Real-Time FEA-based Breast Deformation Simulation using Artificial Neural Network
Computer methods and programs in biomedicine update(2022)
摘要
• A comprehensive workflow is presented that takes a patients diagnostic image as input, preprocesses it with finite element and neural network model, and then provides a breast nodal displacement prediction as output • A parametric study was performed to learn how breast structure and patients posture affect breast deformation • Neural network was constructed to map the direction of gravity and the breast structure to the breast surface nodal displacements and tumor location • Different visualization techniques were implemented and statistical data were collected to show the quality of the neural network model. • This technique can be utilized to provide accurate tumor location during a surgical procedure Background and objectives: Treatment of breast cancer involves two stages: diagnosis and treatment. It is difficult to correlate the imaging results at the two stages because as the patient’s position changes during treatment, the images captured during diagnosis do not represent the tumor location during the treatment. In the absence of real-time imaging during treatment, the visualization of tumor location is challenging for surgeons. In this paper, we combined finite element analysis (FEA) and neural network (NN) to accurately simulation real-time breast deformation for different patient’s positions. Methods: This research focuses on investigating visualization of breast deformation for different patients positions. We collected magnetic resonance imaging (MRI) images of a patient during diagnosis. This data was preprocessed to form a 3D reconstructed model that was used to run a FEA simulation. FEA simulates the deformation of breast tissues for different constraints, such as glandular ratio and gravity angle. However, it typically takes FEA to simulation such deformation from as fast as a few minutes to around 40 minutes when 8 cores were used. To obtain real-time visualization, we constructed a NN model that takes a breast gravity angle and glandular / fat ratio (breast material) as input to estimate breast deformation for different positions offline. The NN predicts the deformation and helps to visualize breast deformation in real-time (5 ms prediction time). Results: Our results show that NN has the ability to map the gravity direction and breast material structure to the breast shape and tumor location accurately, while, keeping run time to a minimum. The average nodal displacement validation error of breast surface was 0.013 mm, and the tumor center location error was 0.05 mm. Conclusions: We took a patient’s diagnostic images as input, and used FEA to simulate breast deformation. Then we implemented two NNs, one to take gravity direction and material composition as input to predict breast surface nodal displacement; the other one used the same input, but output tumor center position. Finally, the model was used to provide a real-time breast deformation prediction during treatment (5ms). Our approach can be used to create tools that can be used inside an operating room for accurate tumor prediction.
更多查看译文
关键词
Finite element,Neural network,Magnetic resonance imaging
AI 理解论文
溯源树
样例
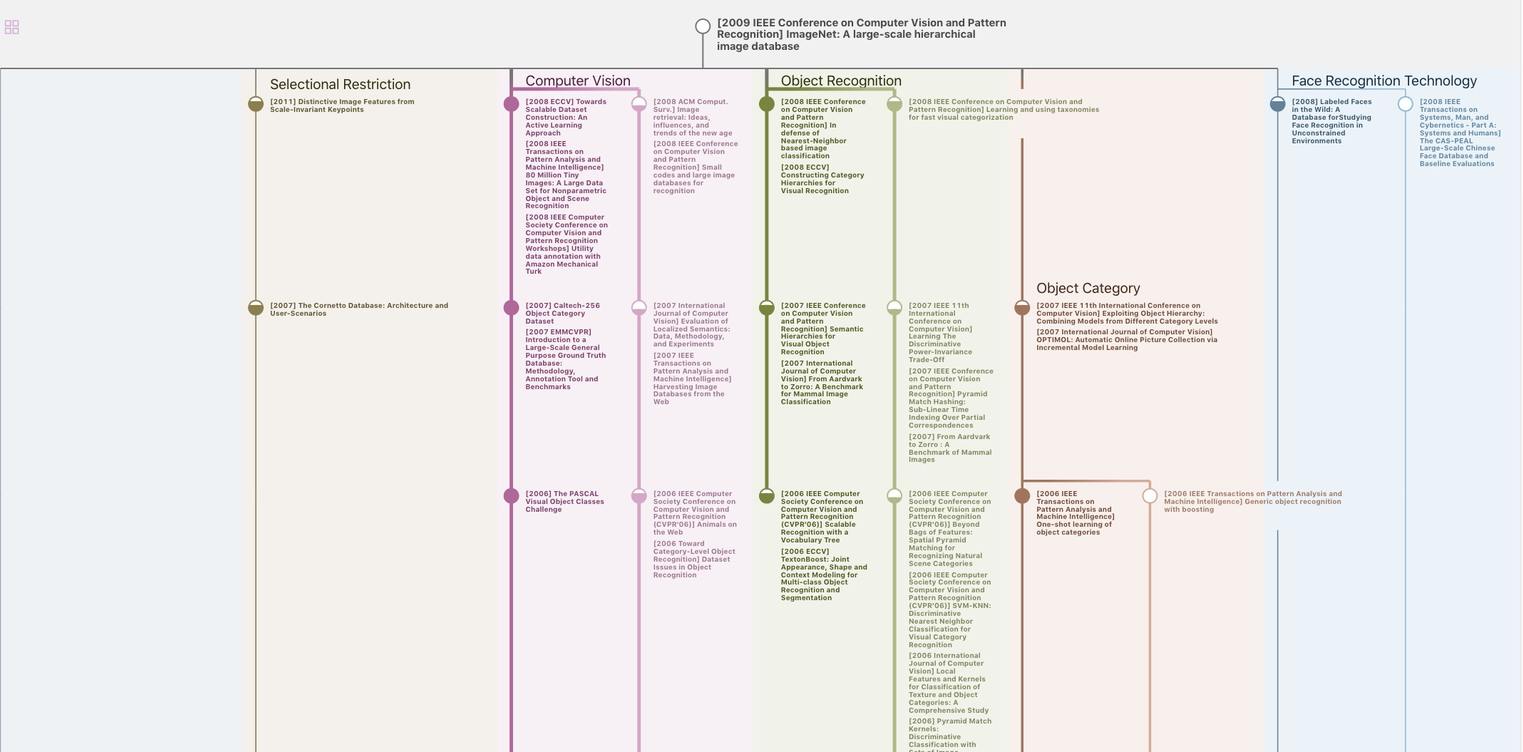
生成溯源树,研究论文发展脉络
Chat Paper
正在生成论文摘要