SAR Target Incremental Recognition Based on Hybrid Loss Function and Class-Bias Correction
Applied sciences(2022)
摘要
The Synthetic Aperture Radar (SAR) target recognition model usually needs to be retrained with all the samples when there are new-coming samples of new targets. Incremental learning emerges to continuously obtain new knowledge from new data while preserving most previously learned knowledge, saving both time and storage. There are still three problems in the existing incremental learning methods: (1) the recognition performance of old target classes degrades significantly during the incremental process; (2) the target classes are easily confused when similar target classes increase; (3) the model is inclined to new target classes due to class imbalance. Regarding the three problems, firstly, the old sample preservation and knowledge distillation were introduced to preserve both old representative knowledge and knowledge structure. Secondly, a class separation loss function was designed to reduce the intra-class distance and increase the inter-class distance, effectively avoiding the confusion between old and new classes. Thirdly, a bias correction layer and a linear model was designed, which enabled the model to treat the old and new target classes more fairly and eliminate the bias. The experimental results on the MSTAR dataset verified the superior performance compared with the other incremental learning methods.
更多查看译文
关键词
incremental learning,SAR target recognition,knowledge distillation,old sample preservation,class imbalance
AI 理解论文
溯源树
样例
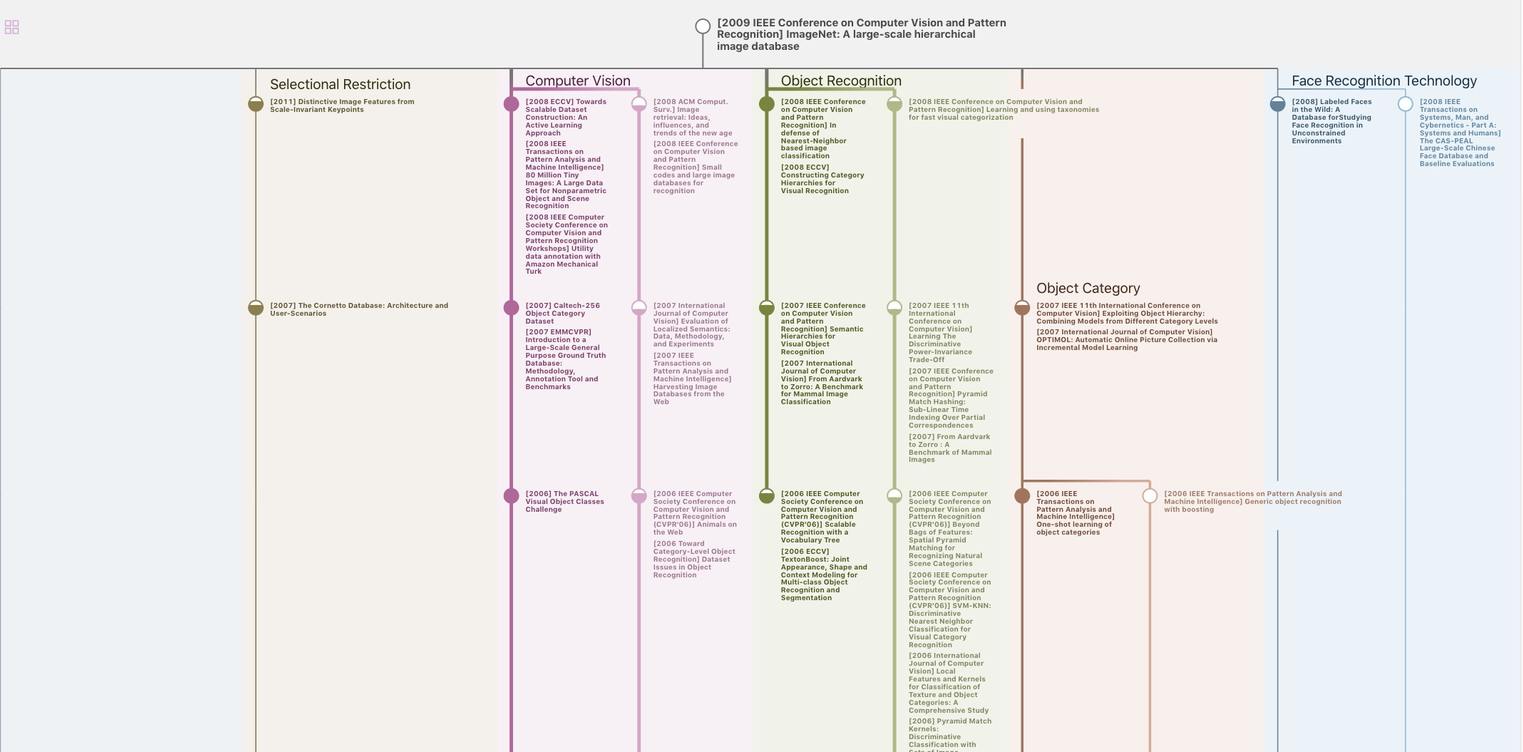
生成溯源树,研究论文发展脉络
Chat Paper
正在生成论文摘要