GCN recommendation model based on the fusion of dynamic multiple-view latent interest topics
Social Science Research Network(2022)
摘要
Graph neural network-based representation models have shown extraordinary potential in numerous recommender system applications. Previous studies mainly considered high-order connectivity information in a single view from an interaction graph but ignored the individualized information of the users or items, making them vulnerable to over-smoothing problems. In this study, we proposed a dynamic multi-view fusion-based graph convolution network model for recommendation systems. Multiple views were generated for learning on the basis of latent user interest topics from the decomposed matrix, and a continuous awareness mechanism was proposed to maintain the model’s focus on the individualized features of the nodes. During the graph learning process, a dynamic aggregation mechanism was designed to adjust the fusion weight of different propagation layers. Lastly, the different features from multiple views were dynamically fused through an attention mechanism and a principal component control mechanism to predict the similarities between users and items. Experimental results of three popular datasets of recommendation systems demonstrated that our method could effectively alleviate the over-smoothing problem and achieved better performance than four state-of-the-art baselines.
更多查看译文
关键词
Multiple-view fusion,Latent interest topic,Recommendation system,Graph convolution network
AI 理解论文
溯源树
样例
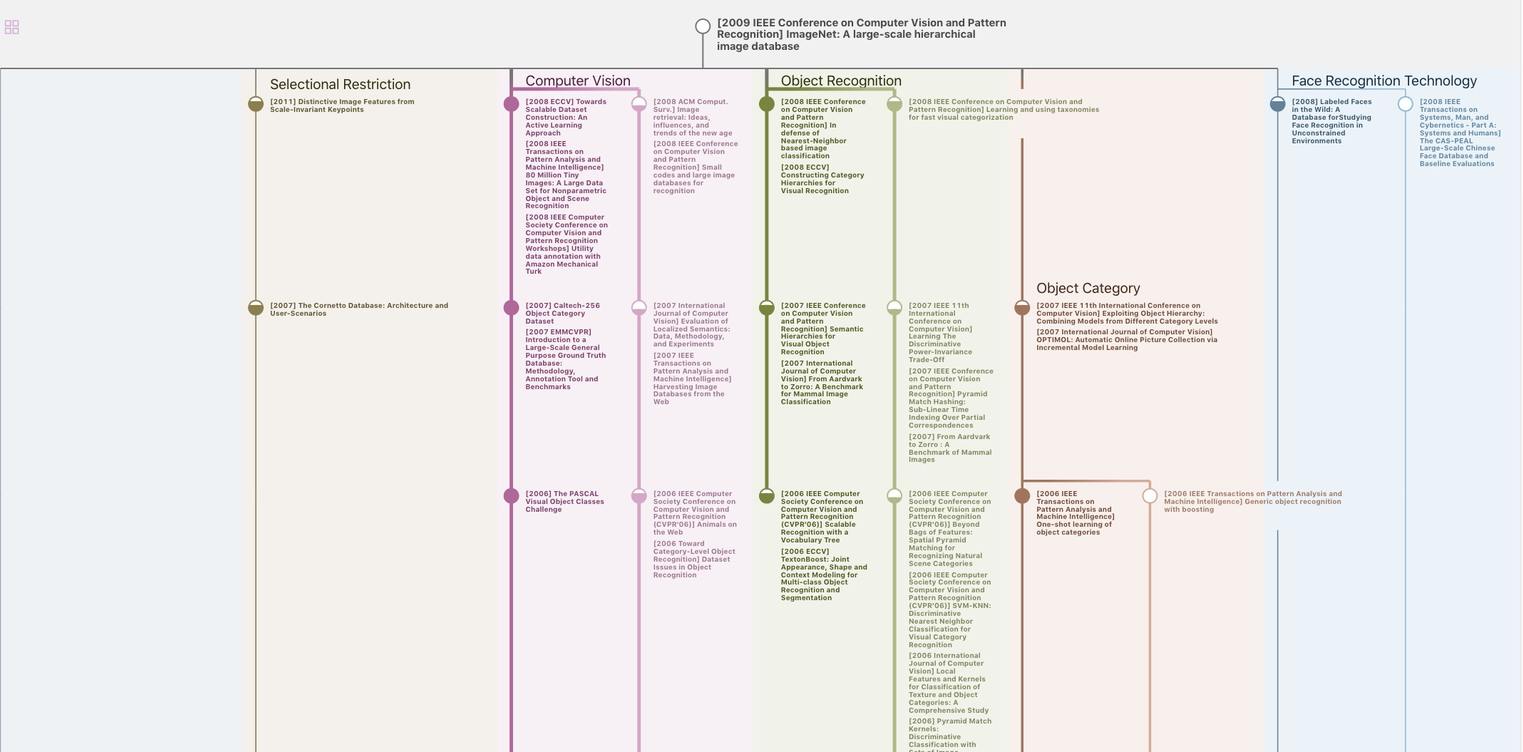
生成溯源树,研究论文发展脉络
Chat Paper
正在生成论文摘要