Autonomous Identification of Suitable Geotechnical Measurement Locations using Underwater Vehicles
OCEANS 2021: San Diego – Porto(2021)
摘要
Determining the geotechnical seafloor properties needed to plan subsea infrastructure is time consuming and expensive as it requires soil sampling or in-situ contact measurements to be made using Remotely Operated Vehicles or ship based systems. To increase the efficiency of such surveys, we introduce a predictive framework for autonomous underwater vehicles (AUV) to determine locations where they can land and make contact measurements. We introduce a geotechnical measurability index that is computed using high, cm-resolution AUV observations. To address the small footprint of high-resolution AUV observations, our method infers the distribution of measurability onto more widely available remote sensed bathymetry that has resolutions of tens of centimeters to metres. Features are extracted from these low-resolution priors using an unsupervised Location-Guided Autoencoder. Geotechnical measurability maps are generated using a Bayesian Neural Network that combines these features with the geotechnical measurability calculated from high-resolution AUV observations to infer the measurability over a wide area. The framework is demonstrated using AUV structured light mapping data that was obtained from a $420 imes 120m$ region of the Takuyo Daigo seamount. The data was artificially down sampled to simulate low resolution priors with sub-regions observed at high-resolution. The geotechnical measurability maps generated using the predictive framework preserve details that would otherwise be lost if the measurability index was calculated directly based on low resolution priors.
更多查看译文
关键词
AUV, autonomous landing, seafloor, Bayesian inference, autoencoders, geotechnical measurements
AI 理解论文
溯源树
样例
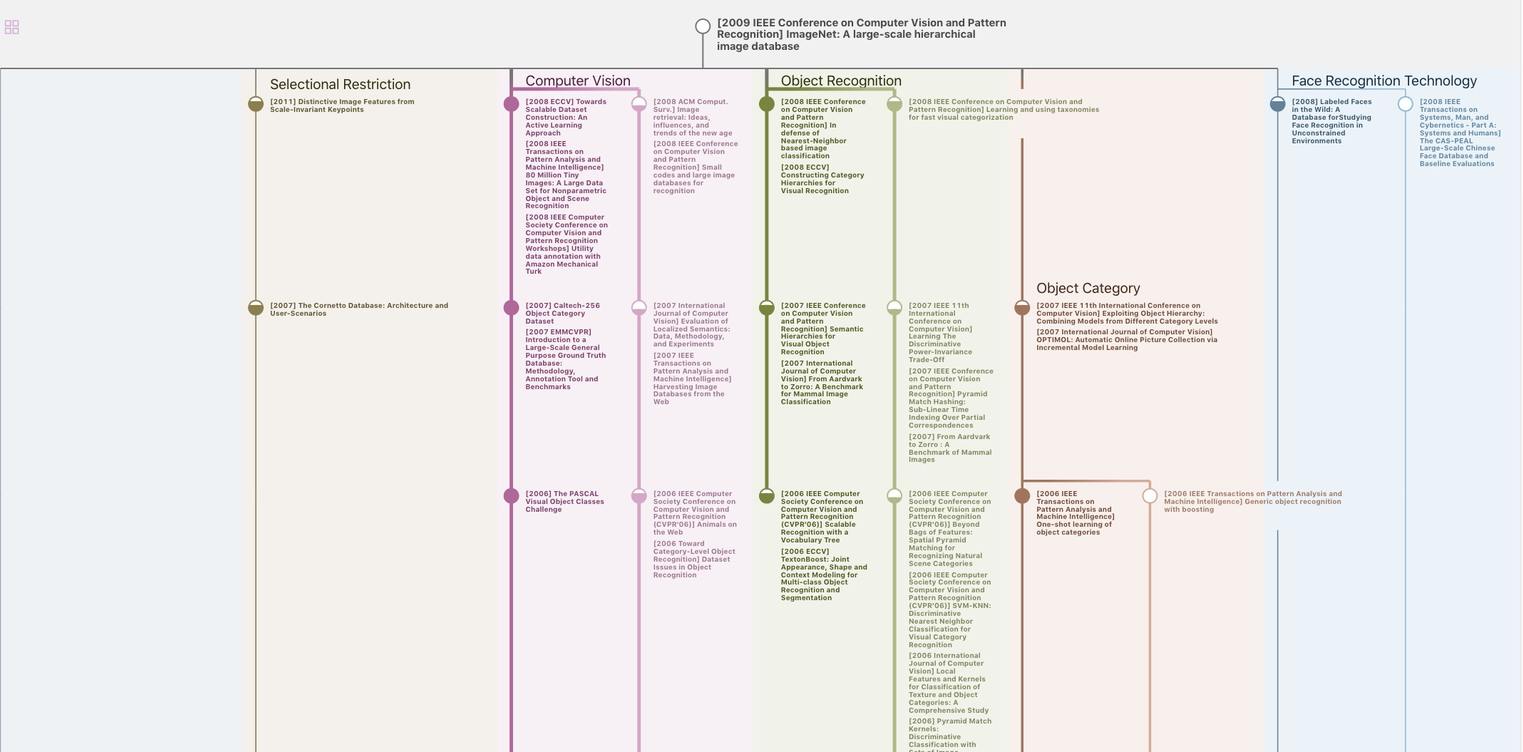
生成溯源树,研究论文发展脉络
Chat Paper
正在生成论文摘要