Robust AUV Visual Loop Closure Detection Based on Variational Auto-Encoder Network
IEEE Transactions on Industrial Informatics(2022)
摘要
The visual loop closure detection for Autonomous Underwater Vehicles (AUVs) is a key component to reduce the drift error accumulated in simultaneous localization and mapping tasks. However, due to viewpoint changes, textureless images, and fast-moving objects, the loop closure detection in dramatically changing underwater environments remains a challenging problem to traditional geometric methods. Inspired by strong feature learning ability of deep neural networks, we propose an underwater loop closure detection method based on a variational auto-encoder network in this paper. Our proposed method can learn effective image representations to overcome the challenges caused by dynamic underwater environments. Specifically, the proposed network is an unsupervised method, which avoids the difficulty and cost of labeling a great quantity of underwater data. Also included is a semantic object segmentation module, which is utilized to segment the underwater environments and assign weights to objects in order to alleviate the impact of fast-moving objects. Furthermore, an underwater image description scheme is used to enable efficient access to geometric and object-level semantic information, which helps to build a robust and real-time system in dramatically changing underwater scenarios. Finally, we test the proposed system under complex underwater environments and get a recall rate of 92.31% in the tested environments.
更多查看译文
关键词
Autonomous underwater vehicle (AUV) simultaneous localization and mapping (SLAM),deep neural network,loop closure detection,semantic segmentation
AI 理解论文
溯源树
样例
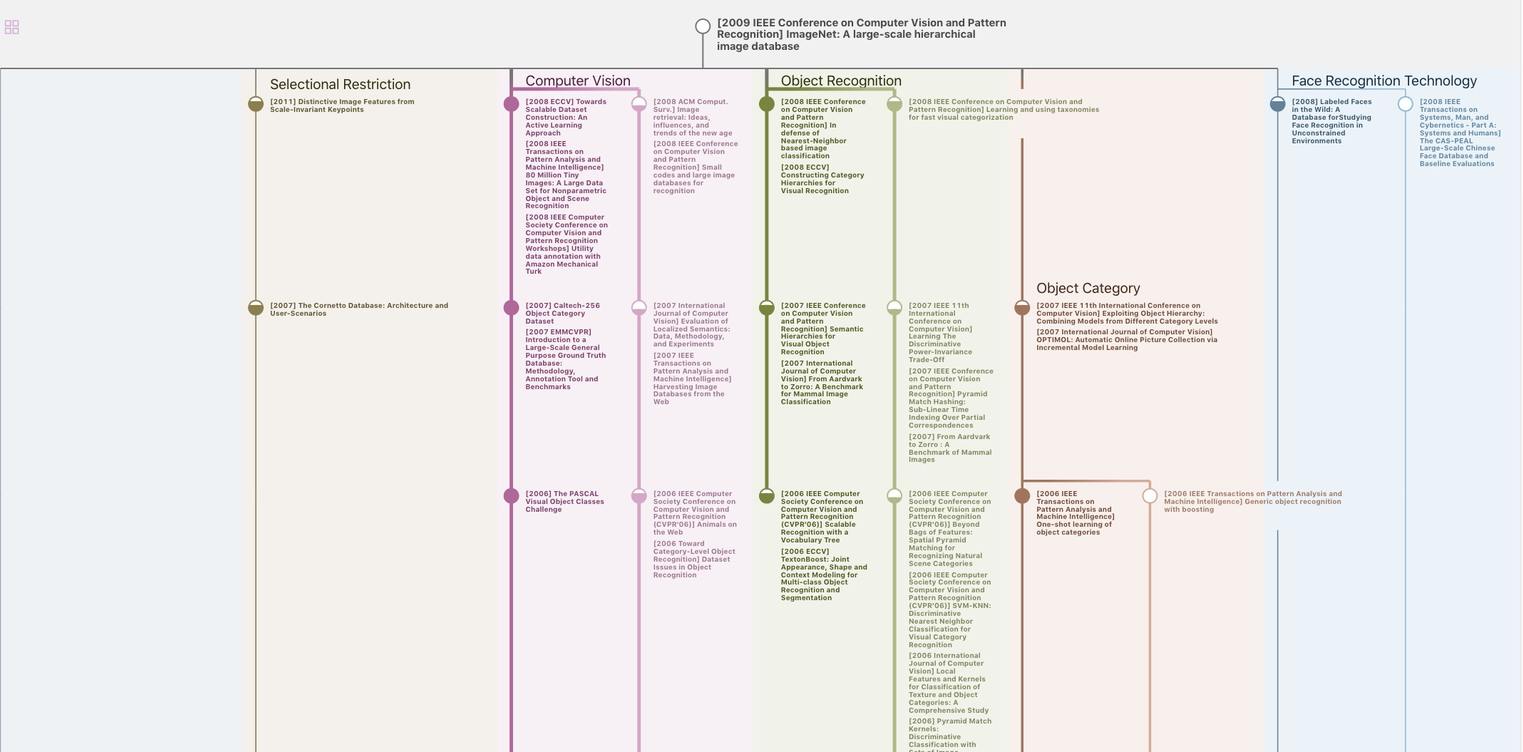
生成溯源树,研究论文发展脉络
Chat Paper
正在生成论文摘要