Unsupervised clustering of autonomic temporal networks in clinically distressed and psychologically healthy individuals
Behaviour Research and Therapy(2022)
摘要
The present study recruited psychologically healthy individuals and individuals with clinically-severe Diagnostic and Statistical Manual of Mental Disorders, Fifth Edition diagnoses, including generalized anxiety disorder, major depressive disorder, social anxiety disorder, posttraumatic stress disorder, panic disorder, persistent depressive disorder, and specific phobia. During the course of a structured clinical interview, 200 individuals provided continuous electrocardiogram and impedance cardiography data. Of these N = 150 were used for exploratory analyses and N = 50 for confirmatory analyses. From these time series, we modeled heart period (i.e. interbeat interval), pre-ejection period, respiratory sinus arrhythmia, and respiration rate. The group iterative multiple model estimation (GIMME) model was used to generate group and individual-level network models which, in turn, were used to conduct unsupervised classification of individual-level models into subgroups. Four subgroups were identified, comprising N = 22, N = 25, N = 26, and N = 61 individuals, with an additional 16 individuals left unclassified. The subgroup models were then used to estimate directed network models, from which out-degree and in-degree centrality were estimated for each group. Two groups, Group 2 and Group 4 exhibited elevated symptoms of depression and anxiety relative to the remaining sample. However, only one of these, Group 2, exhibited additional physiological risk features, including a significantly elevated average heart rate, and significantly reduced parasympathetic regulation (measured via respiratory sinus arrhythmia). We discuss the implications for utilizing network models for conducting systems-level analyses of physiological systems in clinically-distressed and psychologically healthy individuals.
更多查看译文
AI 理解论文
溯源树
样例
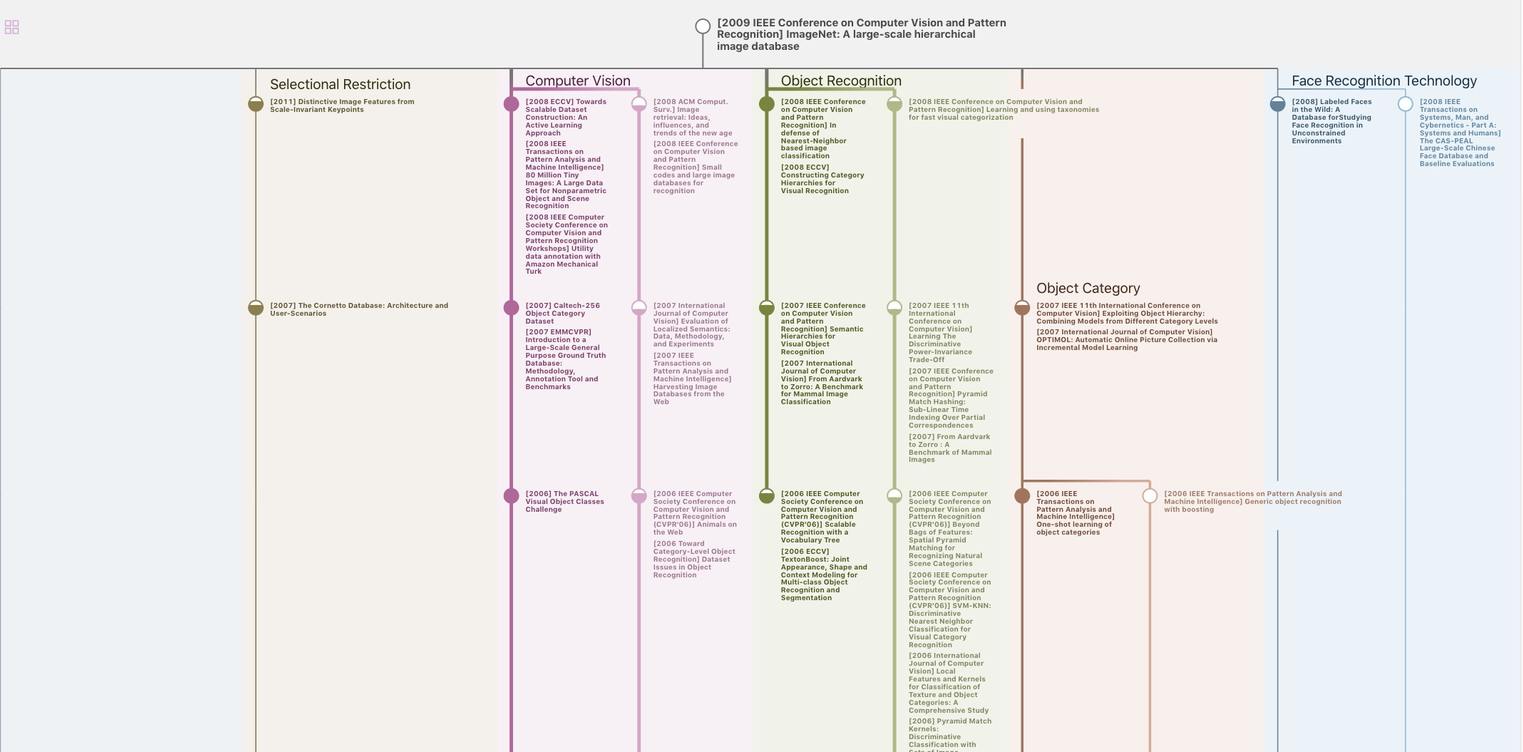
生成溯源树,研究论文发展脉络
Chat Paper
正在生成论文摘要