Intelligent Optimization of Reactive Voltage for Power Grid With New Energy Based on Deep Reinforcement Learning
2021 IEEE 5th Conference on Energy Internet and Energy System Integration (EI2)(2021)
摘要
Energy revolution is the guideline for the construction of modern intelligent power systems. All types of increasing new energy, as well as the requirement of fast demand responses will bring frequent and sizable voltage fluctuations. But existing voltage optimization method lacks accurate grid model and real-time computing capability, and can only make decisions offline. In response to the above problems, this paper proposes an intelligent optimization method for reactive voltage control based on deep reinforcement learning by transforming the voltage optimization problem of the power system into a Markov decision process. The optimization goal is to minimize the voltage deviation of each node, the system network loss, and maximize the reactive power reserve of each equipment. During offline learning, the agent continuously interacts with the power system environment built by the simulation platform to complete the network training. This method for voltage regulation of discrete reactive power compensation devices based on the Deep Q Network (DQN) algorithm does not rely on predictive data, and can achieve online optimization, effectively solving the multi-objective complex combination optimization of discrete reactive power compensation devices problems, improving the ability of the grid to respond to disturbances by massively connected new energy. Finally, through the IEEE test, in the case of new energy, load fluctuations and N-1 fault, voltage of each node, network loss and the reactive reserve of each device can be controlled rapidly, which verifies the validity of the above algorithm.
更多查看译文
关键词
voltage optimization,reactive compensation,deep reinforcement learning,Markov decision process
AI 理解论文
溯源树
样例
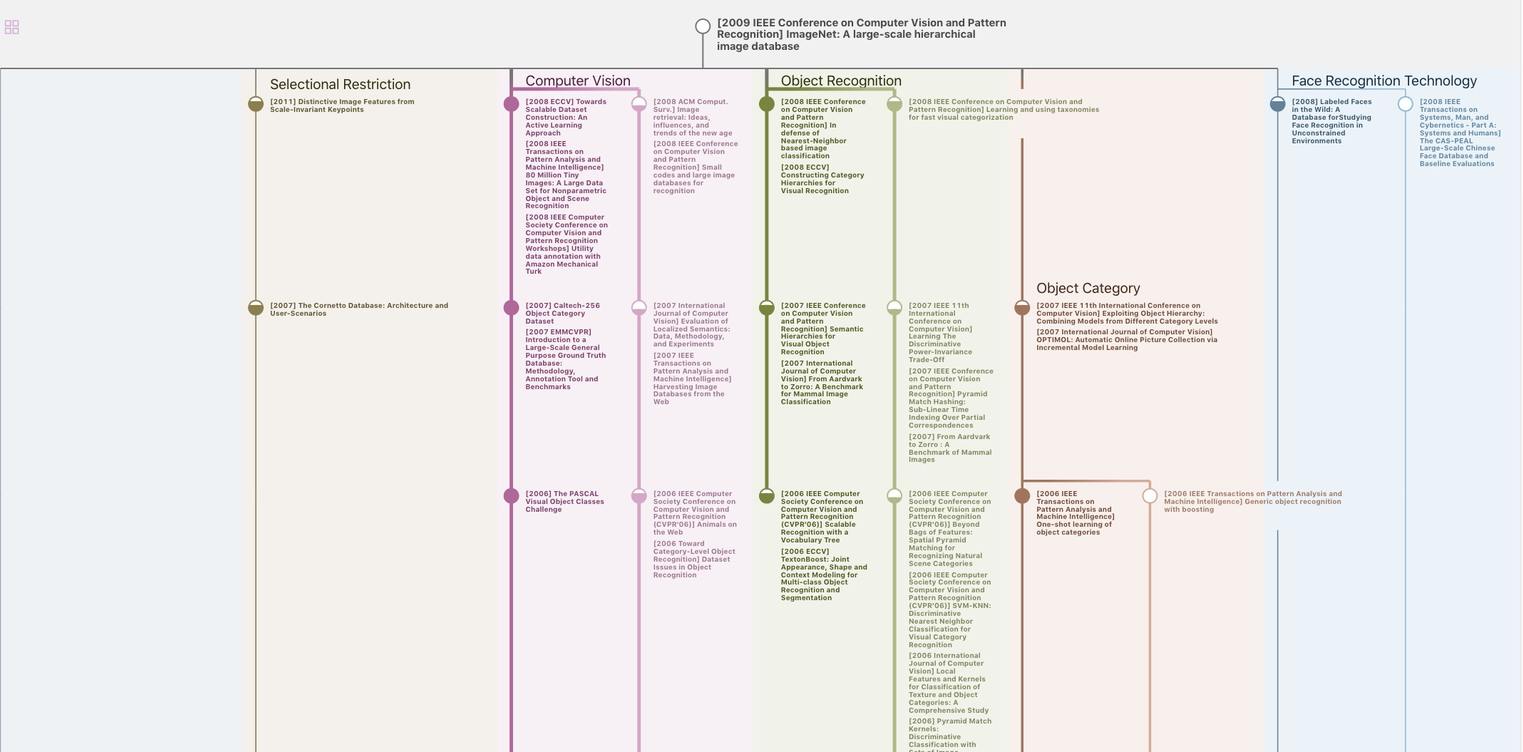
生成溯源树,研究论文发展脉络
Chat Paper
正在生成论文摘要