Compressive Differentially-Private Federated Learning Through Universal Vector Quantization
semanticscholar(2021)
摘要
Collaborative and federated machine learning is an essential vehicle for achieving privacy preserving machine learning. By not forcing participants to share their private datasets, we can adhere to strict local as well as international privacy protection legal regimes. However, a federated learning mechanism is usually hindered by the overhead communication costs between the central server and participants when communication channels have constrained capacities. Furthermore, just refraining from sharing the data will not lead us to absolute privacy, e.g. in presence of privacy attacks such as membership inference. Differential Privacy has provided a set of rigorous privacy standards to protect individual records of a dataset being used by a randomized mechanism. As differential privacy has been widely accepted as the de facto privacy standard in machine learning, it could potentially mitigate privacy concerns. However, addition of differential privacy usually costs even more communication overhead, putting more pressure on uplink and downlink channels. In this work, we present a novel algorithm for achieving both differential privacy and reduced communication overhead through compression of client-server communication by means of quantization. Not only we show acceptable levels of differential privacy, we also show significant gains in terms of communication efficiency by compressing the data on the constrained uplink channel.
更多查看译文
AI 理解论文
溯源树
样例
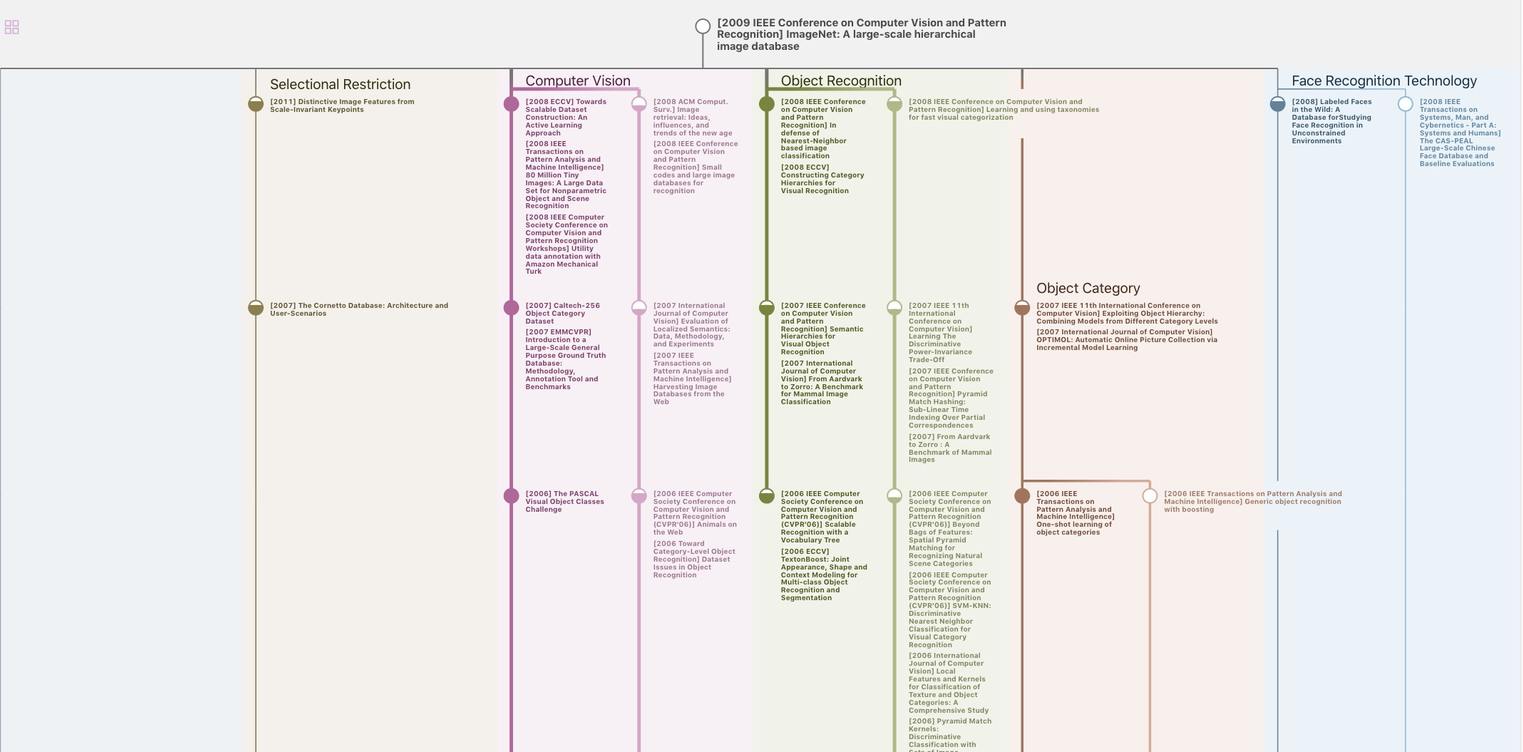
生成溯源树,研究论文发展脉络
Chat Paper
正在生成论文摘要