Paying Attention to Video Generation.
Annual Conference on Neural Information Processing Systems(2020)
摘要
Video generation is a challenging research topic which has been tackled by a variety of methods including Generative Adversarial Networks (GANs), Variational Autoencoders (VAE), optical flow and autoregressive models. However, most of the existing works model the task as image manipulation and learn pixel-level transforms. In contrast, we propose a latent vector manipulation approach using sequential models, particularly the Generative Pre-trained Transformer (GPT). Further, we propose a novel Attention-based Discretized Autoencoder (ADAE) which learns a finite-sized codebook that serves as a basis for latent space representations of frames, to be modelled by the sequential model. To tackle the reduced resolution or the diversity bottleneck caused by the finite codebook, we propose attention-based soft-alignment instead of a hard distance-based choice for sampling the latent vectors. We extensively evaluate the proposed approach on the BAIR Robot Pushing, Sky Time-lapse and Dinosaur Game datasets and compare with state-of-the-art (SOTA) approaches. Upon experimentation, we find that our model suffers mode collapse owing to a single vector latent space learned by the ADAE. The cause for this mode collapse is traced back to the peaky attention scores resulting from the codebook (Keys and Values) and the encoder’s output (Query). Through our findings, we highlight the importance of reliable latent space frame representations for successful sequential modelling.
更多查看译文
AI 理解论文
溯源树
样例
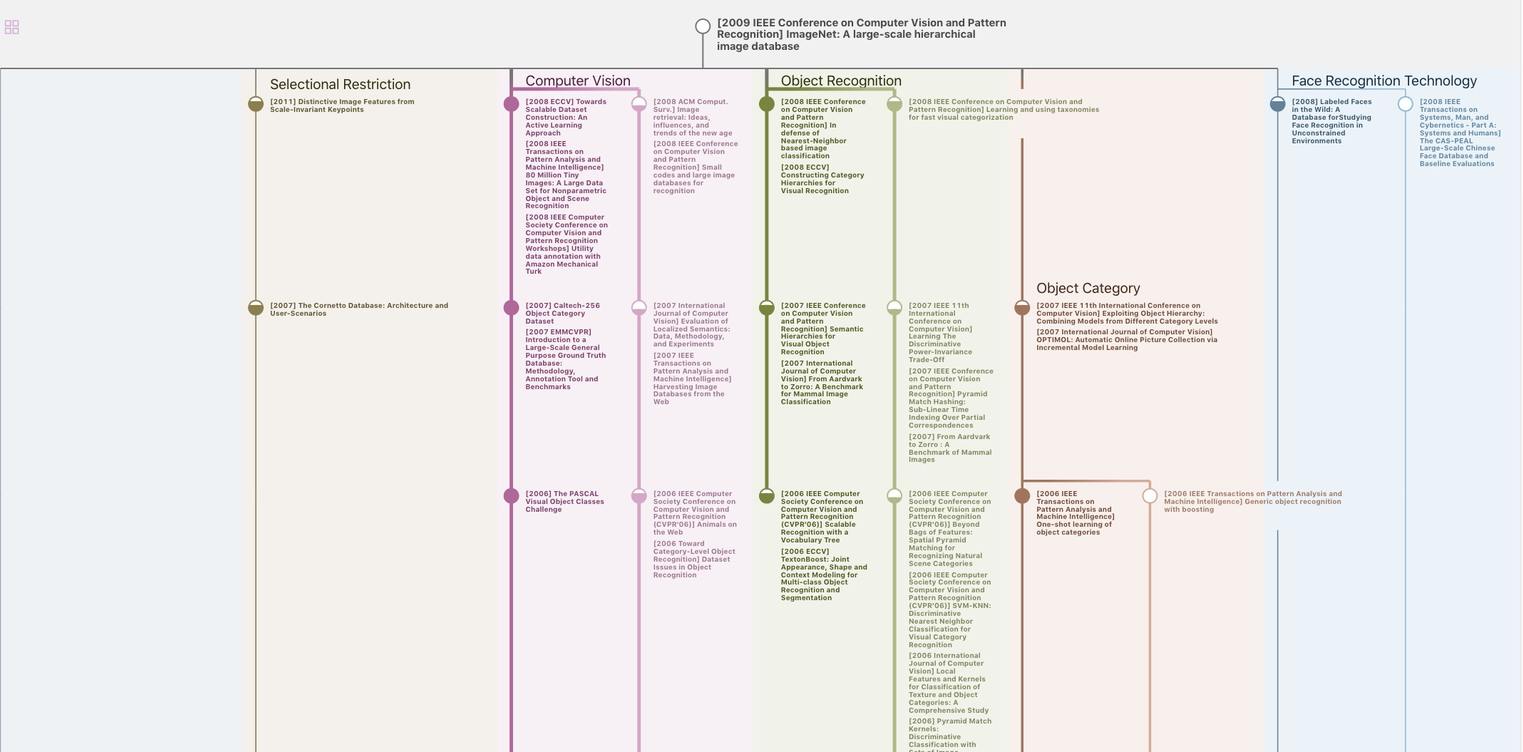
生成溯源树,研究论文发展脉络
Chat Paper
正在生成论文摘要