Sharp asymptotics on the compression of two-layer neural networks
2022 IEEE Information Theory Workshop (ITW)(2022)
摘要
In this paper, we study the compression of a target two-layer neural network with N nodes into a compressed network with M 2
loss between the outputs of the target and of the compressed network, under the assumption of Gaussian inputs. By using tools from high-dimensional probability, we show that this non-convex problem can be simplified when the target network is sufficiently over-parameterized, and provide the error rate of this approximation as a function of the input dimension and N. In this mean-field limit, the simplified objective, as well as the optimal weights of the compressed network, does not depend on the realization of the target network, but only on expected scaling factors. Furthermore, for networks with ReLU activation, we conjecture that the optimum of the simplified optimization problem is achieved by taking weights on the Equiangular Tight Frame (ETF), while the scaling of the weights and the orientation of the ETF depend on the parameters of the target network. Numerical evidence is provided to support this conjecture.
更多查看译文
关键词
compression,sharp asymptotics,neural networks,two-layer
AI 理解论文
溯源树
样例
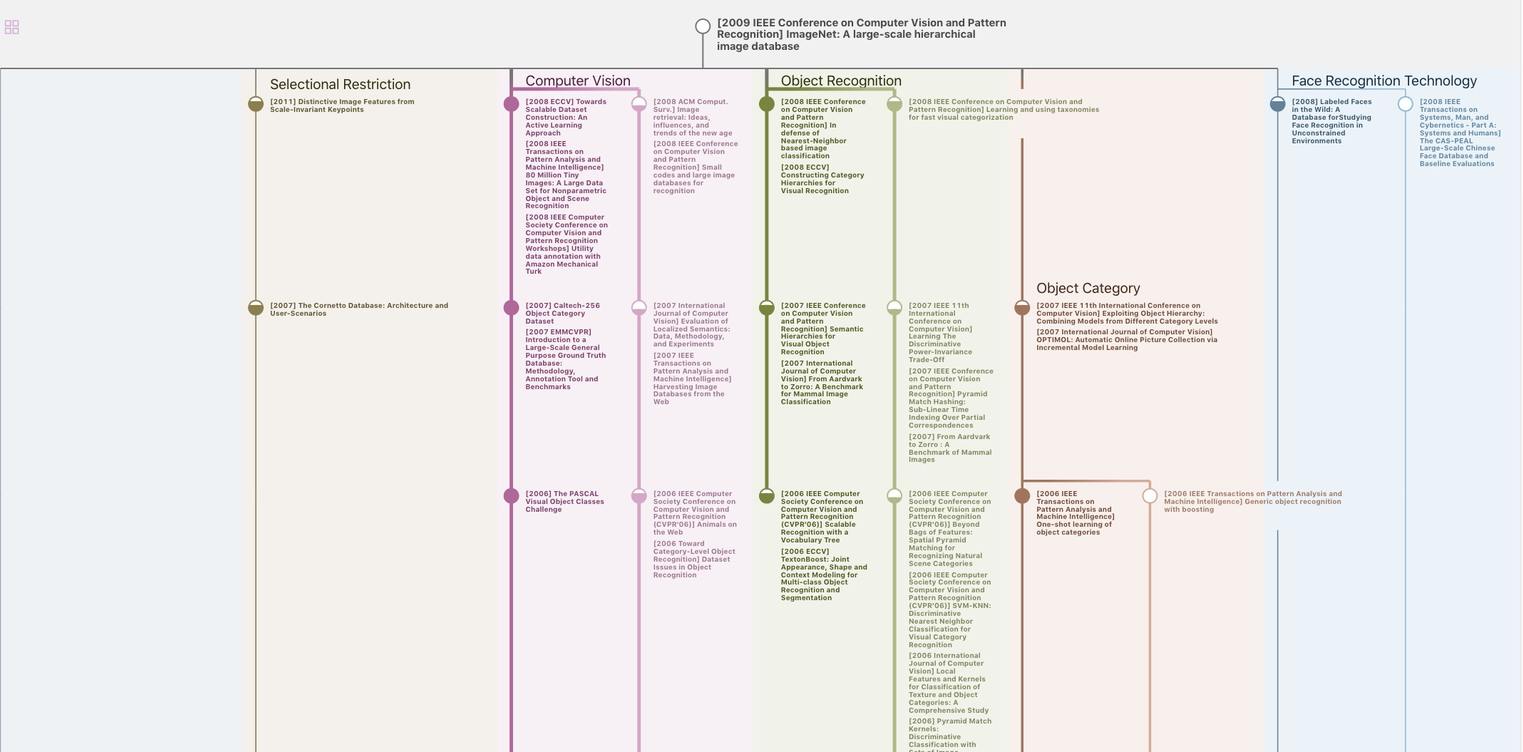
生成溯源树,研究论文发展脉络
Chat Paper
正在生成论文摘要