RFIS: regression-based fuzzy inference system
Neural Computing and Applications(2022)
摘要
This paper proposes a new multivariable fuzzy inference system without explicitly defined fuzzy rules. This system uses Gaussian fuzzy sets for the inputs and linearly and nonlinearly parameterized system functions. To determine their parameters, linear and nonlinear regressions are used. The linear regression is realized by the ridge regression and the nonlinear regression by the Levenberg–Marquardt algorithm. The input fuzzy sets are determined by a multi-objective genetic algorithm with a feature selection method. In the case of linearly parameterized system functions, the following methods are considered: F-test, ReliefF, a regression tree, neighborhood component analysis, and lasso regression. In the case of nonlinearly parameterized system functions, terms from the so-called term matrix are coded in an individual, and they are selected by using a genetic algorithm. In the paper, two pairs of objective functions are defined: one pair, consisting of the number of active predictors and the root of the mean squared error, for constructing fuzzy estimators, and the second pair, consisting of the number of active predictors and confusion values, for constructing fuzzy classifiers. These multi-criteria objective functions enable the selection of models from the Pareto fronts taking into account the compromise between model accuracy and its simplification. The proposed method was tested on four examples: approximation of a one-variable function, two-class classification of banknotes, prediction of a time series, and prediction of automobile fuel consumption. The conducted experiments confirmed the usefulness of the proposed solution.
更多查看译文
关键词
Fuzzy systems, Regression models, Multi-objective optimization, Feature selection
AI 理解论文
溯源树
样例
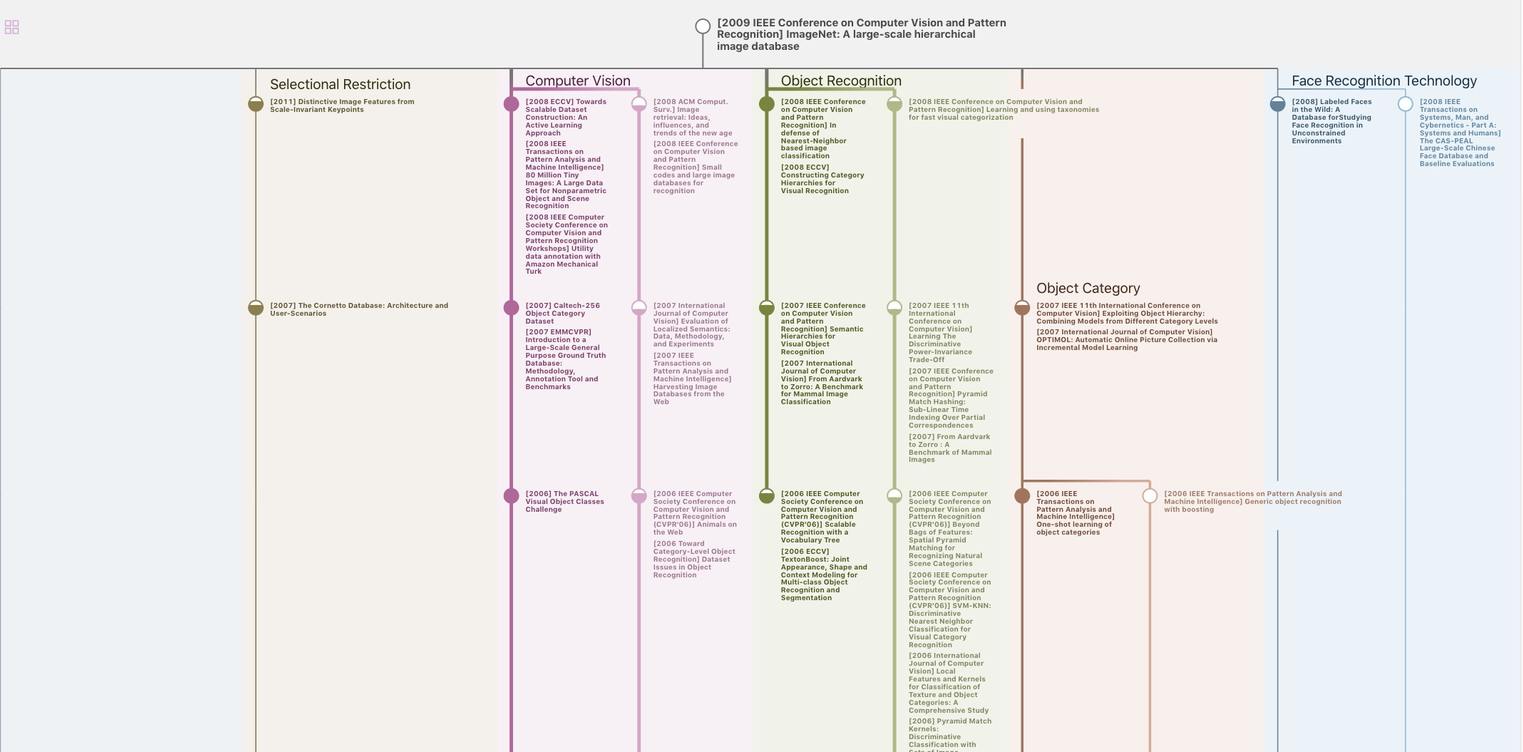
生成溯源树,研究论文发展脉络
Chat Paper
正在生成论文摘要