Machine learning Markovian quantum master equations of few-body observables in interacting spin chains
arXiv (Cornell University)(2022)
摘要
Full information about a many-body quantum system is usually out-of-reach due to the exponential growth - with the size of the system - of the number of parameters needed to encode its state. Nonetheless, in order to understand the complex phenomenology that can be observed in these systems, it is often sufficient to consider dynamical or stationary properties of local observables or, at most, of few-body correlation functions. These quantities are typically studied by singling out a specific subsystem of interest and regarding the remainder of the many-body system as an effective bath. In the simplest scenario, the subsystem dynamics, which is in fact an open quantum dynamics, can be approximated through Markovian quantum master equations. Here, we show how the generator of such a dynamics can be efficiently learned by means of a fully interpretable neural network which provides the relevant dynamical parameters for the subsystem of interest. Importantly, the neural network is constructed such that the learned generator implements a physically consistent open quantum time-evolution. We exploit our method to learn the generator of the dynamics of a subsystem of a many-body system subject to a unitary quantum dynamics. We explore the capability of the network to predict the time-evolution of a two-body subsystem and exploit the physical consistency of the generator to make predictions on the stationary state of the subsystem dynamics.
更多查看译文
关键词
markovian quantum master equations,spin chains,observables,few-body
AI 理解论文
溯源树
样例
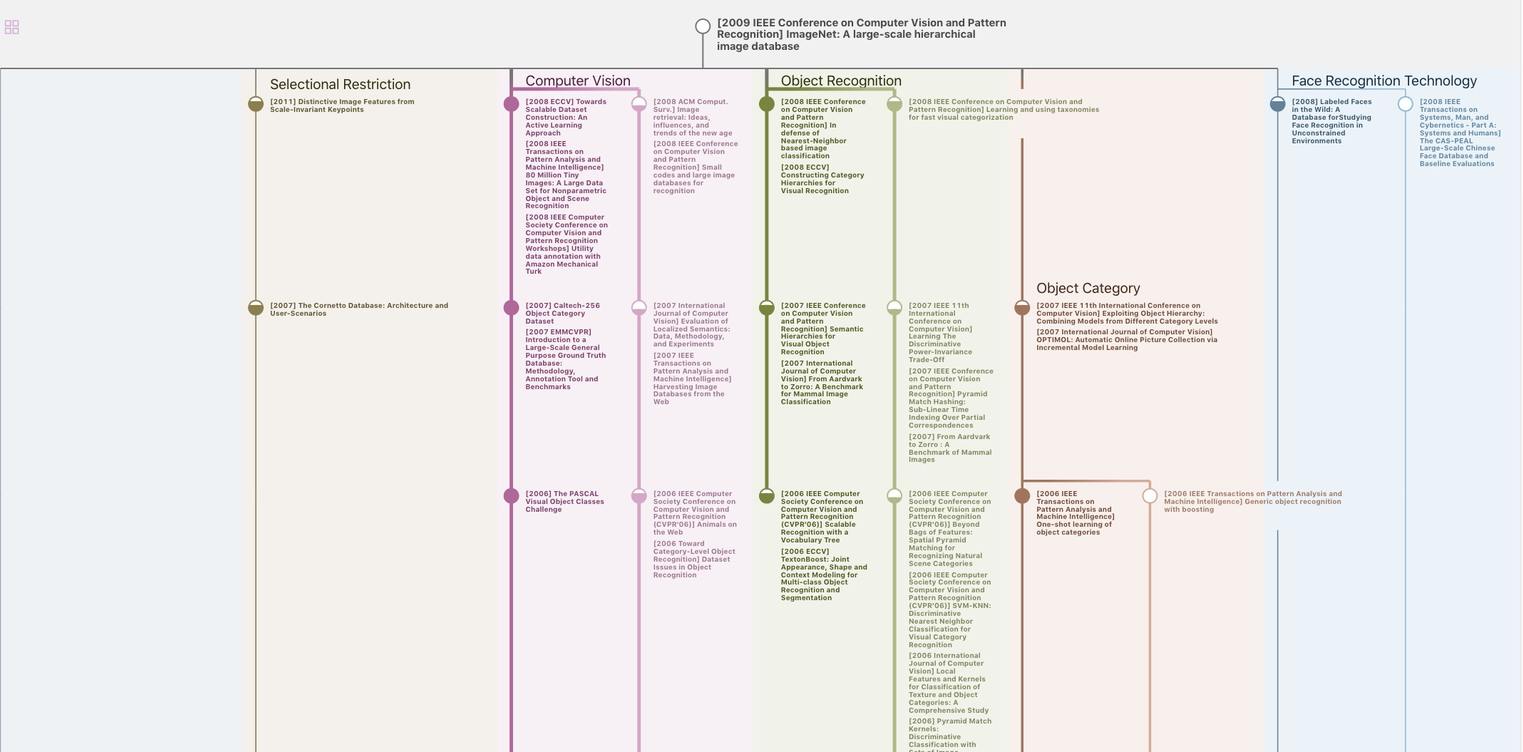
生成溯源树,研究论文发展脉络
Chat Paper
正在生成论文摘要