Fast Reconstruction of non-circular CBCT orbits using CNNs
semanticscholar(2021)
摘要
Non-circular acquisition orbits for cone-beam CT (CBCT) have been investigated for a number of reasons including increased field-of-view, minimal interference within an intraoperative environment, and improved CBCT image quality. Fast reconstruction of the projection data is essential in an interventional imaging setting. While model-based iterative reconstruction can reconstruct data from arbitrary geometries and provide superior noise suppression for a wide variety of non-circular acquisitions, such processing is particularly computationally intensive. In this work, we present a scheme for fast reconstruction of arbitrary non-circular orbits based on Convolutional Neural Networks (CNNs). Specifically, we propose a processing chain that includes a shift-invariant deconvolution of backprojected measurements, followed by CNN processing in a U-Net architecture to address artifacts and deficiencies in the deconvolution process. Synthetic training data is produced using orbital specifications and projections of a large number of procedurally generated objects. Specifically, attenuation volumes are created via randomly placed Delaunay tetrahedrons. We investigated the reconstruction performance for different sets of acquisition orbits including: circular, sinusoidal and randomized parametric trajectories. Our reconstruction scheme yields similar image quality when compared to simultaneous algebraic reconstruction technique (SART) reconstructions, at a small fraction of the computation time. Thus, the proposed work offers a potential way to utilize sophisticated non-circular orbits while maintaining the strict time requirements found in interventional imaging.
更多查看译文
AI 理解论文
溯源树
样例
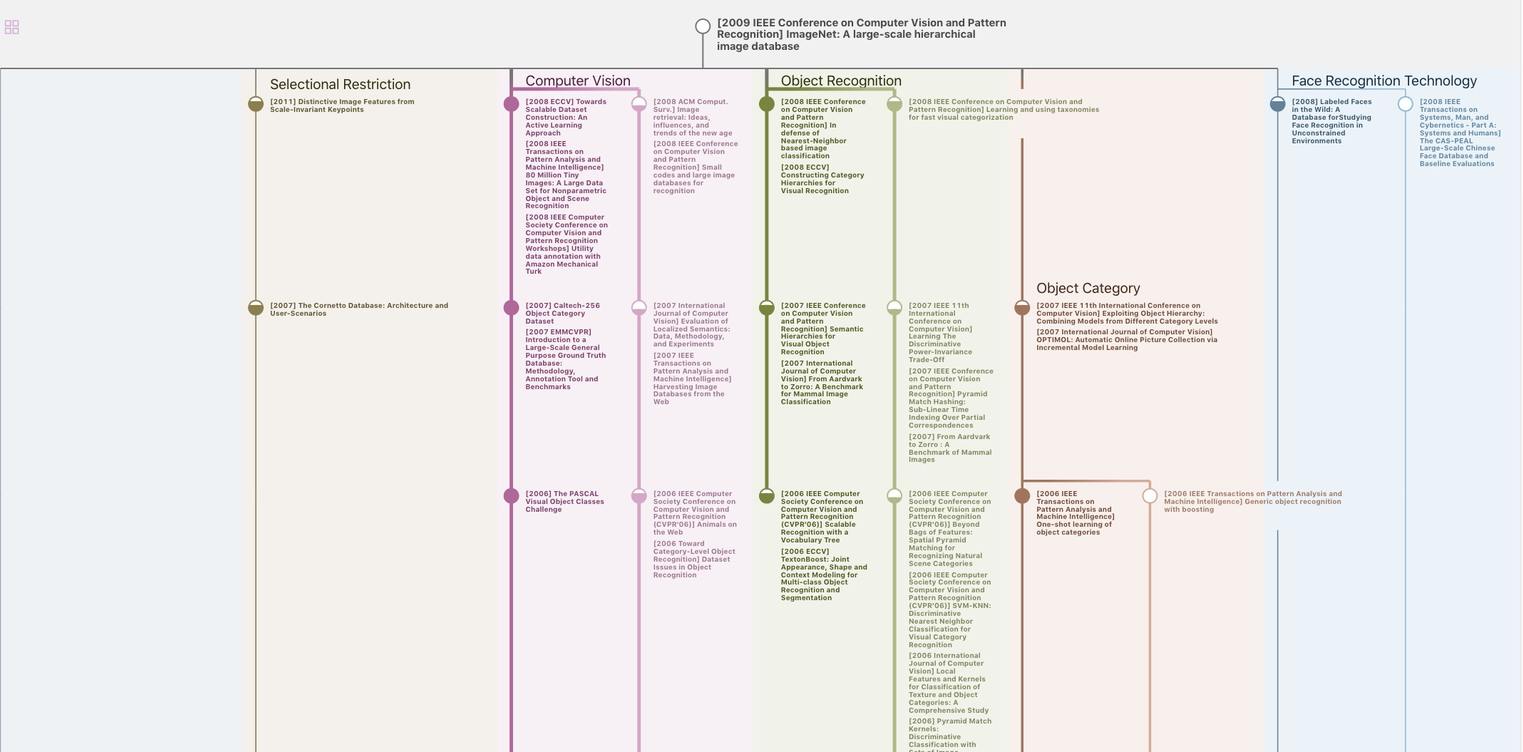
生成溯源树,研究论文发展脉络
Chat Paper
正在生成论文摘要