Automatic atrial fibrillation detection from short ECG signals: A hybrid deep learning approach
IISE Transactions on Healthcare Systems Engineering(2022)
摘要
Atrial fibrillation (AF) is one of the most common arrhythmic complications. Recently, researchers have attempted to use deep learning models, such as convolution neural networks (CNN) and/or Long Short-Term Memory (LSTM) neural networks to alleviate the tedious and time-consuming feature extraction process and achieve good classification results. In this paper we propose a hybrid CNN-LSTM model and use the short ECG signal from the PhysioNet/CinC Challenges 2017 dataset to explore and evaluate the relative performance of four data mining algorithms and three deep learning architectures. The original ECG signal, clinical diagnostic features and 169 features based on time domain, frequency domain and non-linear heart rate variability indicators were used for comparative experiments. The results show that with proper design and tuning, the Hybrid CNN-LSTM model performed much better than other benchmarked algorithms. It achieves 97.42% accuracy, 95.65% sensitivity, 97.14% specificity, 0.99 AUC (Area under the ROC curve) value and 0.98 F1 score. In general, with proper design and configuration, deep learning can be effective for automatic AF detection while data mining methods require domain knowledge and an extensive feature extraction and selection process to get satisfactory results. However, most machine learning algorithms, including deep learning models, perform the task as a black box, making it almost impossible to determine what features in the signal are critical to the analysis.
更多查看译文
关键词
automatic atrial fibrillation detection,atrial fibrillation,short ecg signals,deep learning,deep learning approach
AI 理解论文
溯源树
样例
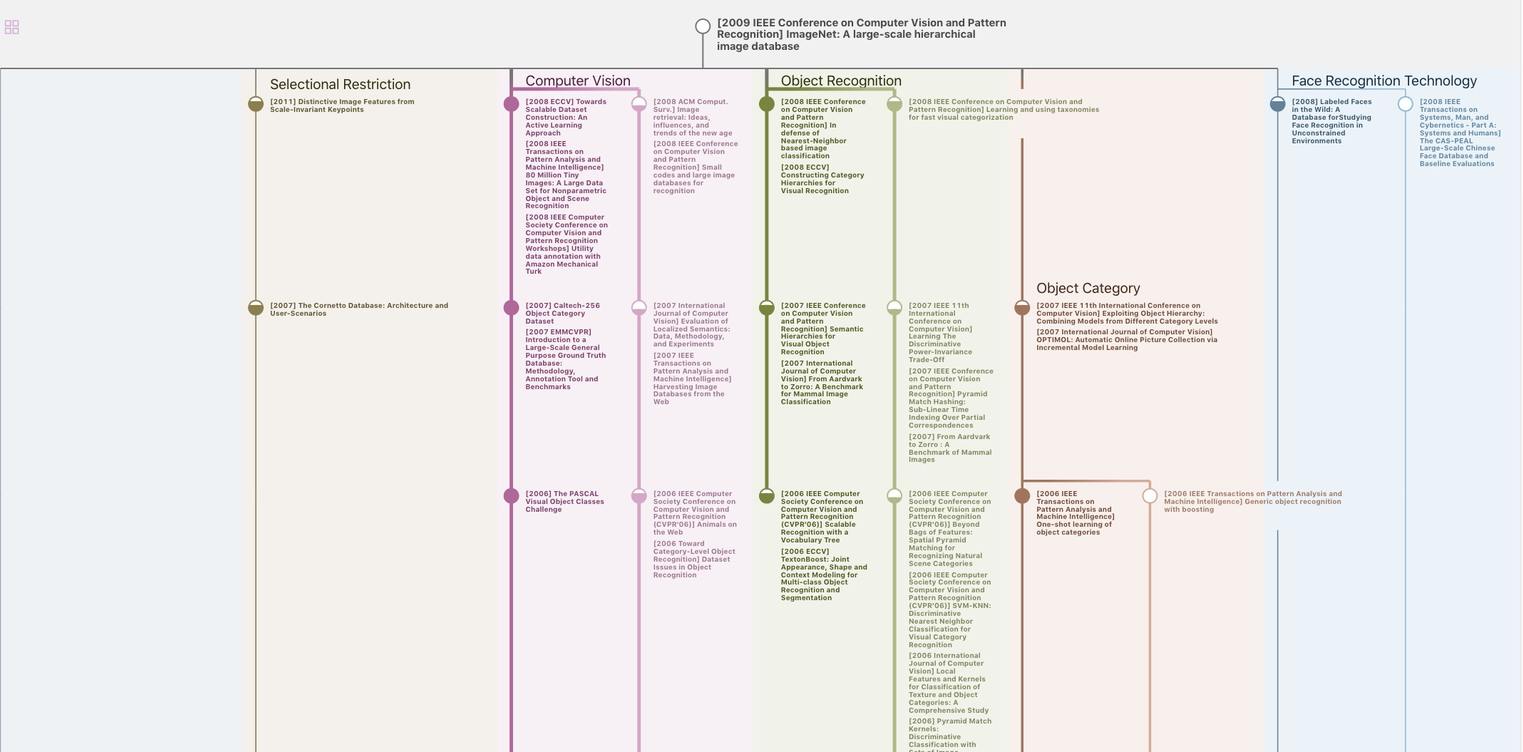
生成溯源树,研究论文发展脉络
Chat Paper
正在生成论文摘要