancy of
future paths of actions and states. According to this maximum occupancy
principle, rewards are the means to occupy path space, not the goal per se;
goal-directedness simply emerges as rational ways of searching for resources so
that movement, understood amply, never ends. We find that action-state path
entropy is the only measure consistent with additivity and other intuitive
properties of expected future action-state path occupancy. We provide
analytical expressions that relate the optimal policy and state-value function,
and prove convergence of our value iteration algorithm. Using discrete and
continuous state tasks, including a high–dimensional controller, we show that
complex behaviors such as `dancing', hide-and-seek and a basic form of
altruistic behavior naturally result from the intrinsic motivation to occupy
path space. All in all, we present a theory of behavior that generates both
variability and goal-directedness in the absence of reward maximization.
更多查看译文
AI 理解论文
溯源树
样例
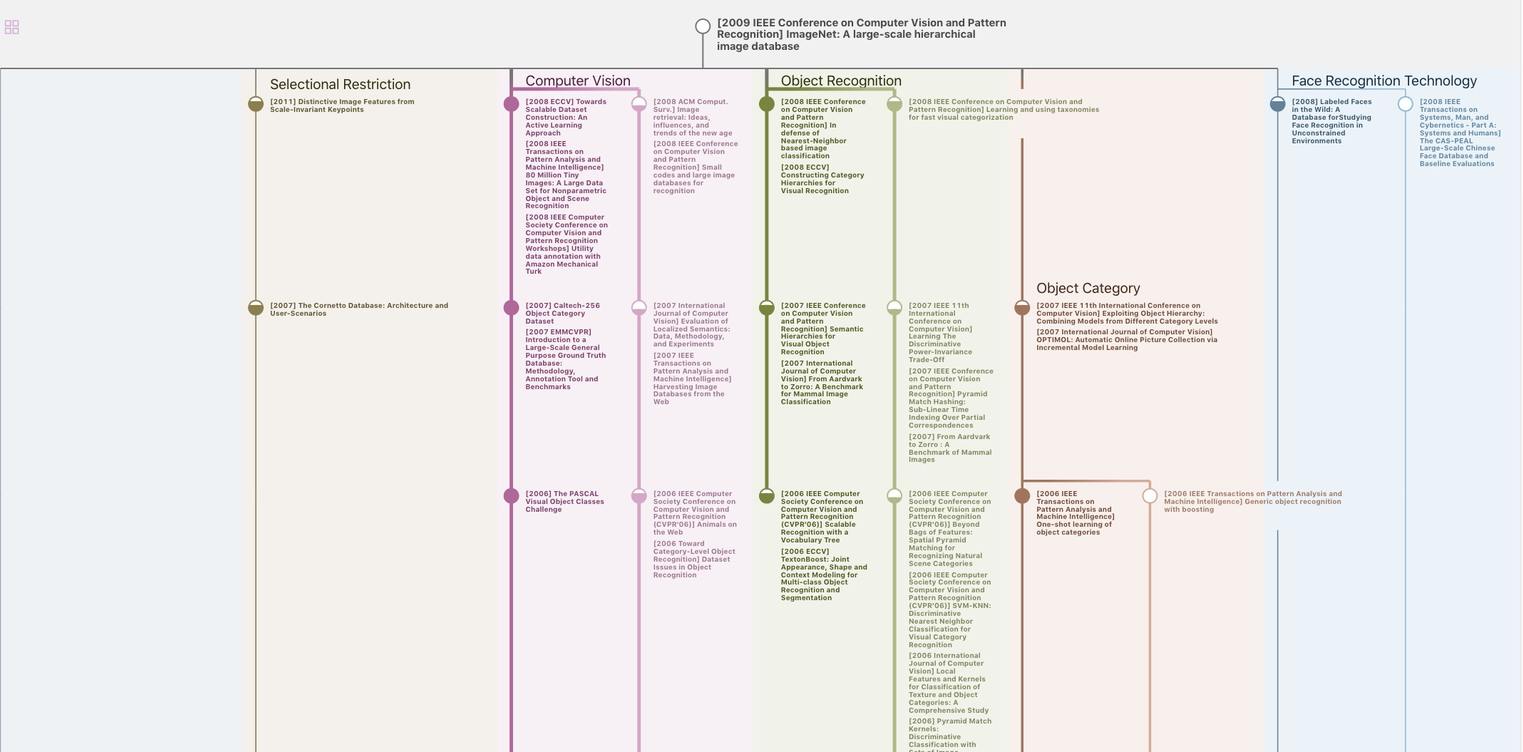
生成溯源树,研究论文发展脉络
Chat Paper
正在生成论文摘要