λ-FL : Serverless Aggregation For Federated Learning
semanticscholar(2022)
摘要
Advances in federated learning (FL) algorithms, along with technologies like differential privacy and homomorphic encryption, have led to FL being increasingly adopted and used in many application domains. Traditional tree-based parallelization schemes can enable FL aggregation to scale to thousands of participants, but (i) waste a lot of resources due to the fact that training on participants, as opposed to aggregation, is the bottleneck in many FL jobs, (ii) require a lot of effort for fault tolerance and elastic scalability. In this paper, we present a new architecture for FL aggregation, based on serverless technology/cloud functions. We describe how our design enables FL aggregation to be dynamically deployed only when necessary, elastically scaled to handle participant joins/leaves and is fault tolerant with minimal effort required on aggregator side. We also demonstrate that our prototype based on Ray (Moritz et al. 2018) scales to thousands of participants, and is able to achieve a > 90% reduction in resource utilization with minimal impact on ag-
更多查看译文
AI 理解论文
溯源树
样例
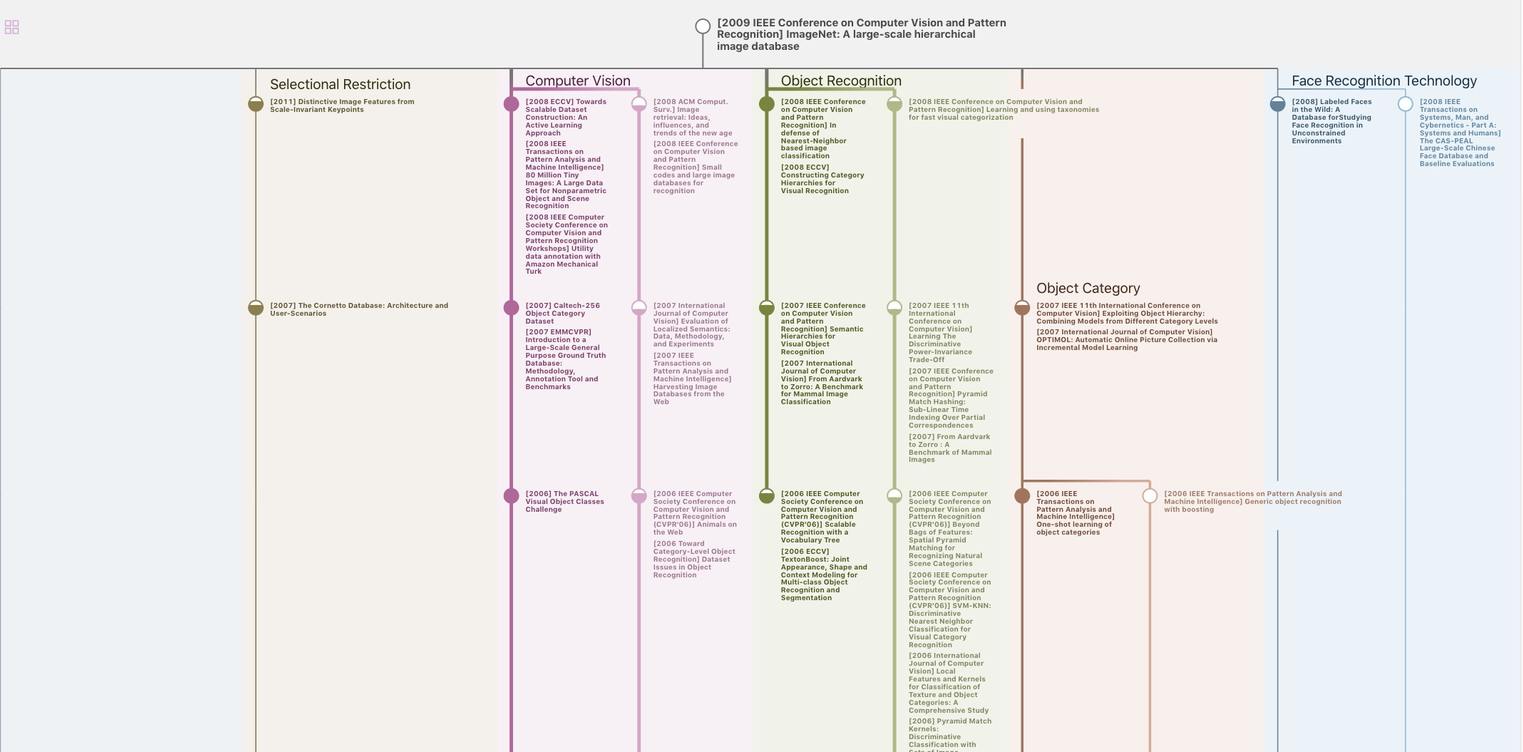
生成溯源树,研究论文发展脉络
Chat Paper
正在生成论文摘要